Medical Image Segmentation Using Seeded Fuzzy C-Means: A Semi-Supervised Clustering Algorithm
2018 INTERNATIONAL JOINT CONFERENCE ON NEURAL NETWORKS (IJCNN)(2018)
摘要
One of the least invasive means of diagnosing certain diseases is through the use of imaging tests. Automated analysis usually begins by detecting the regions to be investigated and extracting relevant information such as shape and texture. In this paper, we propose a semi-supervised clustering algorithm called Seeded Fuzzy C-means to segment regions of interest in medical images. Seeded Fuzzy C-means is based on the well-known Fuzzy C-means; however, it also uses information provided by the physician to insert constraints during group choices. In this way, the element is placed in a group with a higher degree of certainty. We evaluated the proposed algorithm on a total of 2,200 images in leukemia, skin cancer, cervical cancer, and glaucoma image databases. Two semi-supervised clustering algorithms were implemented from literature to perform this analysis. The results illustrate the ability of the algorithm to efficiently assist physicians in detecting regions of interest, as it achieved an "Excellent" Kappa index in most of the tests.
更多查看译文
关键词
Semi-supervised learning, medical image segmentation, fuzzy clustering
AI 理解论文
溯源树
样例
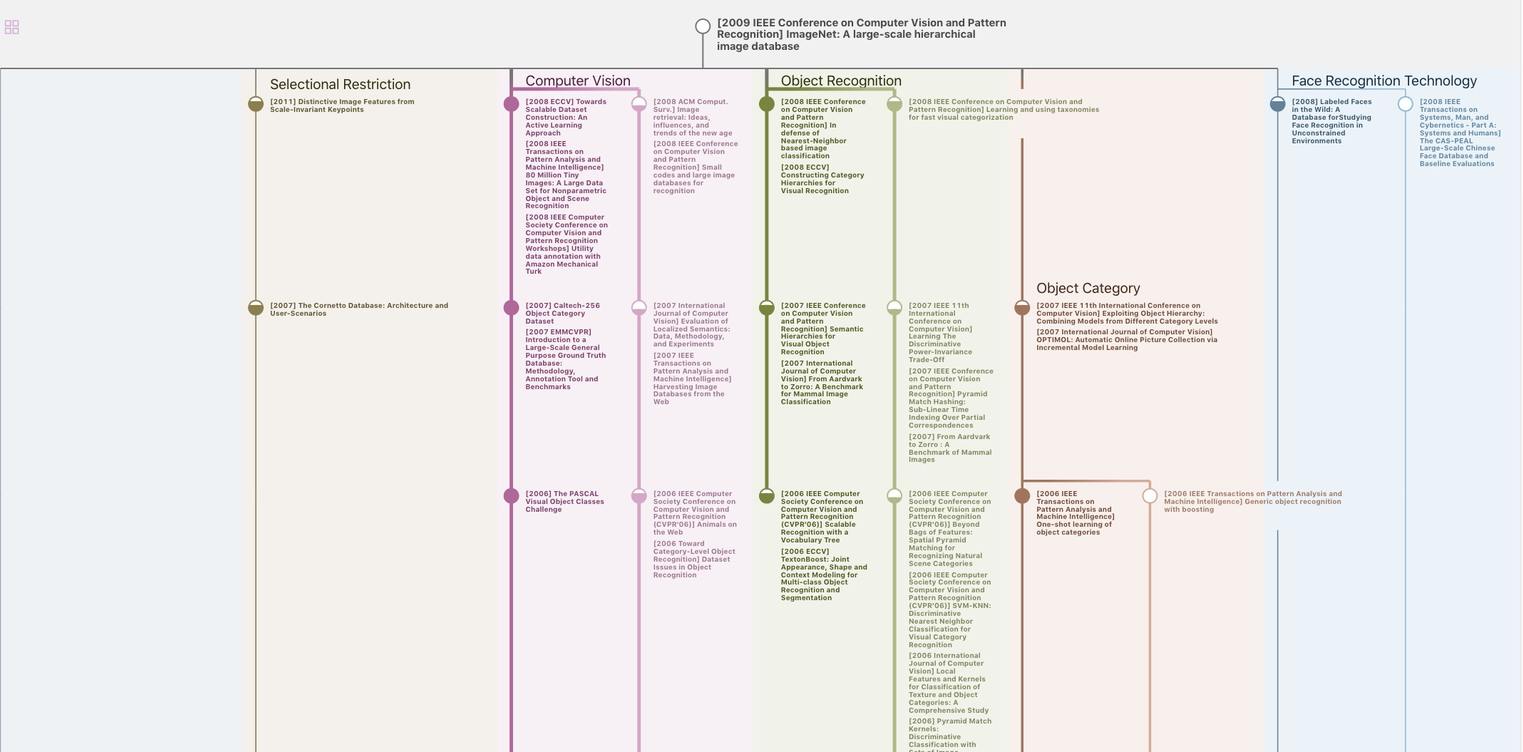
生成溯源树,研究论文发展脉络
Chat Paper
正在生成论文摘要