Rao-Blackwellized Stochastic Gradients for Discrete Distributions
International Conference on Machine Learning(2019)
摘要
We wish to compute the gradient of an expectation over a finite or countably infinite sample space having $K \leq \infty$ categories. When $K$ is indeed infinite, or finite but very large, the relevant summation is intractable. Accordingly, various stochastic gradient estimators have been proposed. In this paper, we describe a technique that can be applied to reduce the variance of any such estimator, without changing its bias---in particular, unbiasedness is retained. We show that our technique is an instance of Rao-Blackwellization, and we demonstrate the improvement it yields on a semi-supervised classification problem and a pixel attention task.
更多查看译文
关键词
discrete distributions,stochastic,rao-blackwellized
AI 理解论文
溯源树
样例
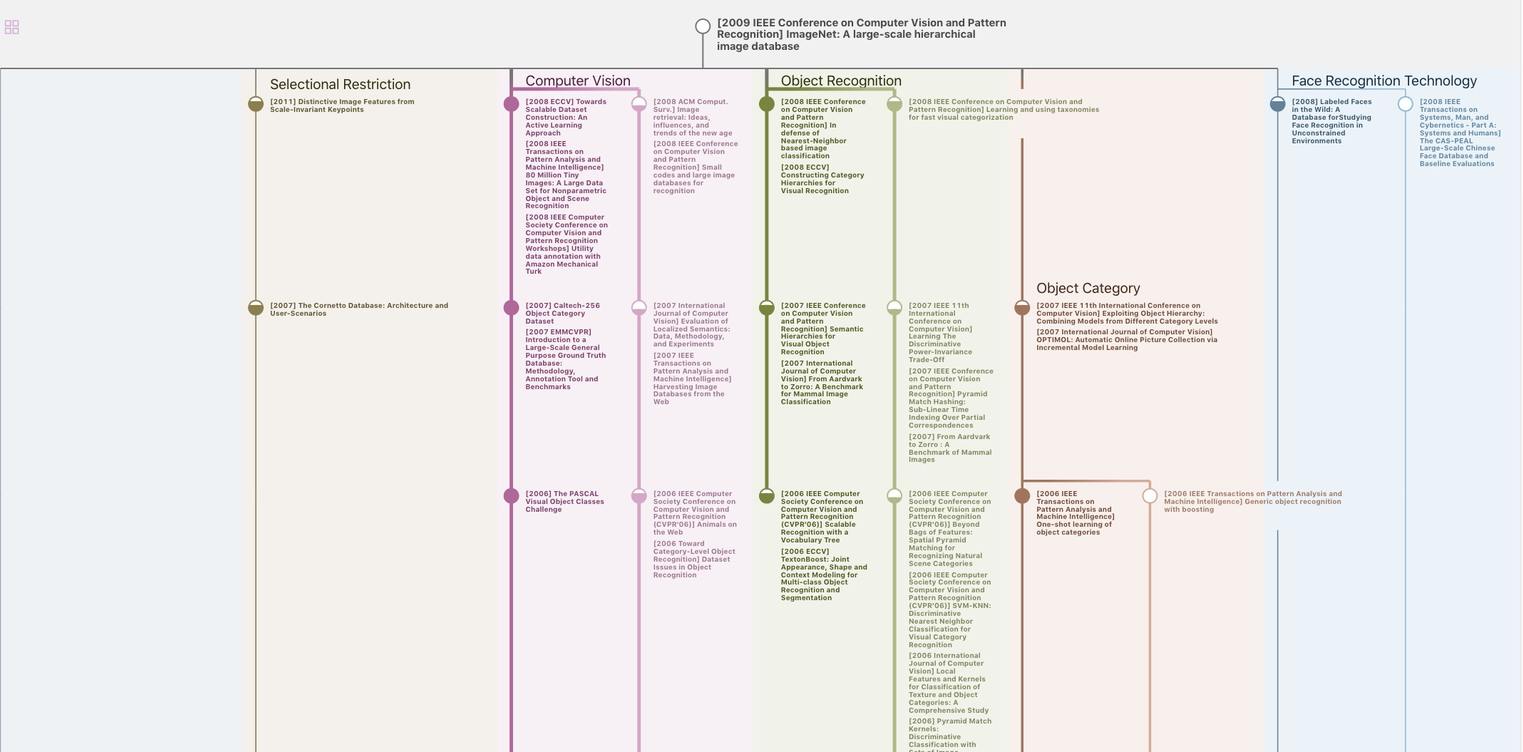
生成溯源树,研究论文发展脉络
Chat Paper
正在生成论文摘要