A Timescale Invariant Stdp-Based Spiking Deep Network For Unsupervised Online Feature Extraction From Event-Based Sensor Data
2018 INTERNATIONAL JOINT CONFERENCE ON NEURAL NETWORKS (IJCNN)(2018)
摘要
We introduce a deep spiking convolutional neural network of integrate-and-fire (IF) neurons, which extracts hierarchical features from a stream of event-based vision data in an unsupervised fashion and online. Our network operates with a simple spike-timing dependent plasticity (STDP) rule, which does not require the definition of an input timescale. We demonstrate how our network is able to learn translational invariant features from the event-based N-MNIST dataset, while preserving dynamic information in the data. We demonstrate how these features can be used to perform online classification. Our network is the first neuromorphic system which can be used to learn complex hierarchical features unsupervised from a continuous stream of address-event-representation (AER) data, operating outside of a database framework and on multiple timescales. Additionally, all the mechanisms we use are simple and generic. This could open the possibility to implement our system on current neuromorphic hardware, to build a real-world fully adaptive event-based vision system.
更多查看译文
关键词
timescale invariant STDP-based spiking deep network,unsupervised online feature extraction,event-based sensor data,deep spiking convolutional neural network,event-based vision data,unsupervised fashion,input timescale,translational invariant features,event-based N-MNIST dataset,online classification,complex hierarchical features,address-event-representation data,multiple timescales,real-world fully adaptive event-based vision system,integrate-and-fire neurons,IF,spike-timing dependent plasticity rule,address-event-representation data,AER,neuromorphic hardware
AI 理解论文
溯源树
样例
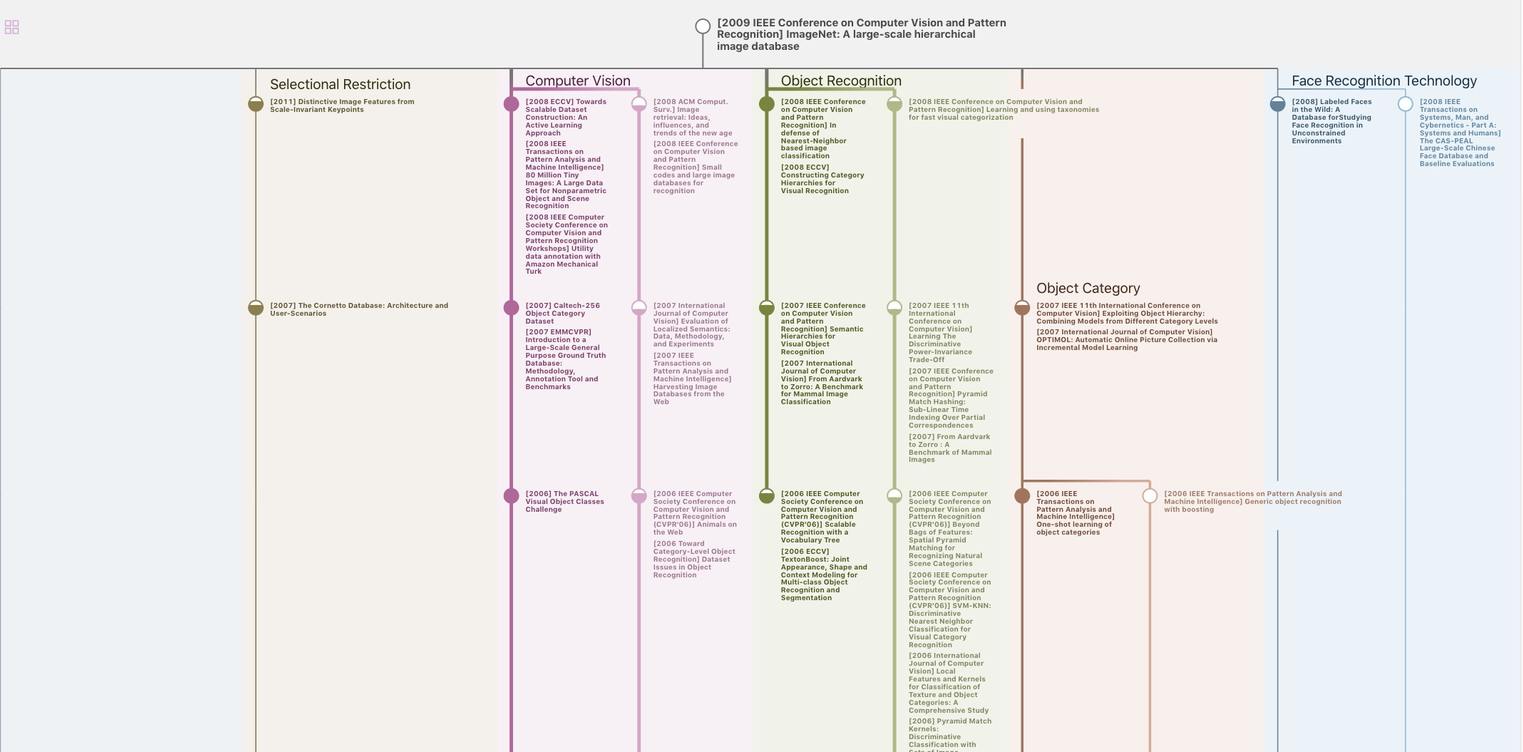
生成溯源树,研究论文发展脉络
Chat Paper
正在生成论文摘要