Adaptive Incremental Gaussian Mixture Network For Non-Stationary Data Stream Classification
2018 INTERNATIONAL JOINT CONFERENCE ON NEURAL NETWORKS (IJCNN)(2018)
摘要
Data stream classification poses many challenges for the data mining community when the environment is non-stationary, among which adaptation to the concept drifts, i.e., changes in the underlying concepts, is a major one. Two main ways to develop adaptive approaches are ensemble methods and incremental algorithms. Ensemble methods play an important role due to its modularity, which provides a natural way of adapting to change. Incremental algorithms are faster and have better anti-noise capacity than ensemble algorithms, but have more restrictions on concept drifting data streams. Thus, it is a challenge to combine the flexibility and adaptation of an ensemble classifier in the presence of concept drift, with the simplicity of use found in a single classifier with incremental learning. With this motivation, this work proposes an incremental, online, and probabilistic algorithm for classification as an effort of tackling concept drifting in data streams. The algorithm is called Incremental Gaussian Mixture Network for Non-Stationary Environments (IGMN-NSE) and is an adaptation of the IGMN algorithm. The two main contributions of IGMN-NSE in relation to the IGMN are predictive power improvement for classification tasks and adaptation to achieve a good performance in non-stationary environments. Extensive experiments with both synthetic and real-world data demonstrate that the IGMN-NSE can track the changing environments very closely, regardless of the type of concept drift.
更多查看译文
关键词
Data streams, classification tasks, concept-drift, incremental learning, Gaussian mixture models
AI 理解论文
溯源树
样例
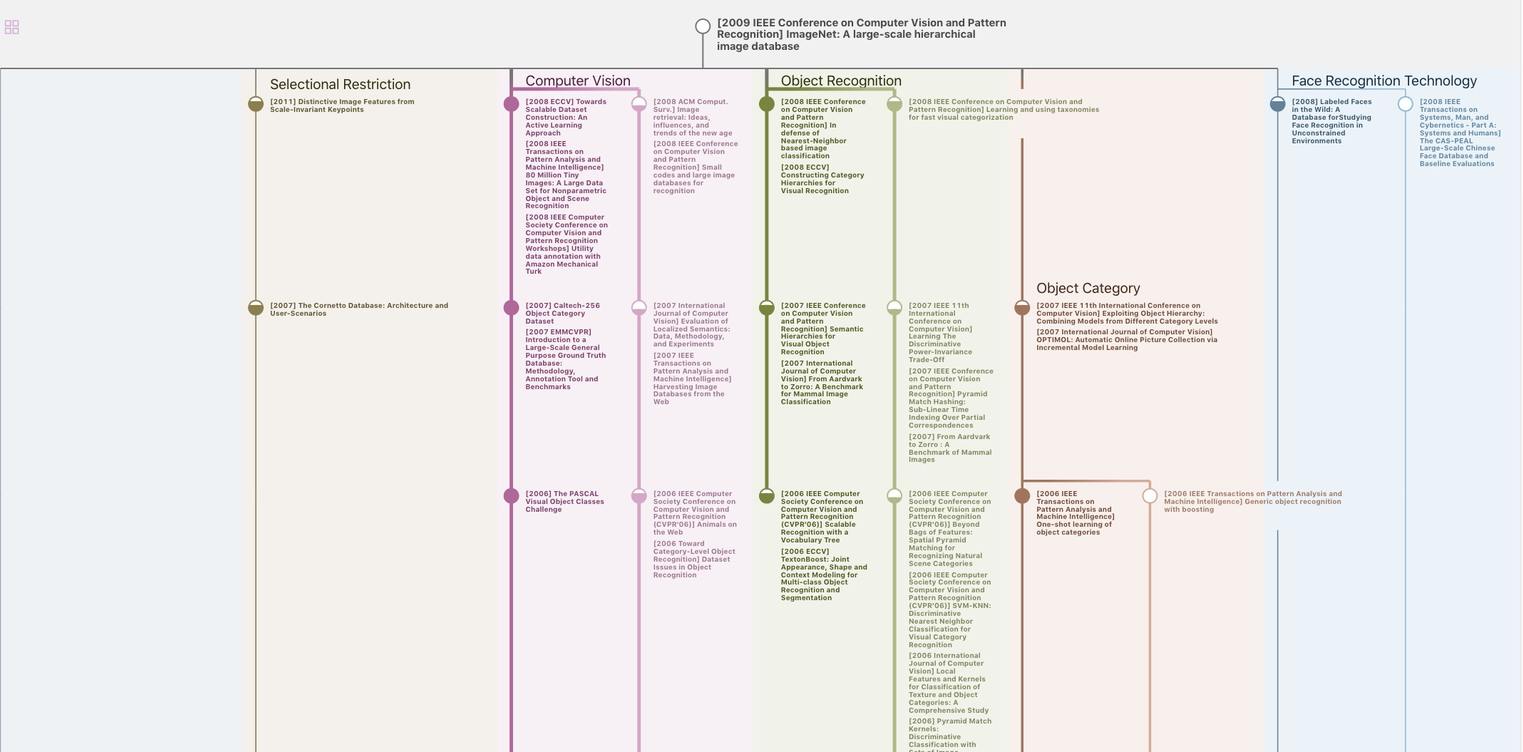
生成溯源树,研究论文发展脉络
Chat Paper
正在生成论文摘要