The Optimized Selection Of Base-Classifiers For Ensemble Classification Using A Multi-Objective Genetic Algorithm
2018 INTERNATIONAL JOINT CONFERENCE ON NEURAL NETWORKS (IJCNN)(2018)
摘要
We propose an ensemble classification algorithm that uses multi-objective optimization instead of relying on heuristics or fragile user-defined parameters. Only two user-defined parameters are included, with both being found to have large windows of values that produce statistically indistinguishable results, indicating the low level of expertise required from the user to achieve good results. Additionally, when given a large initial set of trained base-classifiers, we demonstrate that a multi-objective genetic algorithm aiming to optimize prediction accuracy and diversity will prefer particular types of classifiers over others. The total number of chosen classifiers is also surprisingly small only 5.86 classifiers on average, out of a starting pool of 900. This occurs without any explicit preference for small ensembles of classifiers. Even with these small ensembles, significantly lower empirical classification error is achieved compared to the current state-of-the-art.
更多查看译文
关键词
ensemble classification, multi-objective optimization, genetic algorithm, bagging, multiple classifiers, classifier selection, diversity, double-fault measure
AI 理解论文
溯源树
样例
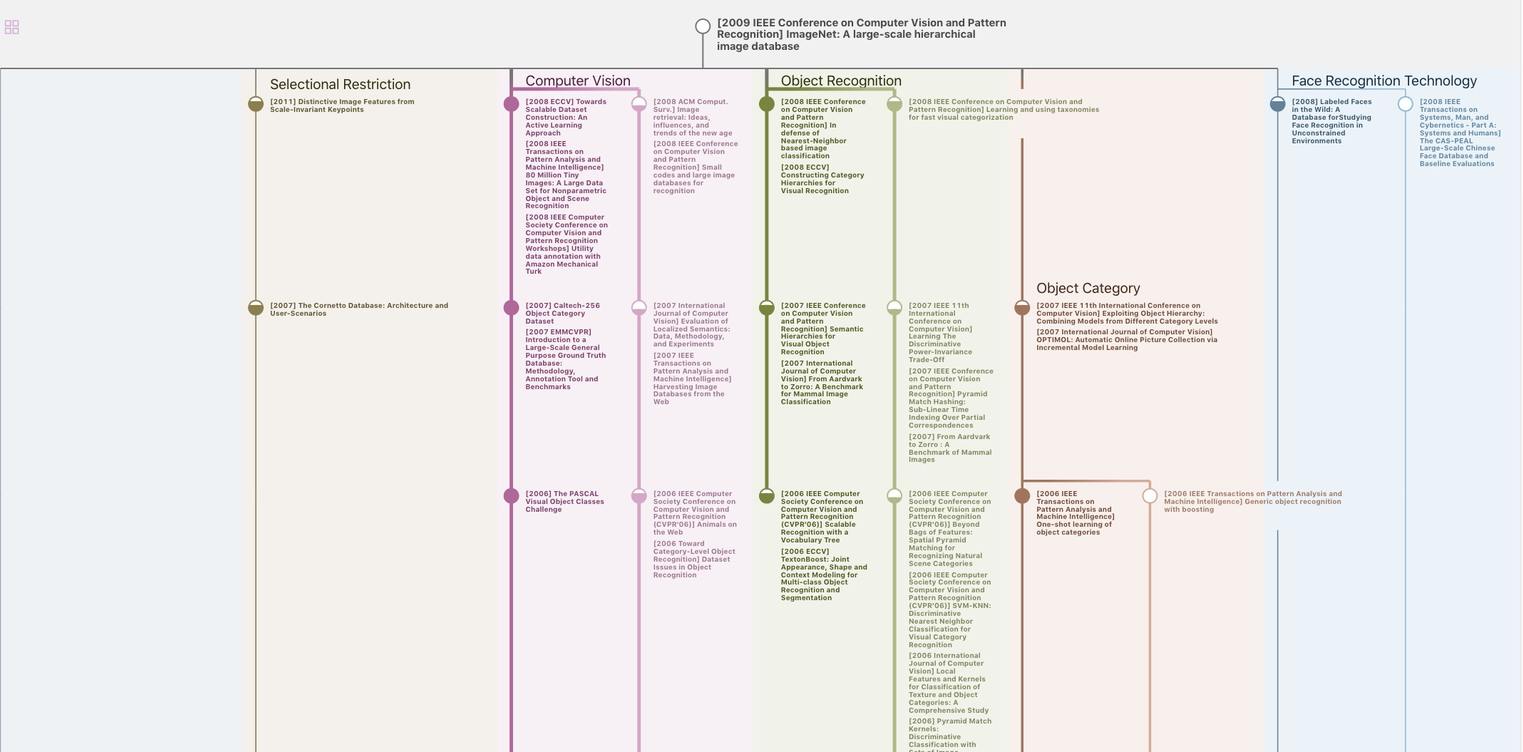
生成溯源树,研究论文发展脉络
Chat Paper
正在生成论文摘要