Understanding Selection and Diversity for Evolution of Spiking Recurrent Neural Networks
2018 INTERNATIONAL JOINT CONFERENCE ON NEURAL NETWORKS (IJCNN)(2018)
摘要
Evolutionary optimization or genetic algorithms have been used to optimize a variety of neural network types, including spiking recurrent neural networks, and are attractive for many reasons. However, a key impediment to their widespread use is the potential for slow training times and failure to converge to a good fitness value in a reasonable amount of time. In this work, we evaluate the effect of different selection algorithms on the performance of an evolutionary optimization method for designing spiking recurrent neural networks, including those that are meant to be deployed in a neuromorphic system. We propose a selection approach that utilizes a richer understanding of the fitness of an individual network to inform the selection process and to promote diversity in the population. We show that including this feature can provide a significant increase in performance over utilizing a standard selection approach.
更多查看译文
关键词
evolutionary optimization method,spiking recurrent neural networks,neuromorphic system,genetic algorithms,training times,selection algorithms
AI 理解论文
溯源树
样例
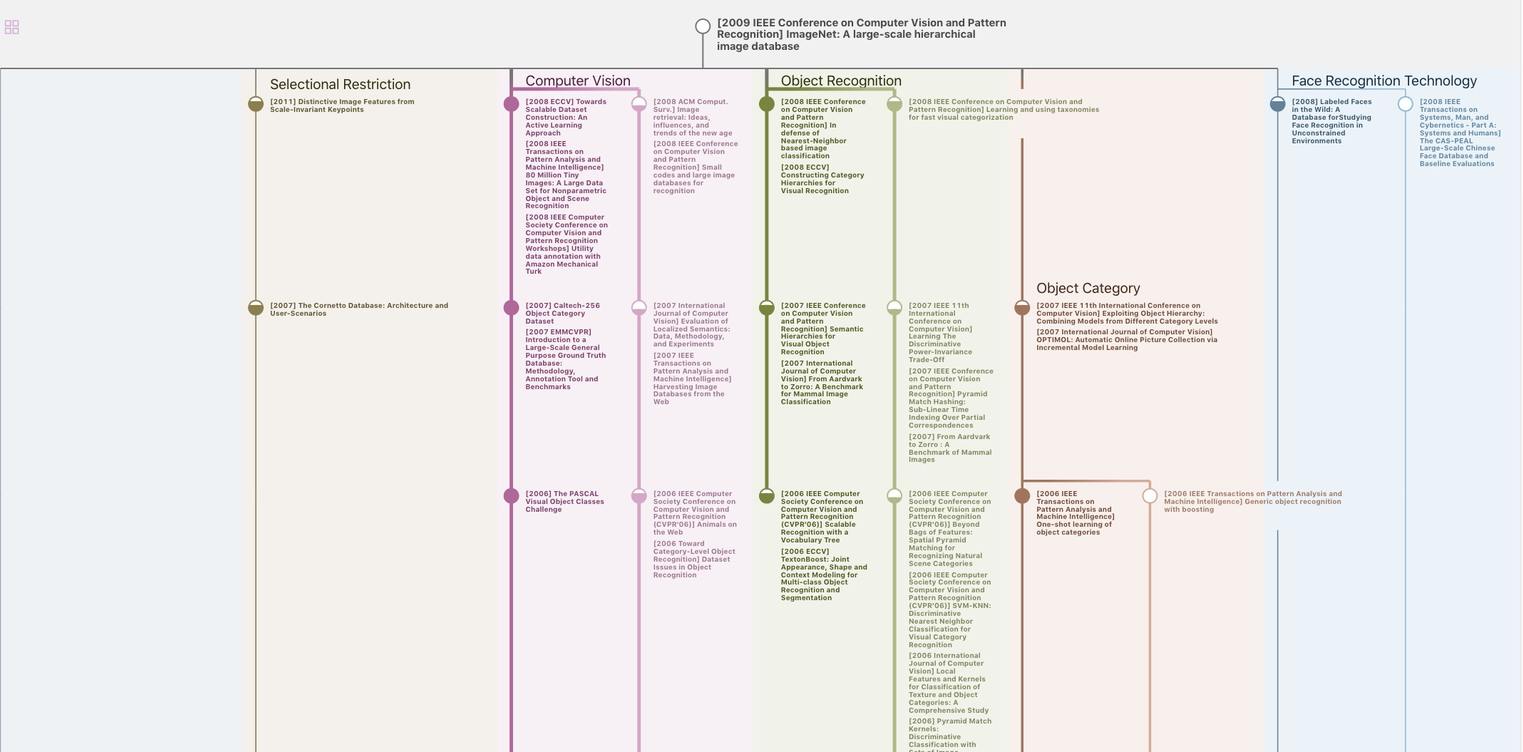
生成溯源树,研究论文发展脉络
Chat Paper
正在生成论文摘要