In-Session Personalization for Talent Search.
CIKM(2018)
摘要
Previous efforts in recommendation of candidates for talent search followed the general pattern of receiving an initial search criteria and generating a set of candidates utilizing a pre-trained model. Traditionally, the generated recommendations are final, that is, the list of potential candidates is not modified unless the user explicitly changes his/her search criteria. In this paper, we are proposing a candidate recommendation model which takes into account the immediate feedback of the user, and updates the candidate recommendations at each step. This setting also allows for very uninformative initial search queries, since we pinpoint the user's intent due to the feedback during the search session. To achieve our goal, we employ an intent clustering method based on topic modeling which separates the candidate space into meaningful, possibly overlapping, subsets (which we call intent clusters) for each position. On top of the candidate segments, we apply a multi-armed bandit approach to choose which intent cluster is more appropriate for the current session. We also present an online learning scheme which updates the intent clusters within the session, due to user feedback, to achieve further personalization. Our offline experiments as well as the results from the online deployment of our solution demonstrate the benefits of our proposed methodology.
更多查看译文
关键词
in session recommendations, talent search, model selection, online learning
AI 理解论文
溯源树
样例
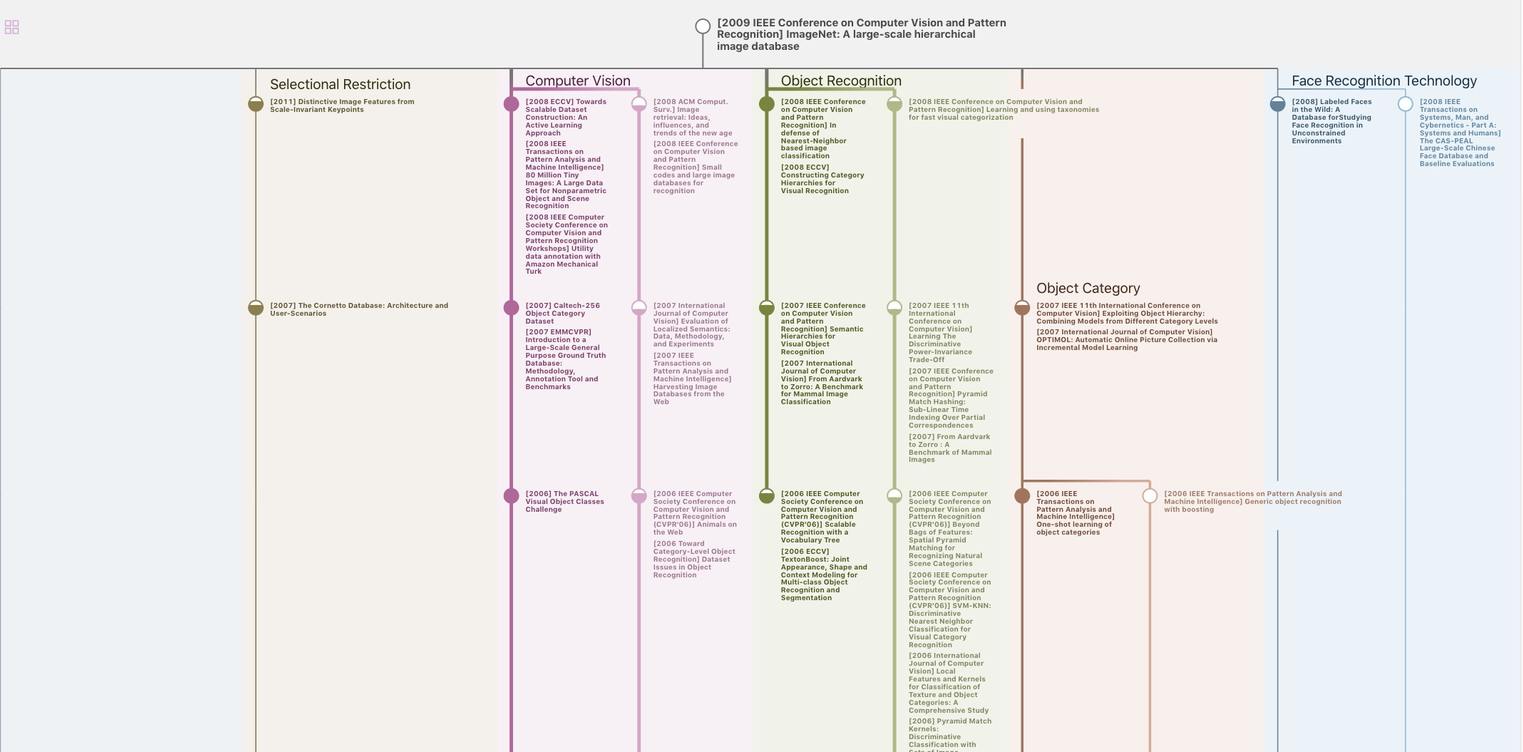
生成溯源树,研究论文发展脉络
Chat Paper
正在生成论文摘要