Emergent Turing Machine As A General Purpose Approximator
2018 INTERNATIONAL JOINT CONFERENCE ON NEURAL NETWORKS (IJCNN)(2018)
摘要
An autonomous navigation agent needs to learn the navigation rules and the transition among different navigation states, which can be summarized in Turing Machine (TM) controlled by a navigation Finite Automata. However, real-world inputs to this TM is always noisy with numerous appearance variances and distractors, which made it impossible to handcraft symbolic representations or features to detect. Developmental Network-1 (DN-1) incrementally learns a Finite Automata error-free using emergent representation but lacks hierarchy in its internal representation. In this paper, we extend DN-1 by adding lateral connection and multiple types of neurons to form hierarchical representation both internally and in the motor area. The formed hierarchical representation is more robust against distractors compared to the global feature patches in DN-1. Compared to other FA based methods, DN-2 directly uses natural input without handcrafting. The navigation states are emergent from the firing patterns in network areas instead of the hand-crafted symbolic states in the neural network output layer. Real-world and simulated navigation experiments showed that DN-2 successfully approximated rules of navigation using natural inputs. The agent learns different levels of concepts from simple to complex with the help of internal hierarchical representation.
更多查看译文
关键词
DN-1,hand-crafted symbolic states,neural network output layer,internal hierarchical representation,general purpose approximator,autonomous navigation agent,navigation rules,symbolic representations,Developmental Network-l,Turing Machine,navigation finite automata
AI 理解论文
溯源树
样例
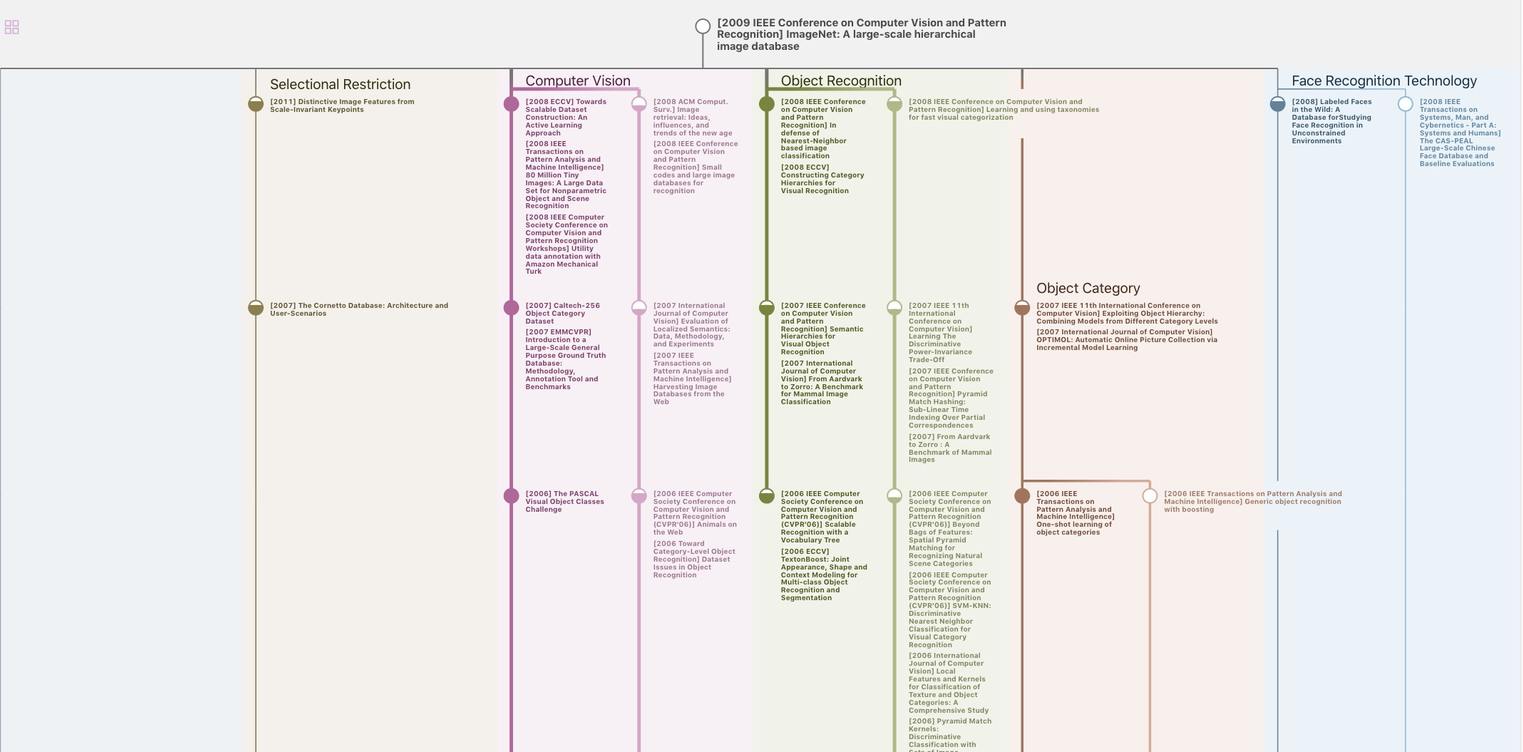
生成溯源树,研究论文发展脉络
Chat Paper
正在生成论文摘要