Adapting Hierarchical Multiclass Classification to Changes in the Target Concept.
ADVANCES IN ARTIFICIAL INTELLIGENCE, CAEPIA 2018(2018)
摘要
Machine learning models often need to be adapted to new contexts, for instance, to deal with situations where the target concept changes. In hierarchical classification, the modularity and flexibility of learning techniques allows us to deal directly with changes in the learning problem by readapting the structure of the model, instead of having to retrain the model from the scratch. In this work, we propose a method for adapting hierarchical models to changes in the target classes. We experimentally evaluate our method over different datasets. The results show that our novel approach improves the original model, and compared to the retraining approach, it performs quite competitive while it implies a significantly smaller computational cost.
更多查看译文
关键词
Hierarchical,Classification,Adaptation,Novelty
AI 理解论文
溯源树
样例
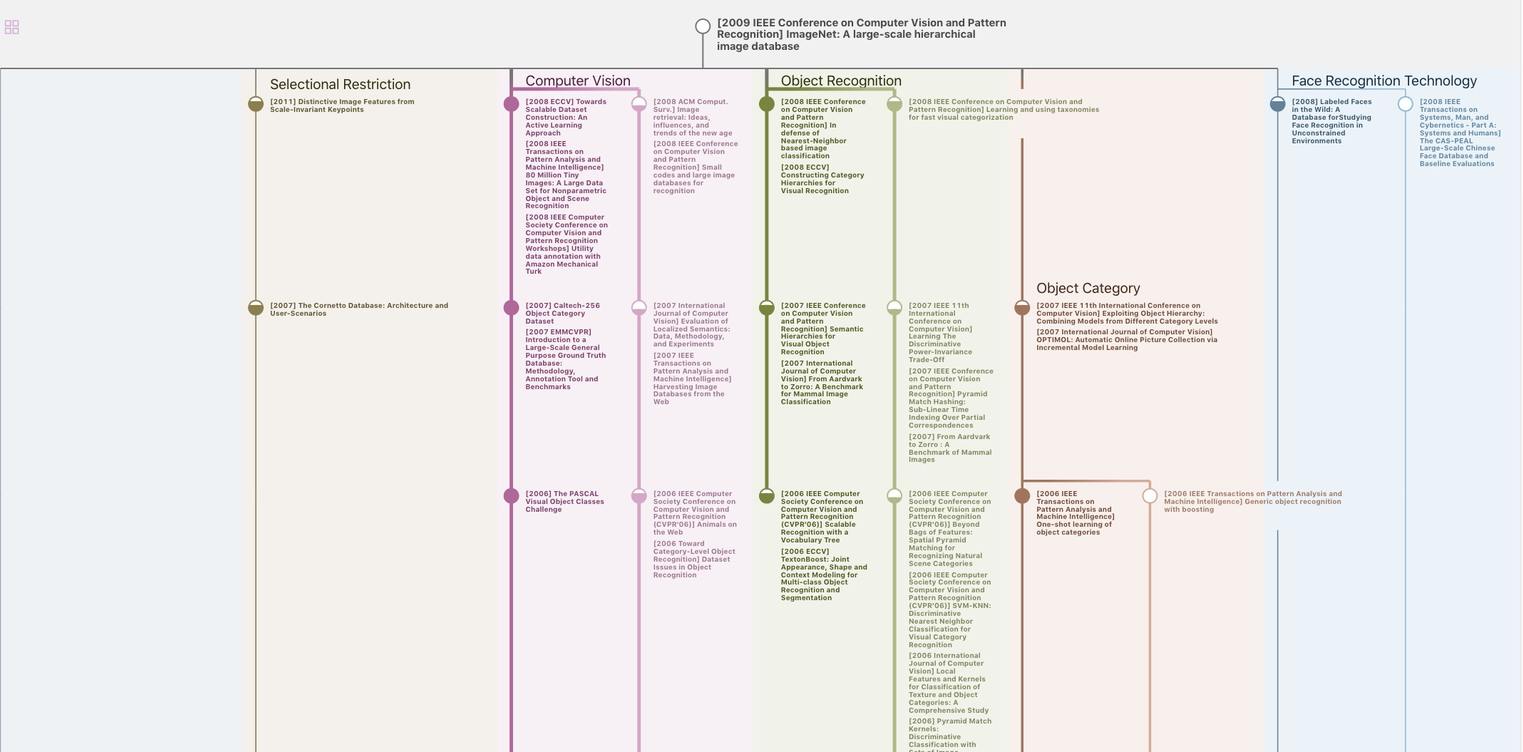
生成溯源树,研究论文发展脉络
Chat Paper
正在生成论文摘要