Massively parallel skyline computation for processing-in-memory architectures
PACT(2018)
摘要
Processing-In-Memory (PIM) is an increasingly popular architecture aimed at addressing the 'memory wall' crisis by prioritizing the integration of processors within DRAM. It promotes low data access latency, high bandwidth, massive parallelism, and low power consumption. The skyline operator is a known primitive used to identify those multi-dimensional points offering optimal trade-offs within a given dataset. For large multidimensional dataset, calculating the skyline is extensively compute and data intensive. Although, PIM systems present opportunities to mitigate this cost, their execution model relies on all processors operating in isolation with minimal data exchange. This prohibits direct application of known skyline optimizations which are inherently sequential, creating dependencies and large intermediate results that limit the maximum parallelism, throughput, and require an expensive merging phase.
In this work, we address these challenges by introducing the first skyline algorithm for PIM architectures, called DSky. It is designed to be massively parallel and throughput efficient by leveraging a novel work assignment strategy that emphasizes load balancing. Our experiments demonstrate that it outperforms the state-of-the-art algorithms for CPUs and GPUs, in most cases. DSky achieves 2× to 14× higher throughput compared to the state-of-the-art solutions on competing CPU and GPU architectures. Furthermore, we showcase DSky's good scaling properties which are intertwined with PIM's ability to allocate resources with minimal added cost. In addition, we showcase an order of magnitude better energy consumption compared to CPUs and GPUs.
更多查看译文
关键词
processing-in-memory, skyline queries, pareto dominance, massive parallelism, processing-near-memory, load balancing
AI 理解论文
溯源树
样例
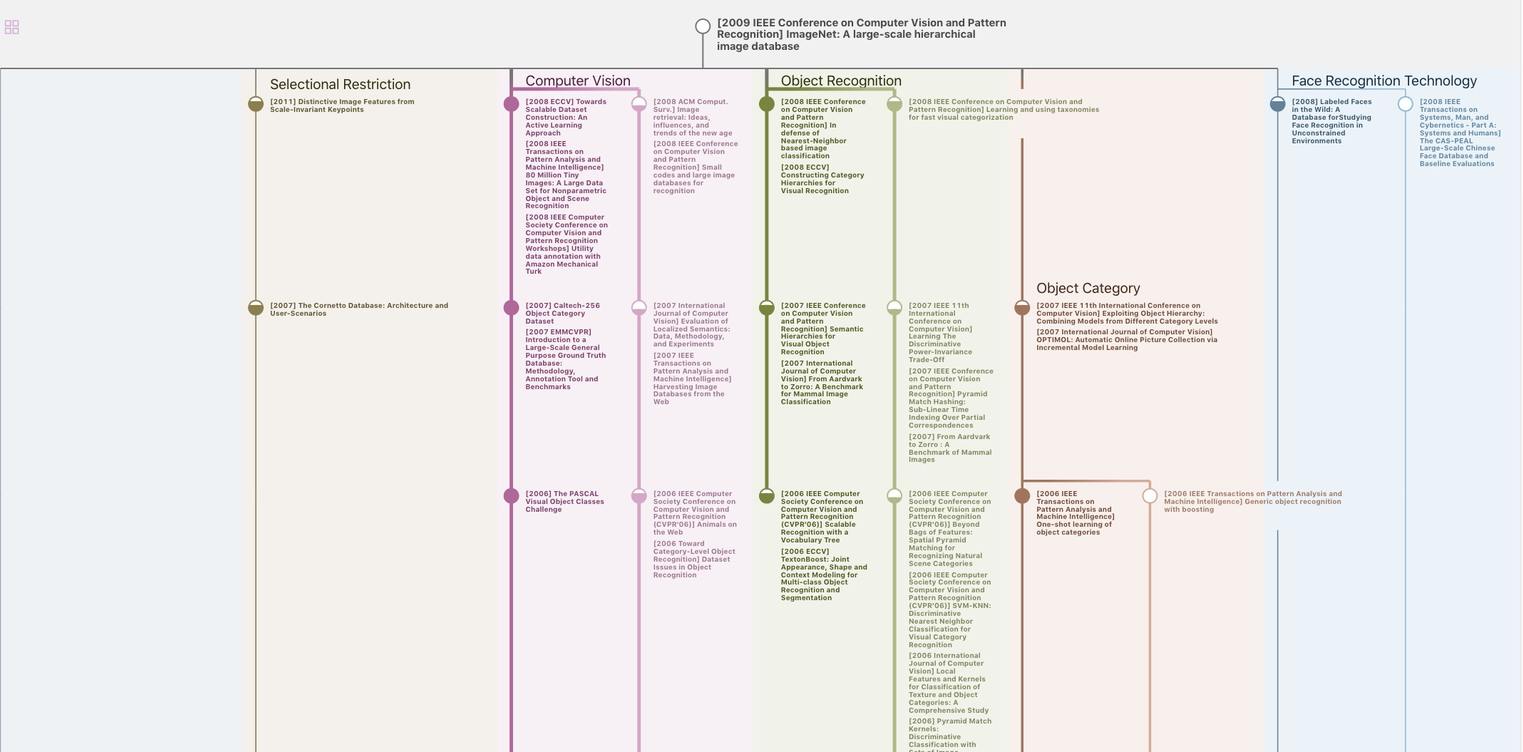
生成溯源树,研究论文发展脉络
Chat Paper
正在生成论文摘要