Static And Dynamic Ensembles Of Neural Networks For Solar Power Forecasting
2018 INTERNATIONAL JOINT CONFERENCE ON NEURAL NETWORKS (IJCNN)(2018)
摘要
Accurate forecasting of the power generated by PhotoVoltaic (PV) systems is needed for the successful integration of solar power into the electricity grid. In this paper, we consider the task of simultaneously forecasting the PV power output for the next day at half-hourly intervals using only previous PV power data. We propose a number of methods for constructing static and dynamic ensembles of neural networks. The static ensembles are based on random sampling and random feature selection, and the dynamic ones adaptively weight the contribution of the ensemble members based on their recent performance. We conduct an evaluation using Australian solar PV data for two years, and compare the results with state-of-the-art single prediction models and classical ensemble models. The results show that the proposed static ensembles are beneficial, achieving higher accuracy than the single neural networks and the other single and ensemble models used for comparison. The dynamic ensemble versions further improve the accuracy, demonstrating the potential of adaptively combining the predictions of ensemble members for accurate solar power forecasting.
更多查看译文
关键词
solar power forecasting, neural network ensembles, random feature selection, random example sampling, dynamic ensembles
AI 理解论文
溯源树
样例
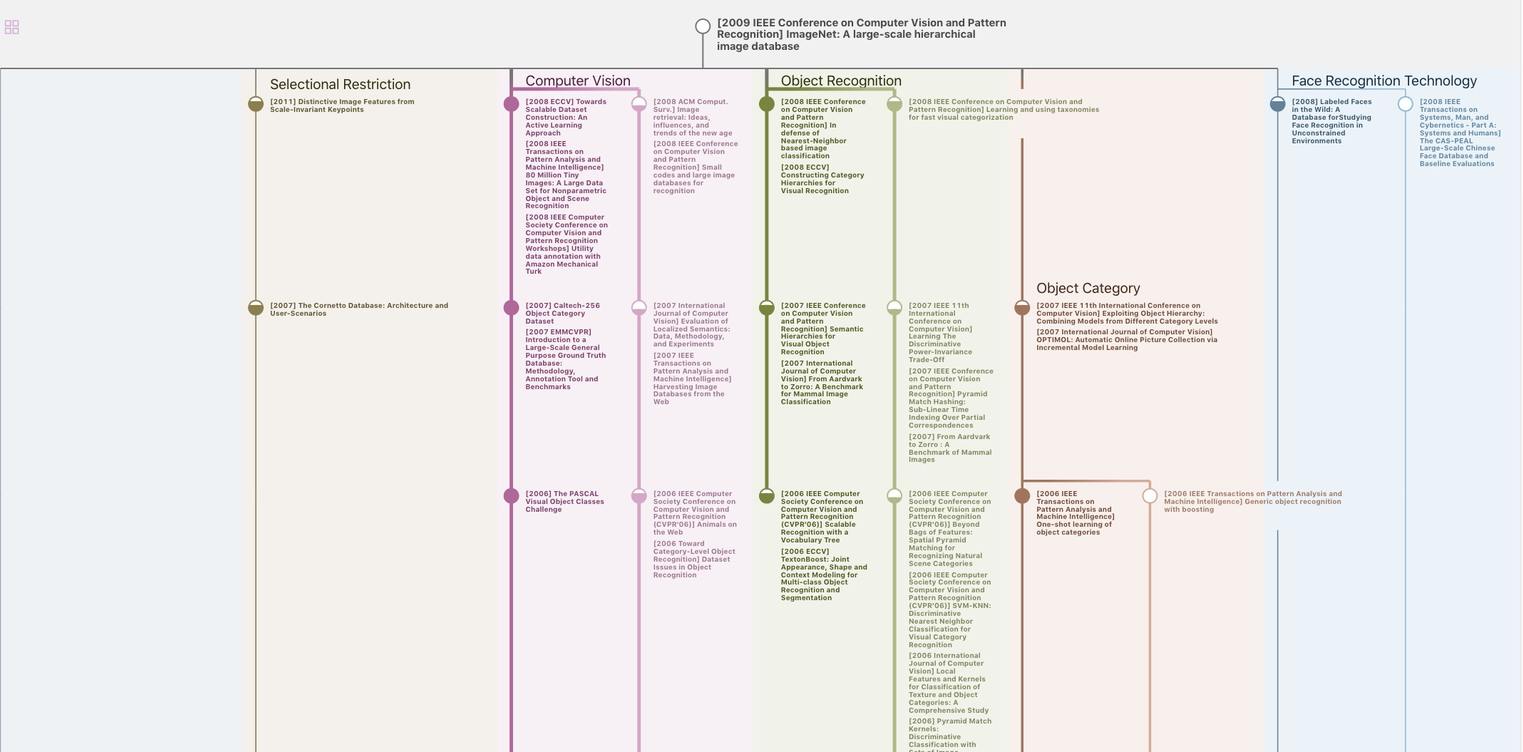
生成溯源树,研究论文发展脉络
Chat Paper
正在生成论文摘要