A visual analytics approach for pattern-recognition in patient-generated data.
JOURNAL OF THE AMERICAN MEDICAL INFORMATICS ASSOCIATION(2018)
摘要
Objective:To develop and test a visual analytics tool to help clinicians identify systematic and clinically meaningful patterns in patient-generated data (PGD) while decreasing perceived information overload.
Methods:Participatory design was used to develop Glucolyzer, an interactive tool featuring hierarchical clustering and a heatmap visualization to help registered dietitians (RDs) identify associative patterns between blood glucose levels and per-meal macronutrient composition for individuals with type 2 diabetes (T2DM). Ten RDs participated in a within-subjects experiment to compare Glucolyzer to a static logbook format. For each representation, participants had 25 minutes to examine 1 month of diabetes self-monitoring data captured by an individual with T2DM and identify clinically meaningful patterns. We compared the quality and accuracy of the observations generated using each representation.
Results:Participants generated 50% more observations when using Glucolyzer (98) than when using the logbook format (64) without any loss in accuracy (69% accuracy vs 62%, respectively, p = .17). Participants identified more observations that included ingredients other than carbohydrates using Glucolyzer (36% vs 16%, p = .027). Fewer RDs reported feelings of information overload using Glucolyzer compared to the logbook format. Study participants displayed variable acceptance of hierarchical clustering.
Conclusions:Visual analytics have the potential to mitigate provider concerns about the volume of self-monitoring data. Glucolyzer helped dietitians identify meaningful patterns in self-monitoring data without incurring perceived information overload. Future studies should assess whether similar tools can support clinicians in personalizing behavioral interventions that improve patient outcomes.
更多查看译文
关键词
visual analytics,self-monitoring data,patient-generated data,diabetes clustering
AI 理解论文
溯源树
样例
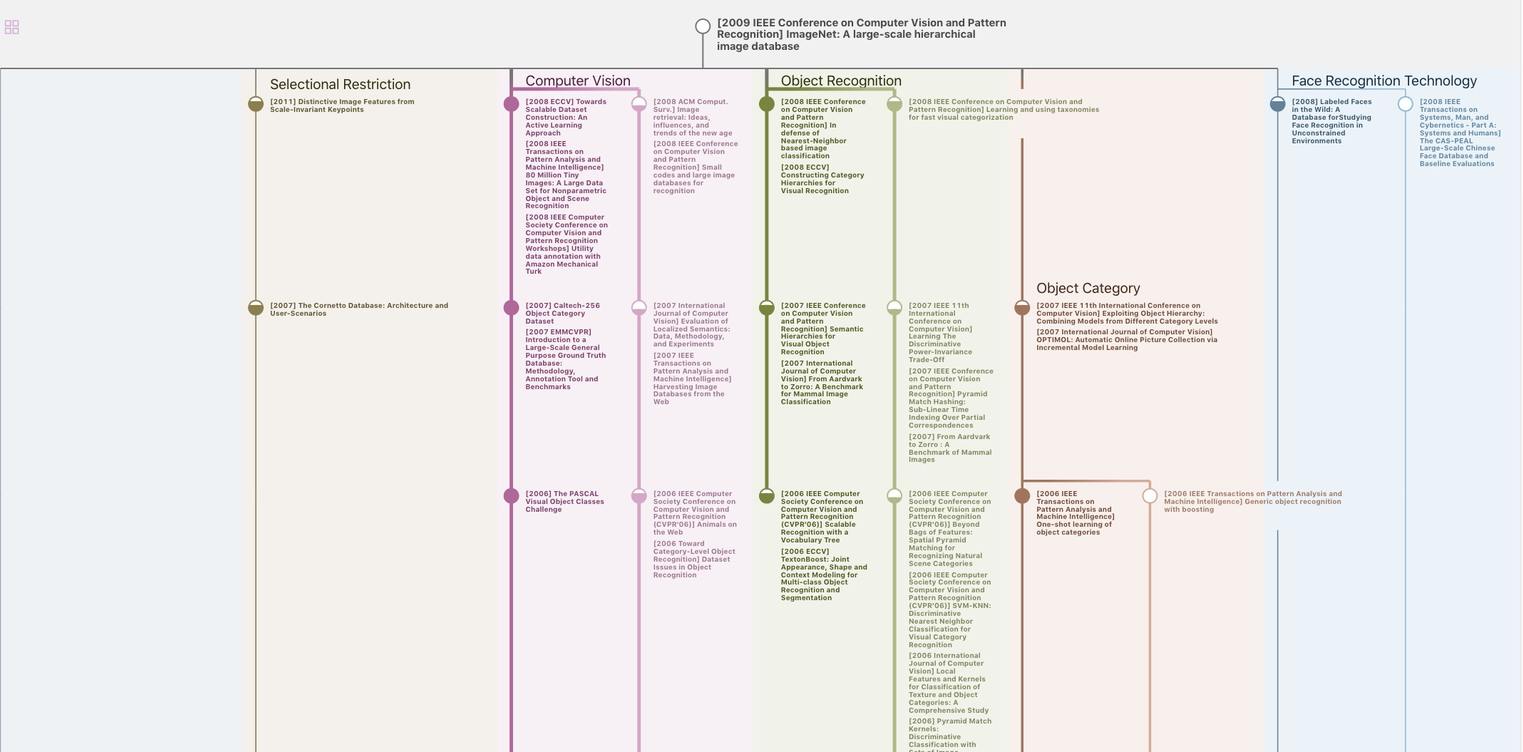
生成溯源树,研究论文发展脉络
Chat Paper
正在生成论文摘要