Self Hyper-Parameter Tuning for Data Streams.
DS(2018)
摘要
The widespread usage of smart devices and sensors together with the ubiquity of the Internet access is behind the exponential growth of data streams. Nowadays, there are hundreds of machine learning algorithms able to process high-speed data streams. However, these algorithms rely on human expertise to perform complex processing tasks like hyper-parameter tuning. This paper addresses the problem of data variability modelling in data streams. Specifically, we propose and evaluate a new parameter tuning algorithm called Self Parameter Tuning (SPT). SPT consists of an online adaptation of the Nelder u0026 Mead optimisation algorithm for hyper-parameter tuning. The method explores a dynamic size sample method to evaluate the current solution, and uses the Nelder u0026 Mead operators to update the current set of parameters. The main contribution is the adaptation of the Nelder-Mead algorithm to automatically tune regression hyper-parameters for data streams. Additionally, whenever concept drifts occur in the data stream, it re-initiates the search for new hyper-parameters. The proposed method has been evaluated on regression scenario. Experiments with well known time-evolving data streams show that the proposed SPT hyper-parameter optimisation outperforms the results of previous expert hyper-parameter tuning efforts.
更多查看译文
关键词
Parameter tuning, Hyper-parameters, Optimisation, Nelder-Mead, Regression
AI 理解论文
溯源树
样例
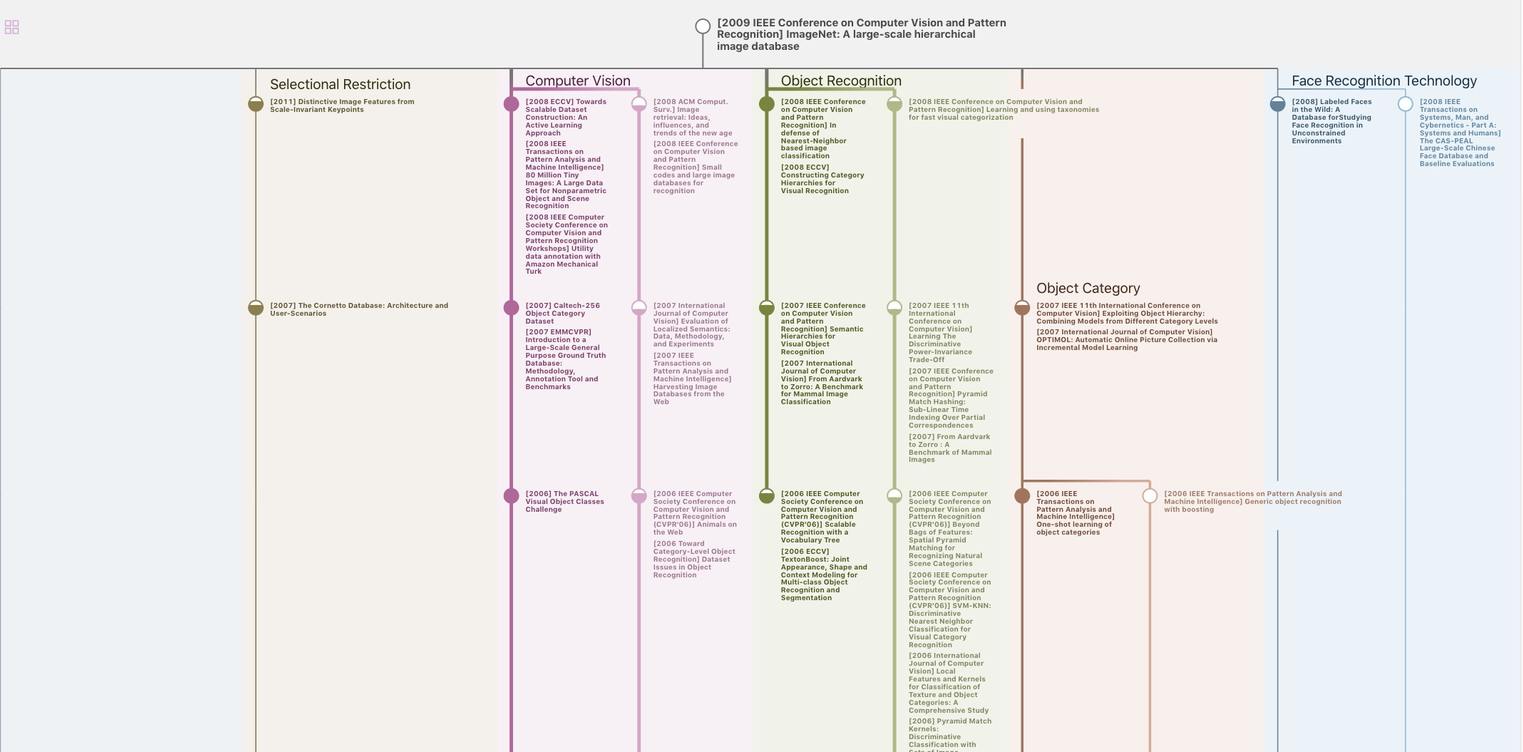
生成溯源树,研究论文发展脉络
Chat Paper
正在生成论文摘要