Skeleton Feature Fusion Based on Multi-Stream LSTM for Action Recognition.
IEEE ACCESS(2018)
摘要
Human action recognition from skeleton sequences has attracted a lot of attention in the computer vision community. Long short term memory (LSTM) network has shown its promising performance for this problem, due to their strengths in modeling the dependencies and temporal dynamics of sequential data. However, original LSTM is difficult to grasp the dynamics of entire sequence data, if the input feature of each time step is just a simple combination of raw skeleton data. In this paper, we present a fusion model to make full use of the skeleton data through multi-stream LSTM for action recognition. In each stream of the model, skeleton feature fed to each step of LSTM are extracted from different time duration, which are called single frame feature, short term feature, and long term feature, respectively. Single frame feature represents static pose, which is converted from joints coordinates directly. Short term feature represents skeleton kinematics, which is extracted from a short time window. Long term feature represents joints mutuality during the action process, which is extracted from a longer time window. All these features are modeled by LSTM, and the final states of LSTM streams are fused to predict the underlying actions. The proposed model makes better use of the skeleton dynamics than standard LSTM model. Experimental results on two benchmark skeleton data sets NTU RGB+D data set and SBU interaction dataset show that our proposed approach achieved significant performance.
更多查看译文
关键词
Action recognition,long short term memory network,skeleton feature fusion
AI 理解论文
溯源树
样例
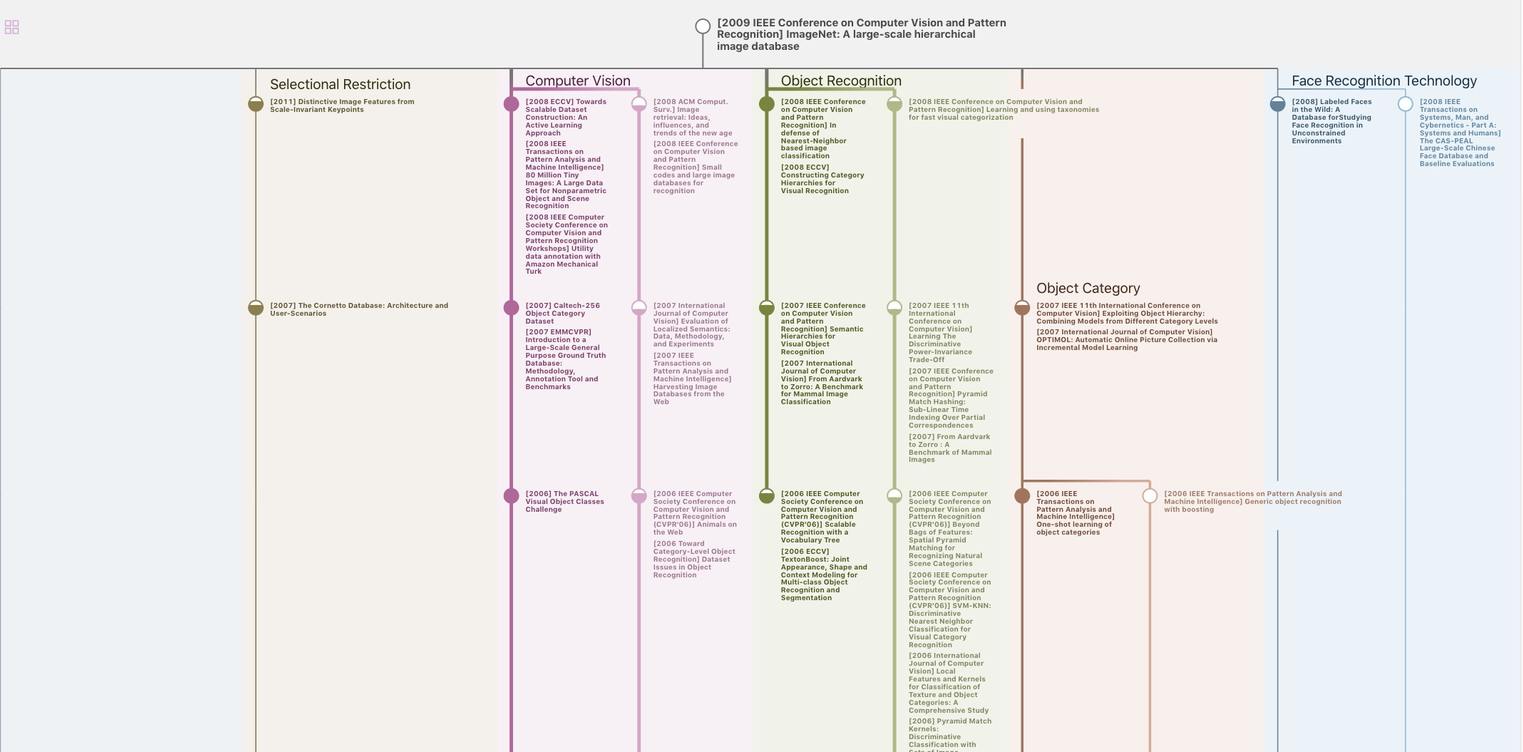
生成溯源树,研究论文发展脉络
Chat Paper
正在生成论文摘要