Topic Recommendation Using Doc2vec
2018 INTERNATIONAL JOINT CONFERENCE ON NEURAL NETWORKS (IJCNN)(2018)
摘要
The ever-increasing number of electronic content stored in digital libraries requires a significant amount of effort in cataloguing and has led to self-deposit solutions where the authors submit and publish their own digital records. Even in self-deposit, going through the abstract and assigning subject terms or keywords is a time consuming and expensive process, yet crucial for the metadata quality of the record that affects retrieval. Therefore, an automatic, or even a semi-automatic process that can recommend topics for a new entry is of huge practical value. A system that can address that has to rely basically on two components, one component for efficiently representing the relevant information of the new document and one component for recommending an appropriate set of topics based on the representation of the previous stage. In this work, different candidate solutions for both components are investigated and compared. For the first stage both distributed Document to Vector (doc2vec) and conventional Bag of Words (BoW) components are employed, while for the latter two different transformation approaches from the field of multi-label classification are compared. For the comparison, a collection of Ph.D. abstracts (similar to 19000 documents) from the MIT Libraries Dspace repository is used suggesting that different combinations can provide high quality solutions.
更多查看译文
关键词
Recommender system, multilabel classification, word2vec, doc2vec, bag of words
AI 理解论文
溯源树
样例
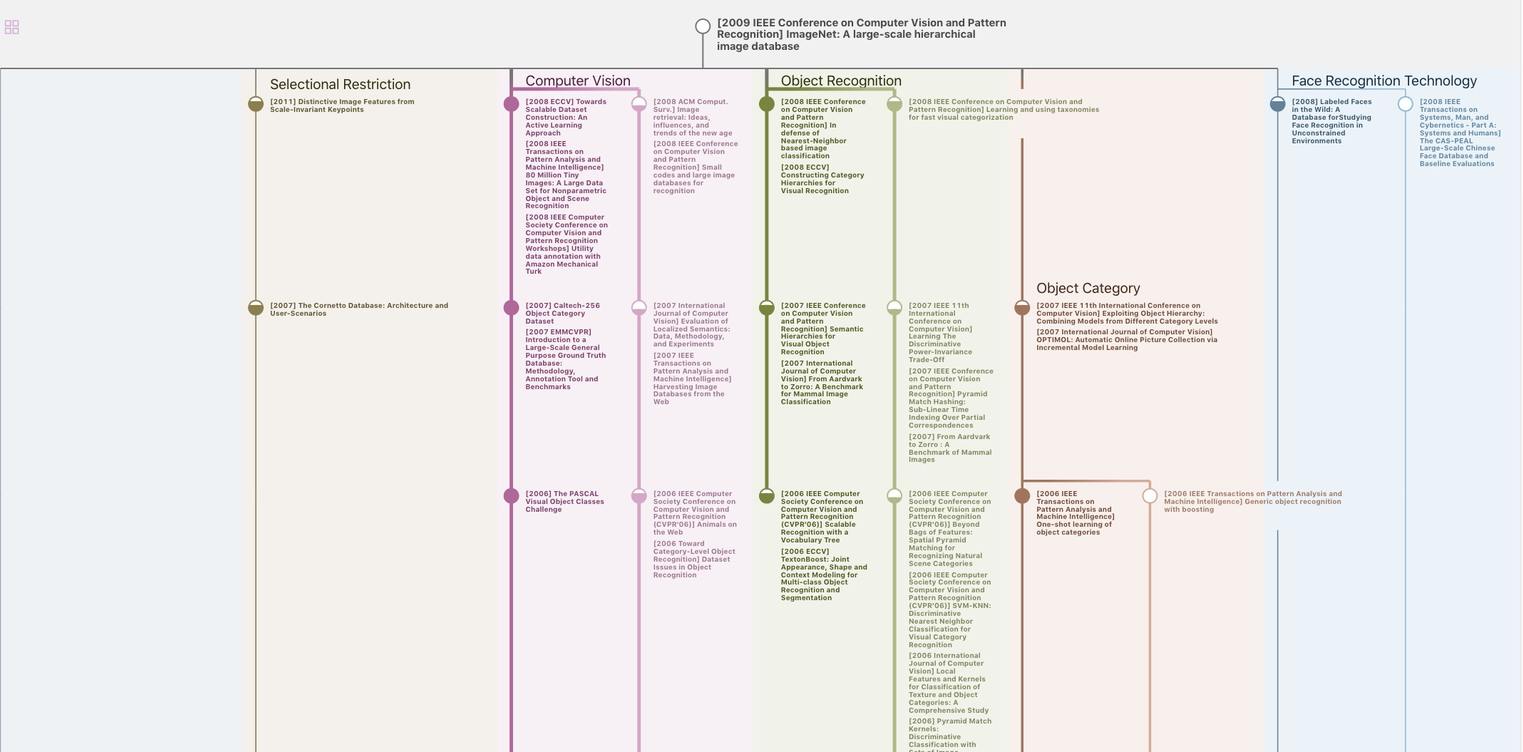
生成溯源树,研究论文发展脉络
Chat Paper
正在生成论文摘要