Pseudo-Random Number Generation using Generative Adversarial Networks.
Nemesis/UrbReas/SoGood/IWAISe/GDM@PKDD/ECML(2018)
摘要
Pseudo-random number generators (PRNG) are a fundamental element of many security algorithms. We introduce a novel approach to their implementation, by proposing the use of generative adversarial networks (GAN) to train a neural network to behave as a PRNG. Furthermore, we showcase a number of interesting modifications to the standard GAN architecture. The most significant is partially concealing the output of the GAN’s generator, and training the adversary to discover a mapping from the overt part to the concealed part. The generator therefore learns to produce values the adversary cannot predict, rather than to approximate an explicit reference distribution. We demonstrate that a GAN can effectively train even a small feed-forward fully connected neural network to produce pseudo-random number sequences with good statistical properties. At best, subjected to the NIST test suite, the trained generator passed around 99% of test instances and 98% of overall tests, outperforming a number of standard non-cryptographic PRNGs.
更多查看译文
关键词
Adversarial neural networks, Pseudo-random number generators, Neural cryptography
AI 理解论文
溯源树
样例
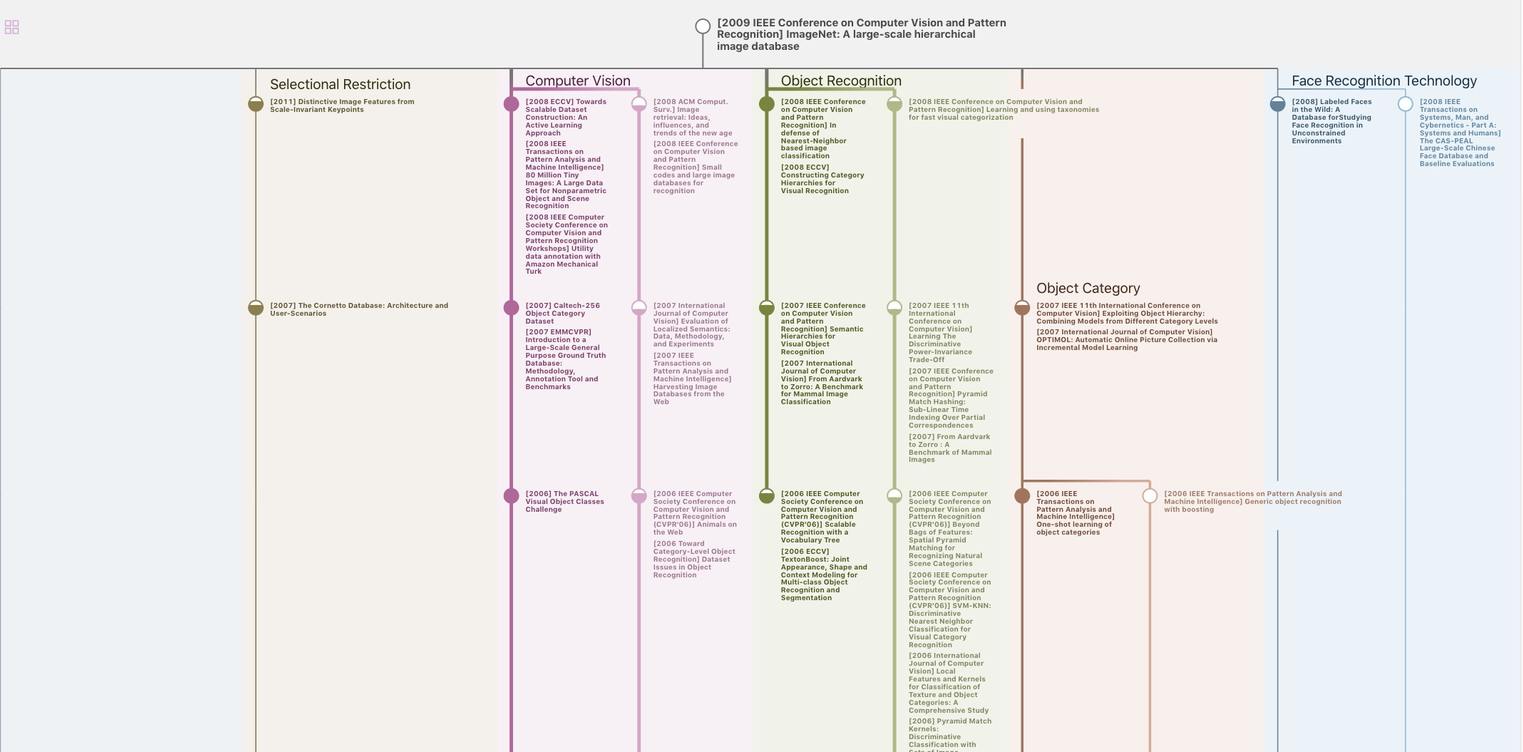
生成溯源树,研究论文发展脉络
Chat Paper
正在生成论文摘要