An Fpga-Based Som Circuit Architecture For Online Learning Of 64-Qam Data Streams
2018 INTERNATIONAL JOINT CONFERENCE ON NEURAL NETWORKS (IJCNN)(2018)
摘要
Responsible for fast transmission and large data volume, Quadrature Amplitude Modulation (QAM) has been applied in many communication systems, which explains the great interest in finding compatible techniques to improve its behavior. Kohonen Self-Organizing Maps (SOM) were implemented in a FPGA circuit to detect 64-QAM symbols. The use of SOM in the Quadrature/In-phase pair detection allowed the continuous adjustment to the QAM constellation with no supervision, as required in some adaptive communication systems. Bandwidth can thus be saved and training data retransmission was no longer necessary. The parallelism provided by FPGA processing showed to be compatible to the state-of-the-art works, presenting a performance of 8.05 GCUPs combined with a low percentage of hardware use (similar to 7%). In addition, experiments with different physical channels data have been performed and the bit error rates (BER) are reported for several signal to noise ratios.
更多查看译文
关键词
Quadrature Amplitude Modulation, embedded neural systems, Self-organizing Maps, online learning, FPGA
AI 理解论文
溯源树
样例
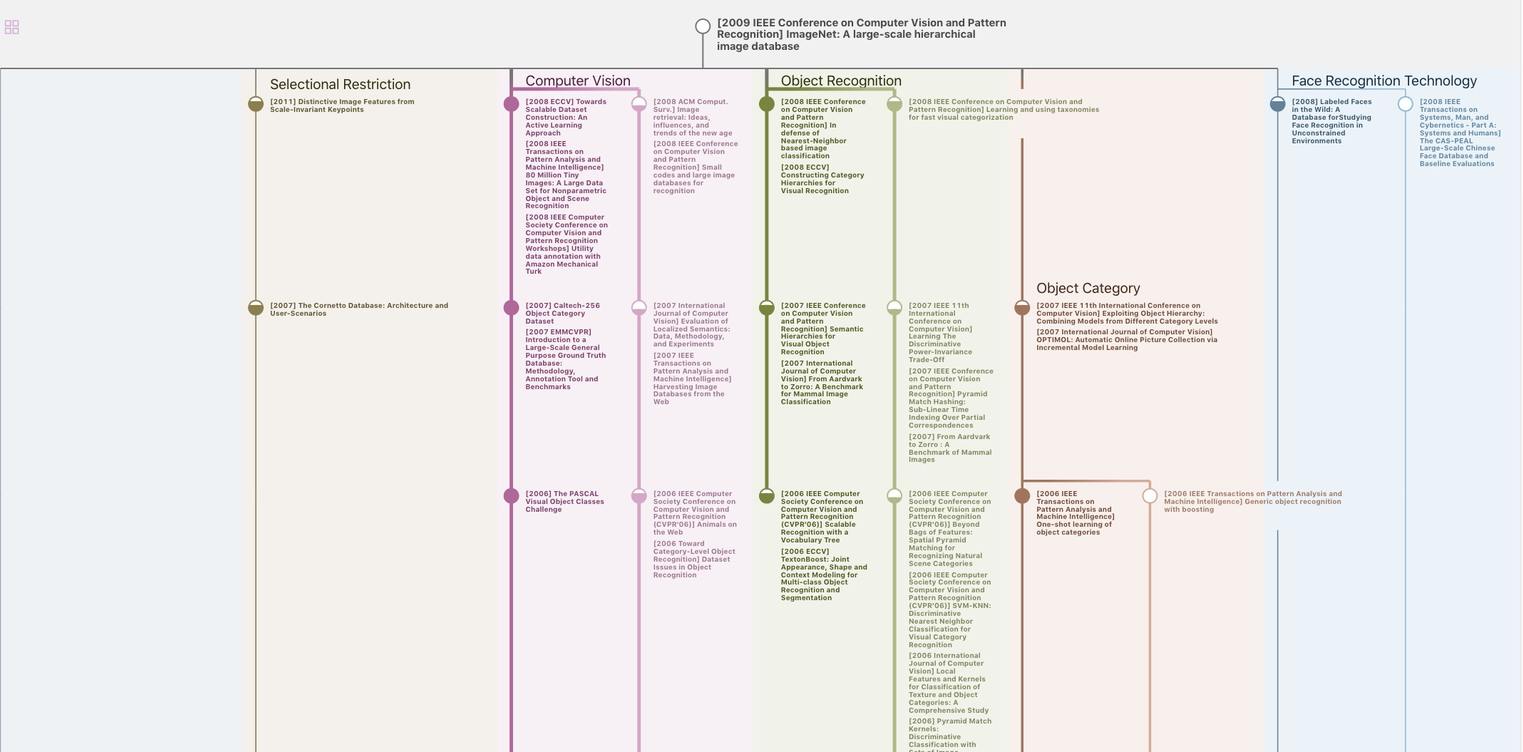
生成溯源树,研究论文发展脉络
Chat Paper
正在生成论文摘要