Learning acoustic features to detect Parkinson's disease
NEUROCOMPUTING(2018)
摘要
Detecting Parkinson's disease (PD) based on voice analysis is meaningful due to its non-invasion and convenience. Traditional features adopted for PD detection are often hand-crafted, in which special expertise is needed. In this paper, we propose to employ feature learning technique to learn features automatically, where special expertise is unnecessary. First, calculate the first derivative of Mel-spectrogram with respect to time for pre-processed audio signals. Then, we use spherical K-means to train two dictionaries using samples of PD patients and healthy controls, respectively. Third, frames in an audio signal are encoded with the two dictionaries, followed by a pooling method to summarize over frames to obtain the learned features. Experiments show the superiority of the proposed method after comparison with two baseline algorithms. Additionally, issues like clustering number in spherical K-means and pooling method are discussed. Finally, by analyzing the similarities between the hand-crafted and learned features, some knowledge is obtained, which can guide future learning and design of features in PD detection. (C) 2018 Elsevier B.V. All rights reserved.
更多查看译文
关键词
Feature learning,Mel-spectrogram,Parkinson's disease,Spherical K-means
AI 理解论文
溯源树
样例
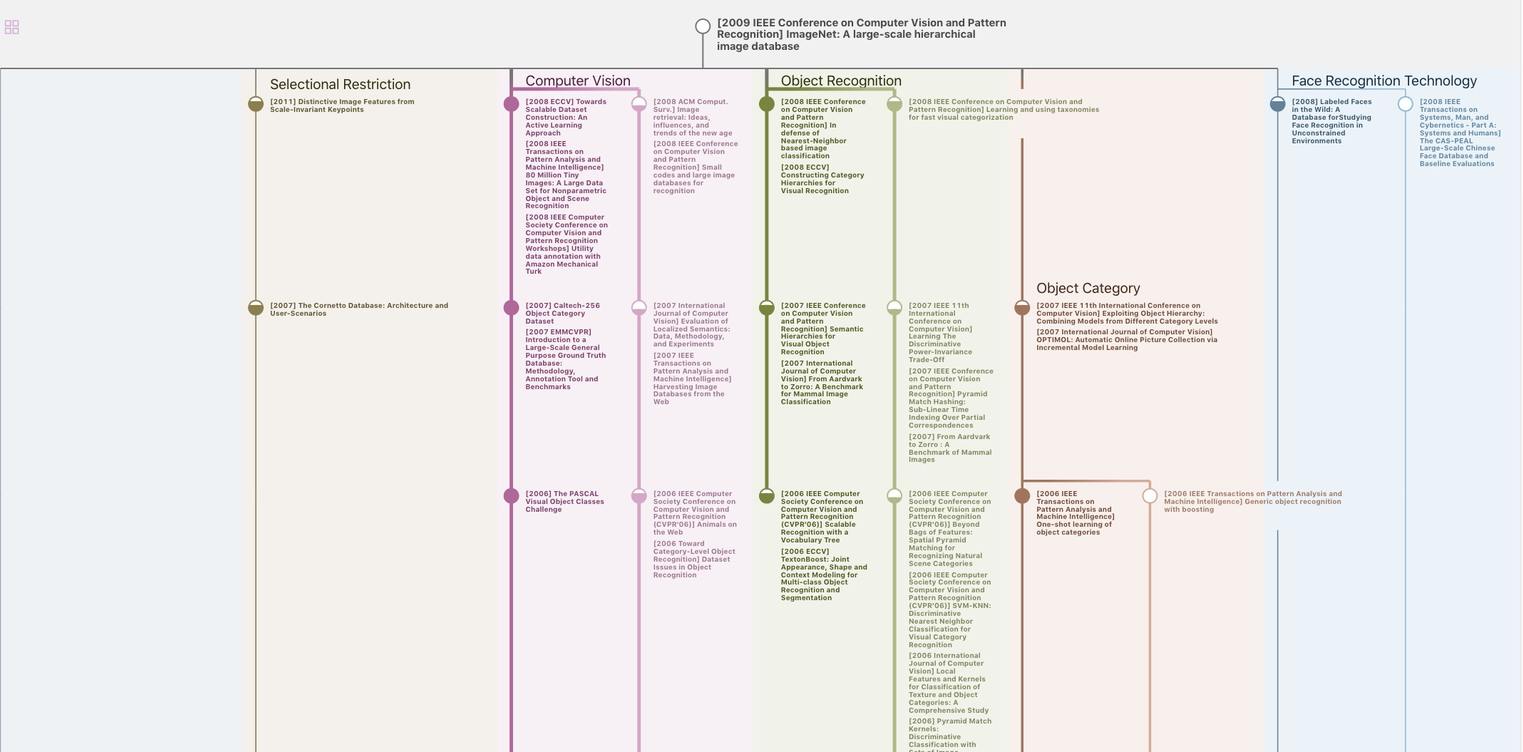
生成溯源树,研究论文发展脉络
Chat Paper
正在生成论文摘要