Automatic Multicarrier Waveform Classification via PCA and Convolutional Neural Networks.
IEEE ACCESS(2018)
摘要
To make up the disadvantages of OFDM-QAM applied in 4G systems, some new non-orthogonal asynchronous PHY multicarrier techniques have been proposed for multiple 5G application scenarios, such as universal-filtered multicarrier (UFMC) and filterbank-based multicarrier (FBMC). Accurate classification of multiple multicarrier waveforms is indispensable in the future wireless communication systems. Here, we propose a new multicarrier waveforms classification system, which utilizes deep convolutional neural networks to classify OFDM-QAM, UFMC, and FBMC-OQAM. Since the classification accuracy is greatly reduced in AWGN environment, principal component analysis (PCA)-based processing method is proposed in this paper to suppress the AWGN and reduce the dimensions of inputs of convolutional neural networks. Amplitude data is used in this paper as input for CNN and shows promise when compared to which uses raw I/Q data as input. The dense channel environment effects including timing errors, carrier frequency offsets, and multi-fading channels are also considered in this paper and our proposed system can obtain the high classification accuracy in noisy channels.
更多查看译文
关键词
OFDM-QAM,FBMC-OQAM,UFMC,multicarrier waveform classification,PCA,CNN
AI 理解论文
溯源树
样例
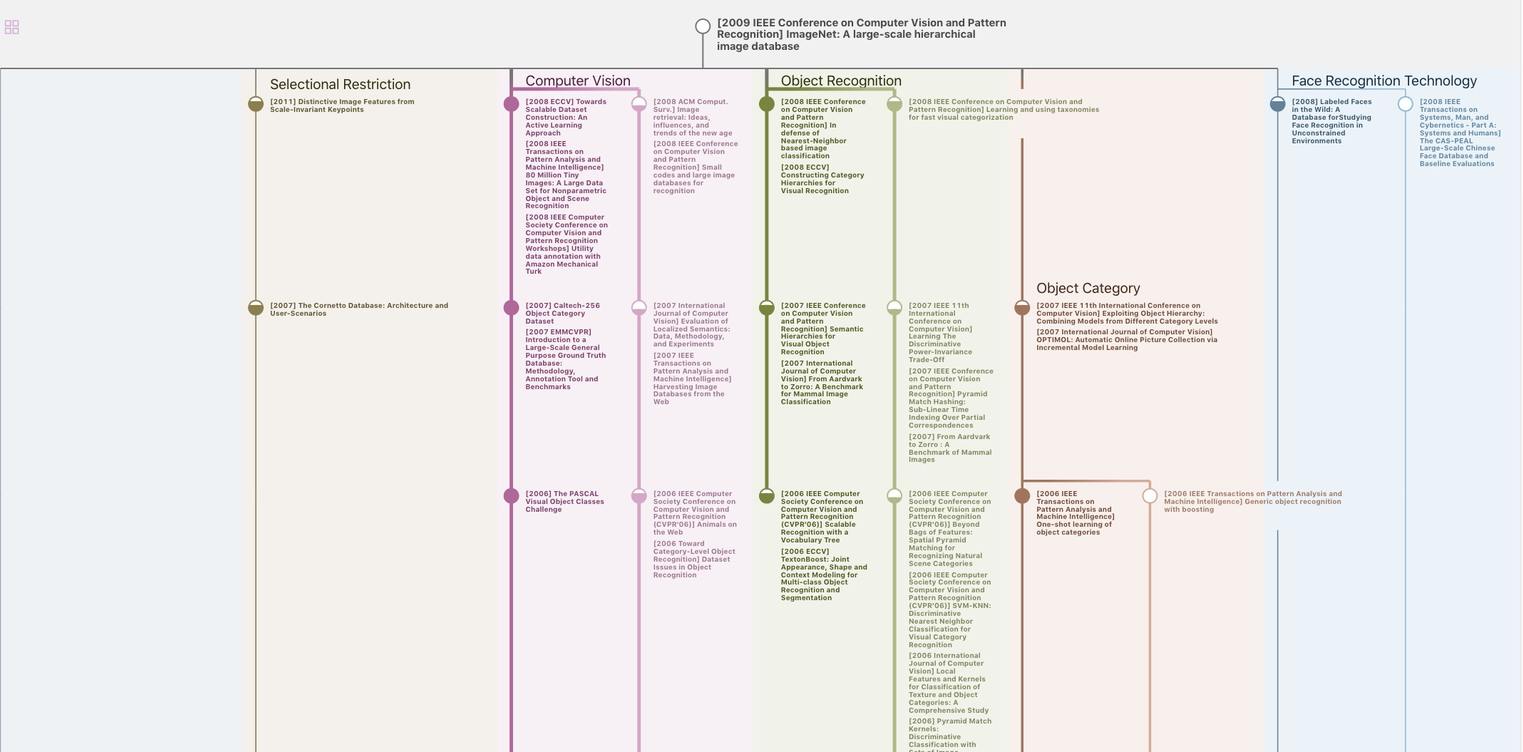
生成溯源树,研究论文发展脉络
Chat Paper
正在生成论文摘要