RevHashNet: Perceptually de-hashing real-valued image hashes for similarity retrieval.
Signal Processing: Image Communication(2018)
摘要
Image hashing has attracted increasing popularity in recent years. Some off-the-shelf image hashing methods are able to generate more compact and robust hashes for fast indexing and content-based similarity retrieval. However, the ability to infer original image contents from their real-valued image hashes has seldom been examined. Inherited from cryptographic hashing for image privacy protection, general image hashing is supposed to be a non-revertible function. Should there be a way to revert (or perceptually reconstruct) images from the corresponding real-valued image hashes? This paper explores the feasibility of perceptually image hashing reversion, and fill this gap by proposing a deep learning based framework, entitled RevHashNet. Given real-valued image hashes from certain image hashing methods, the proposed RevHashNet can automatically reconstruct perceptually similar images with respect to the original ones with high visual quality. Experiments and simulations on real image datasets support the de-hashing effectiveness of the proposed RevHashNet.
更多查看译文
关键词
RevHashNet,Image hashing reversion,Image de-hashing,Security,Deep learning
AI 理解论文
溯源树
样例
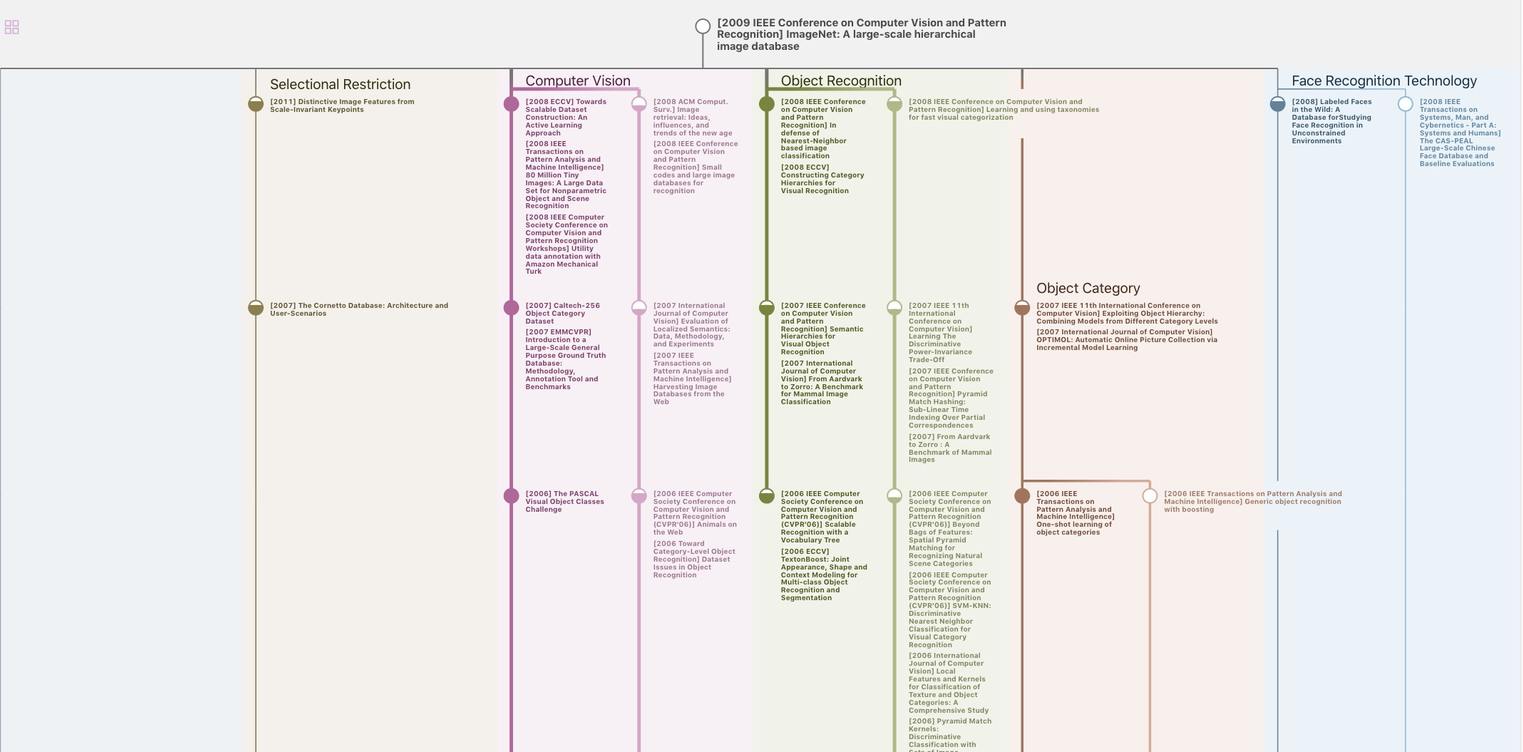
生成溯源树,研究论文发展脉络
Chat Paper
正在生成论文摘要