Approximate Bayesian Computation via Population Monte Carlo and Classification
arXiv: Machine Learning(2018)
摘要
Approximate Bayesian computation (ABC) methods can be used to sample from posterior distributions when the likelihood function is unavailable or intractable, as is often the case in biological systems. ABC methods suffer from inefficient particle proposals in high dimensions, and subjectivity in the choice of summary statistics, discrepancy measure, and error tolerance. Sequential Monte Carlo (SMC) methods have been combined with ABC to improve the efficiency of particle proposals, but suffer from subjectivity and require many simulations from the likelihood function. Likelihood-Free Inference by Ratio Estimation (LFIRE) leverages classification to estimate the posterior density directly but does not explore the parameter space efficiently. This work proposes a classification approach that approximates population Monte Carlo (PMC), where model class probabilities from classification are used to update particle weights. This approach, called Classification-PMC, blends adaptive proposals and classification, efficiently producing samples from the posterior without subjectivity. We show through a simulation study that Classification-PMC outperforms two state-of-the-art methods: ratio estimation and SMC ABC when it is computationally difficult to simulate from the likelihood.
更多查看译文
AI 理解论文
溯源树
样例
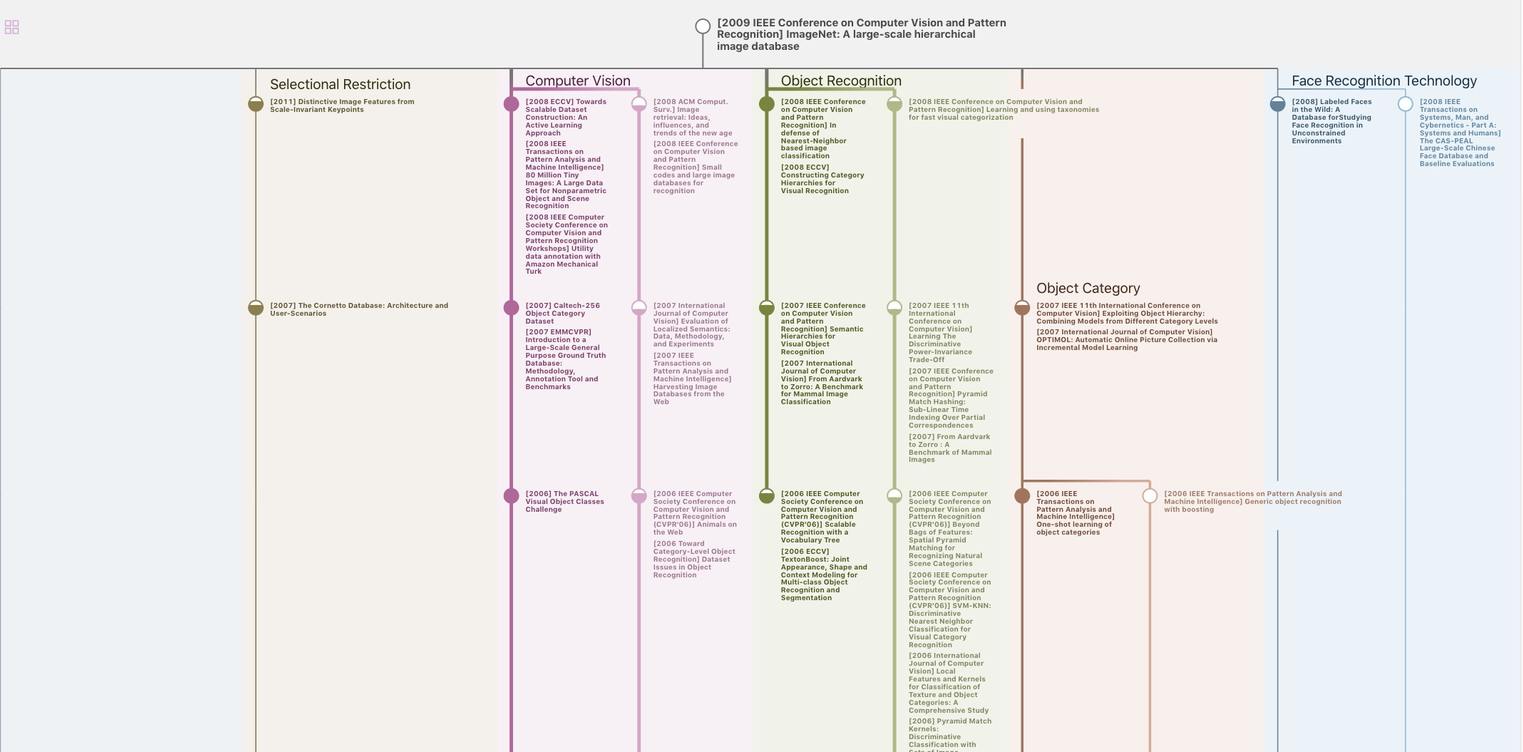
生成溯源树,研究论文发展脉络
Chat Paper
正在生成论文摘要