Synchronization of Nonlinearly and Stochastically Coupled Markovian Switching Networks via Event-Triggered Sampling.
IEEE Transactions on Neural Networks and Learning Systems(2018)
摘要
This paper studies the exponential synchronization problem for a new array of nonlinearly and stochastically coupled networks via events-triggered sampling (ETS) by self-adaptive learning. The networks include the following features: 1) a Bernoulli stochastic variable is introduced to describe the random structural coupling; 2) a stochastic variable with positive mean is used to model the coupling strength; and 3) a continuous time homogeneous Markov chain is employed to characterize the dynamical switching of the coupling structure and pinned node sets. The proposed network model is capable to capture various stochastic effect of an external environment during the network operations. In order to reduce networks' workload, different ETS strategies for network self-adaptive learning are proposed under continuous and discrete monitoring, respectively. Based on these ETS approaches, several sufficient conditions for synchronization are derived by employing stochastic Lyapunov-Krasovskii functions, the properties of stochastic processes, and some linear matrix inequalities. Numerical simulations are provided to demonstrate the effectiveness of the theoretical results and the superiority of the proposed ETS approach.
更多查看译文
关键词
Couplings,Synchronization,Switches,Stochastic processes,Complex networks,Monitoring,Uncertainty
AI 理解论文
溯源树
样例
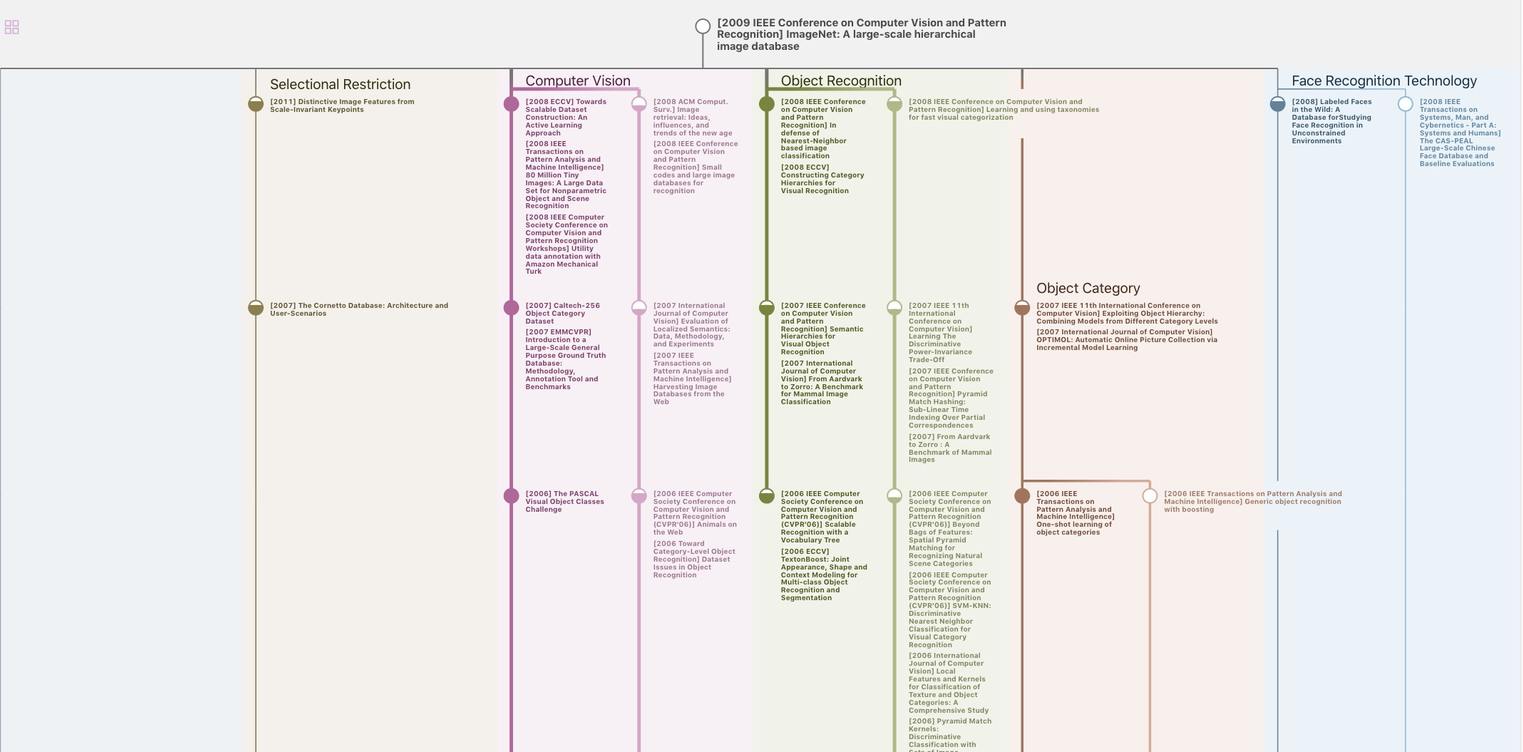
生成溯源树,研究论文发展脉络
Chat Paper
正在生成论文摘要