In Situ and Context-Aware Target Apps Selection for Unified Mobile Search.
CIKM(2018)
摘要
With the recent growth in the use of conversational systems and intelligent assistants such as Google Assistant and Microsoft Cortana, mobile devices are becoming even more pervasive in our lives. As a consequence, users are getting engaged with mobile apps and frequently search for an information need using different apps. Recent work has stated the need for a unified mobile search system that would act as meta search on users' mobile devices: it would identify the target apps for the user's query, submit the query to the apps, and present the results to the user. Moreover, mobile devices provide rich contextual information about users and their whereabouts. In this paper, we introduce the task of context-aware target apps selection as part of a unified mobile search framework. To this aim, we designed an in situ study to collect thousands of mobile queries enriched with mobile sensor data from 255 users during a three month period. With the aid of this dataset, we were able to study user behavior as they performed cross-app search. We finally study the performance of state-of-the-art retrieval models for this task and propose a simple yet effective neural model that significantly outperforms the baselines. Our neural approach is based on learning high-dimensional representations for mobile apps and contextual information. Furthermore, we show that incorporating context improves the performance by 20% in terms of [email protected], enabling the model to perform better for 57% of users. Our data is publicly available for research purposes.
更多查看译文
AI 理解论文
溯源树
样例
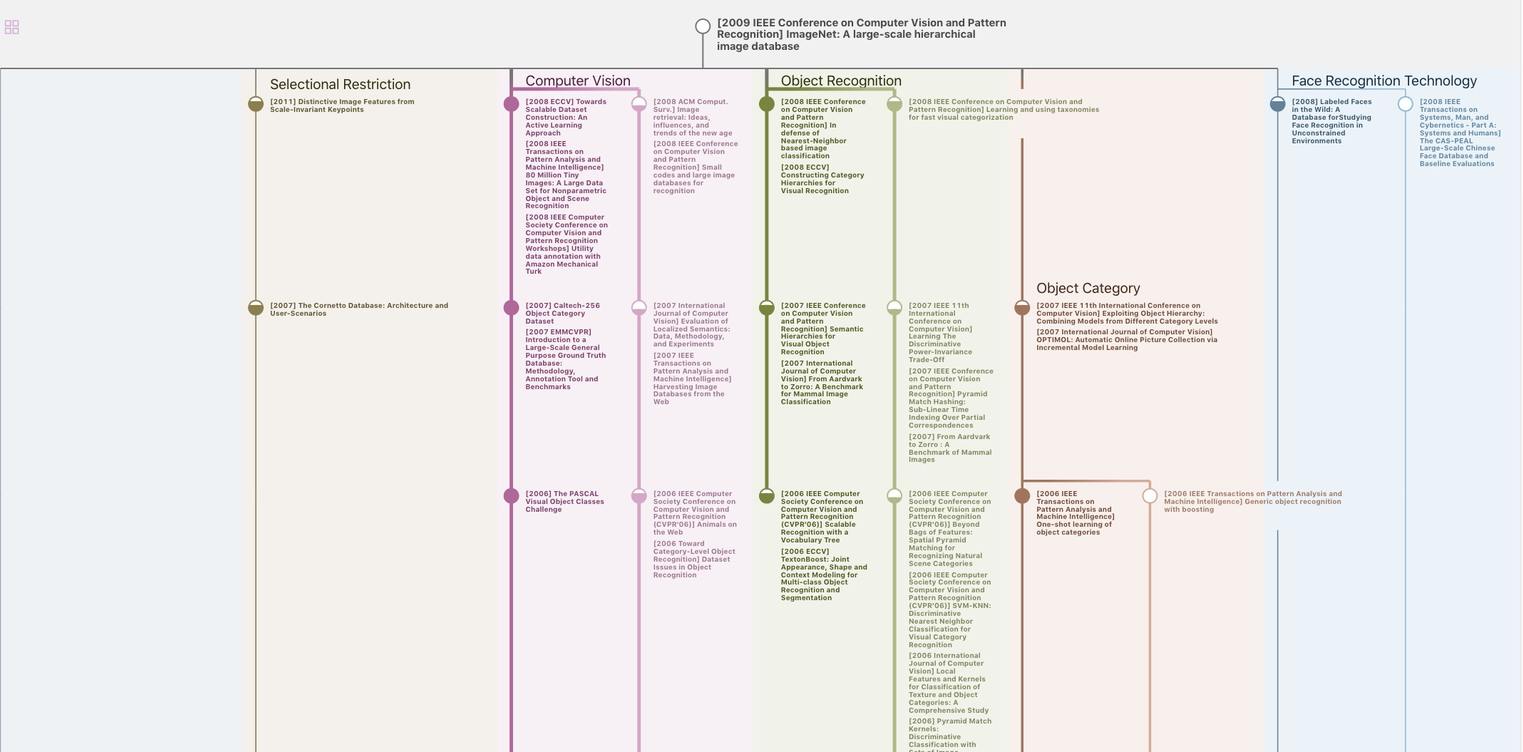
生成溯源树,研究论文发展脉络
Chat Paper
正在生成论文摘要