Linear feature extraction for ranking
Inf. Retr. Journal(2018)
摘要
We address the feature extraction problem for document ranking in information retrieval. We then propose LifeRank, a Li near f eature e xtraction algorithm for Rank ing. In LifeRank, we regard each document collection for ranking as a matrix, referred to as the original matrix . We try to optimize a transformation matrix , so that a new matrix (dataset) can be generated as the product of the original matrix and a transformation matrix. The transformation matrix projects high-dimensional document vectors into lower dimensions. Theoretically, there could be very large transformation matrices, each leading to a new generated matrix. In LifeRank, we produce a transformation matrix so that the generated new matrix can match the learning to rank problem. Extensive experiments on benchmark datasets show the performance gains of LifeRank in comparison with state-of-the-art feature selection algorithms.
更多查看译文
关键词
Feature extraction, Dimension reduction, Learning to rank, Information retrieval
AI 理解论文
溯源树
样例
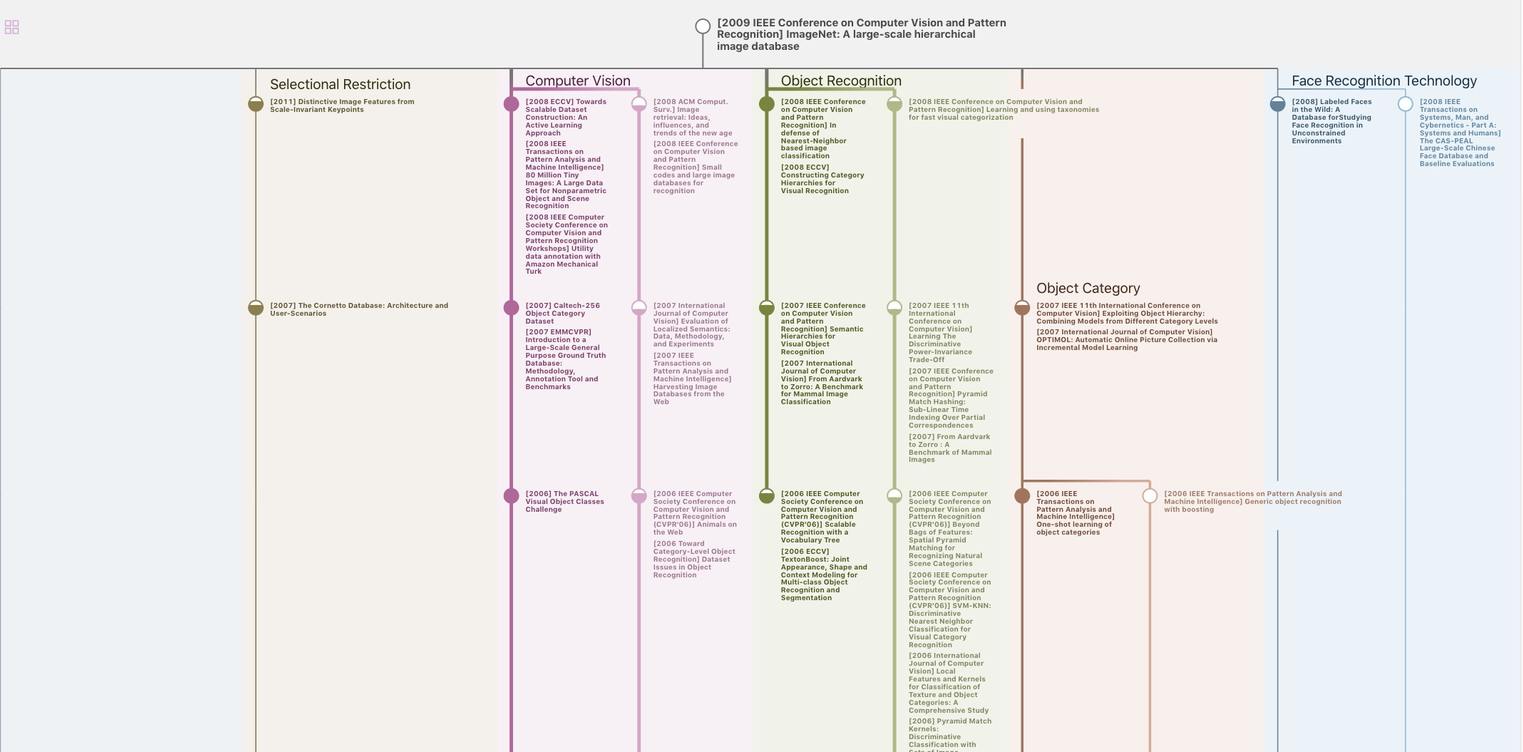
生成溯源树,研究论文发展脉络
Chat Paper
正在生成论文摘要