Uncertain data classification with additive kernel support vector machine.
Data & Knowledge Engineering(2018)
摘要
In this work, a classification learning algorithm is designed within the framework of support vector machines through modeling uncertain data with additive kernels, which are introduced to calculate the similarity between uncertain samples characterized by probability density functions (PDFs). The PDFs are used as features of the uncertain samples, where the value of a feature is not a single value, but a set of values that represent the probability distribution of the noise. This is different with the existing methods which represent an uncertain sample by a set of new samples around it, but use the farthest or nearest value in the distribution to construct the optimal hyperplane. With the properties of kernel functions, we can easily extend additive kernels to compute the similarity between samples described with multiple uncertain features. Furthermore, we introduce an efficient algorithm to compute the kernel functions, and solve the additive kernel SVMs. The experimental results show the efficiency of additive-kernel SVMs in uncertain data classification.
更多查看译文
关键词
Uncertain data,Additive kernel,Support vector machines,Classification
AI 理解论文
溯源树
样例
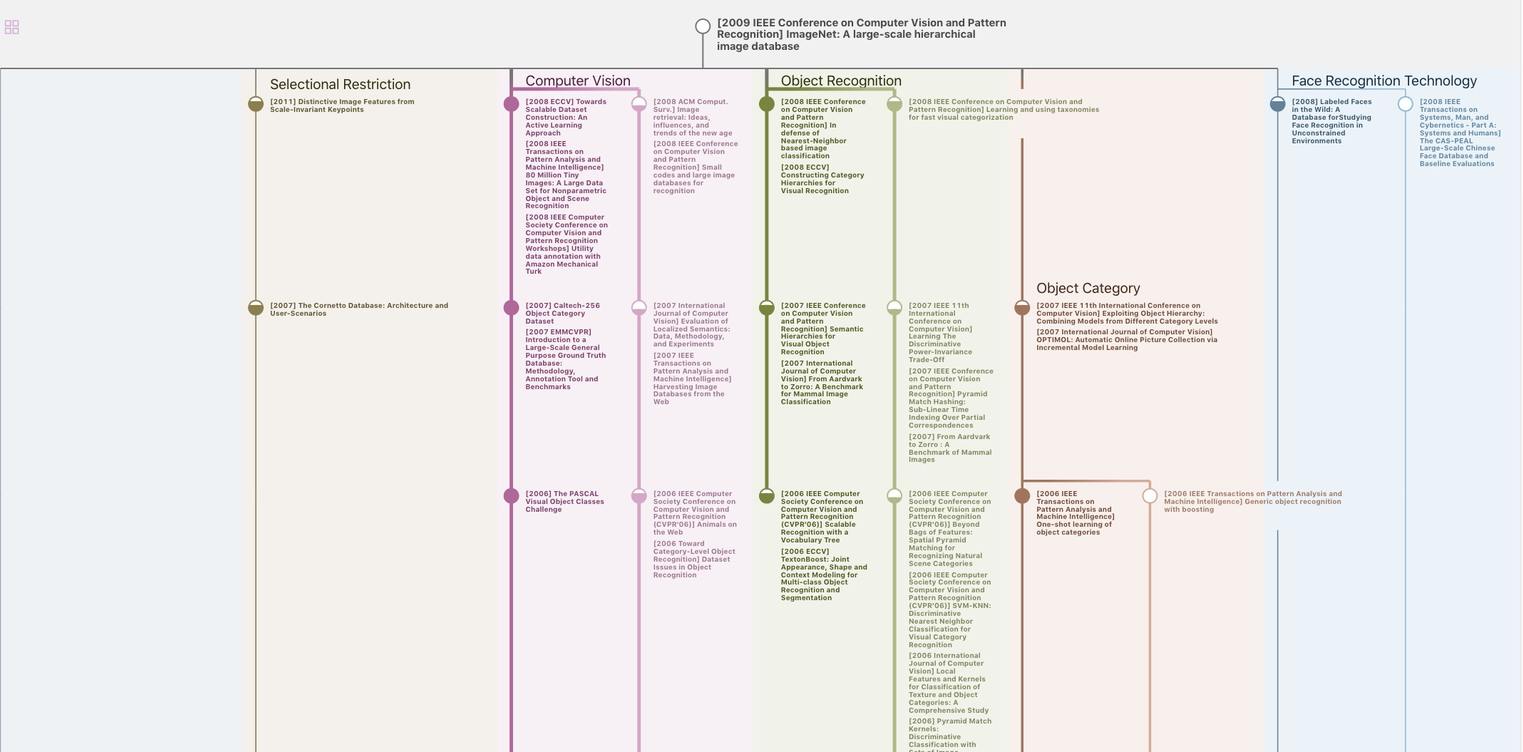
生成溯源树,研究论文发展脉络
Chat Paper
正在生成论文摘要