An Efficient Recommender System Method Based on the Numerical Relevances and the Non-Numerical Structures of the Ratings.
IEEE ACCESS(2018)
摘要
In this paper, we propose a collaborative filtering method designed to improve the current memory-based prediction times without worsening and even improving the existing accuracy results. The accuracy improvement is achieved by combining the numerical relevance of the ratings with non-numerical information based on the votes structure. The improvement of the prediction time is achieved by setting four actions: 1) simplification of the similarity measure design, in order to minimize the necessary calculations; 2) construction and maintenance of a model that simplifies the predictions processing; 3) optimization of the computation, using a set-based model and a bit-based processing implementation; and 4) switching between the bit processing and the numerical processing, depending on the density of the users' ratings. Experimental results show the improvements both in the prediction time and the accuracy. Experiments have used a significant amount of state-of-the-art baselines and collaborative filtering public data sets.
更多查看译文
关键词
Recommender systems,collaborative filtering,performance,prediction time,similarity measures,model-based methods
AI 理解论文
溯源树
样例
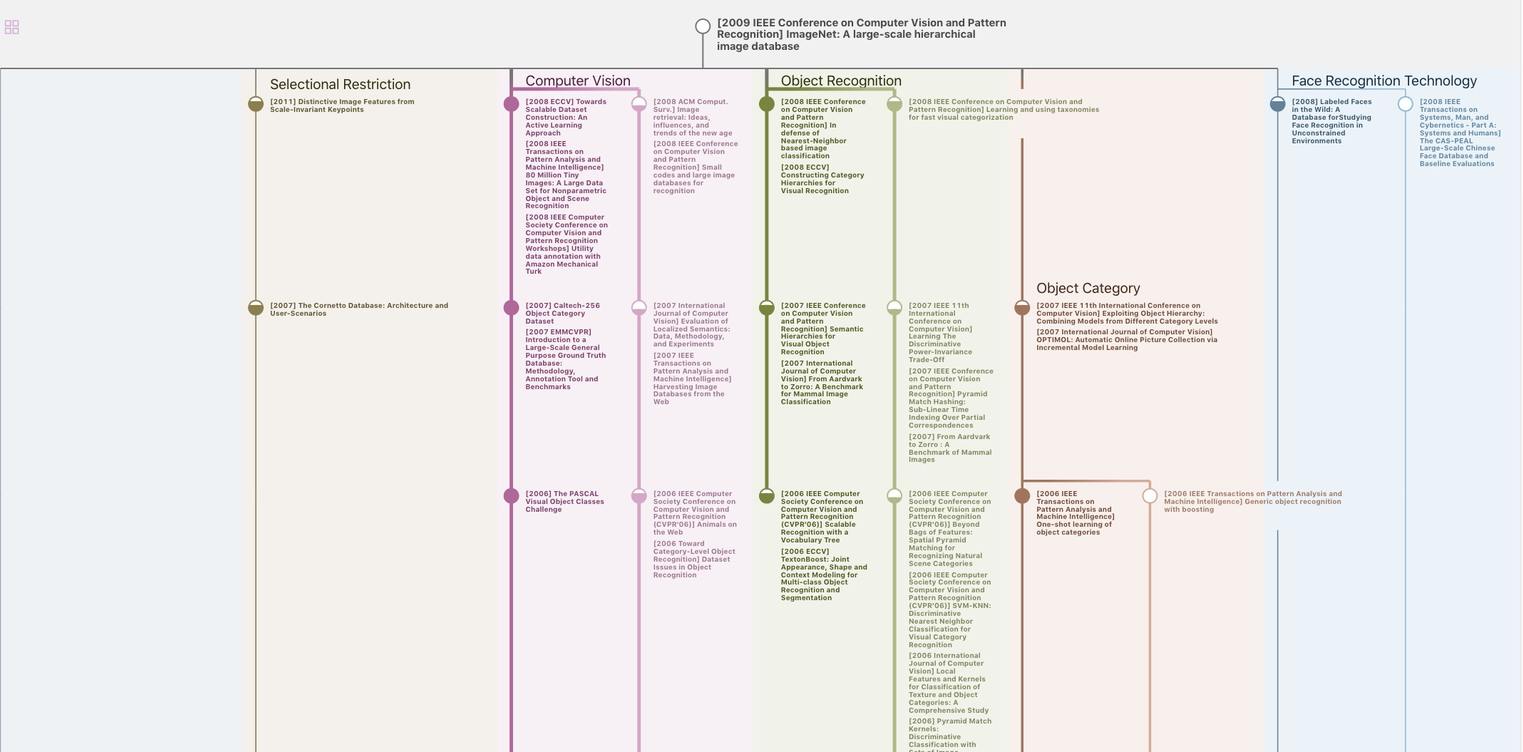
生成溯源树,研究论文发展脉络
Chat Paper
正在生成论文摘要