Substituting Missing Values In End-To-End Internet Performance Measurements Using K-Nearest Neighbors
2018 16TH IEEE INT CONF ON DEPENDABLE, AUTONOM AND SECURE COMP, 16TH IEEE INT CONF ON PERVAS INTELLIGENCE AND COMP, 4TH IEEE INT CONF ON BIG DATA INTELLIGENCE AND COMP, 3RD IEEE CYBER SCI AND TECHNOL CONGRESS (DASC/PICOM/DATACOM/CYBERSCITECH)(2018)
摘要
PingER (Ping End-to-end Reporting) is a worldwide end-to-end Internet performance measurement framework running for the last 20 years and led by the SLAC National Accelerator Laboratory USA. The objective of the project is to monitor the performance of the Internet links around the world using the ubiquitous ping facility. Currently, the framework comprises of about 50 active Monitoring Agents (MAs) in 20 countries of the world. These MAs probe 700 remote sites located in 170 countries of the world. They are covering an area containing over 98% of the world's population. Currently, the size of the PingER data is about 60 GB stored in 100,000 flat files with a compression ratio of 5:1. The data is of an historical nature and very useful for fine-grained Internet performance analysis. However, the data contains missing values due to congestion, queuing overflow, faulty hardware or software and unavailability of MAs & remote sites. These missing values affect the quality of the Internet performance analysis. The objective of this paper is to substitute the missing values using the k-Nearest Neighbors algorithm (k-NN) and compare the estimation with the statistical method. Therefore, PingER historical data is first transformed into CSV format using a PingER data dimensional model. Afterward, missing values are imputed, using the statistical method and the k-NN algorithm, on data containing the different percentages of missing values. The results conclude that the k-NN algorithm is best suited for the substitution of missing values in the PingER data as compared to the method based on the statistical procedure.
更多查看译文
关键词
Internet performance monitoring, PingER, missing value, k-Nearest Neighbors
AI 理解论文
溯源树
样例
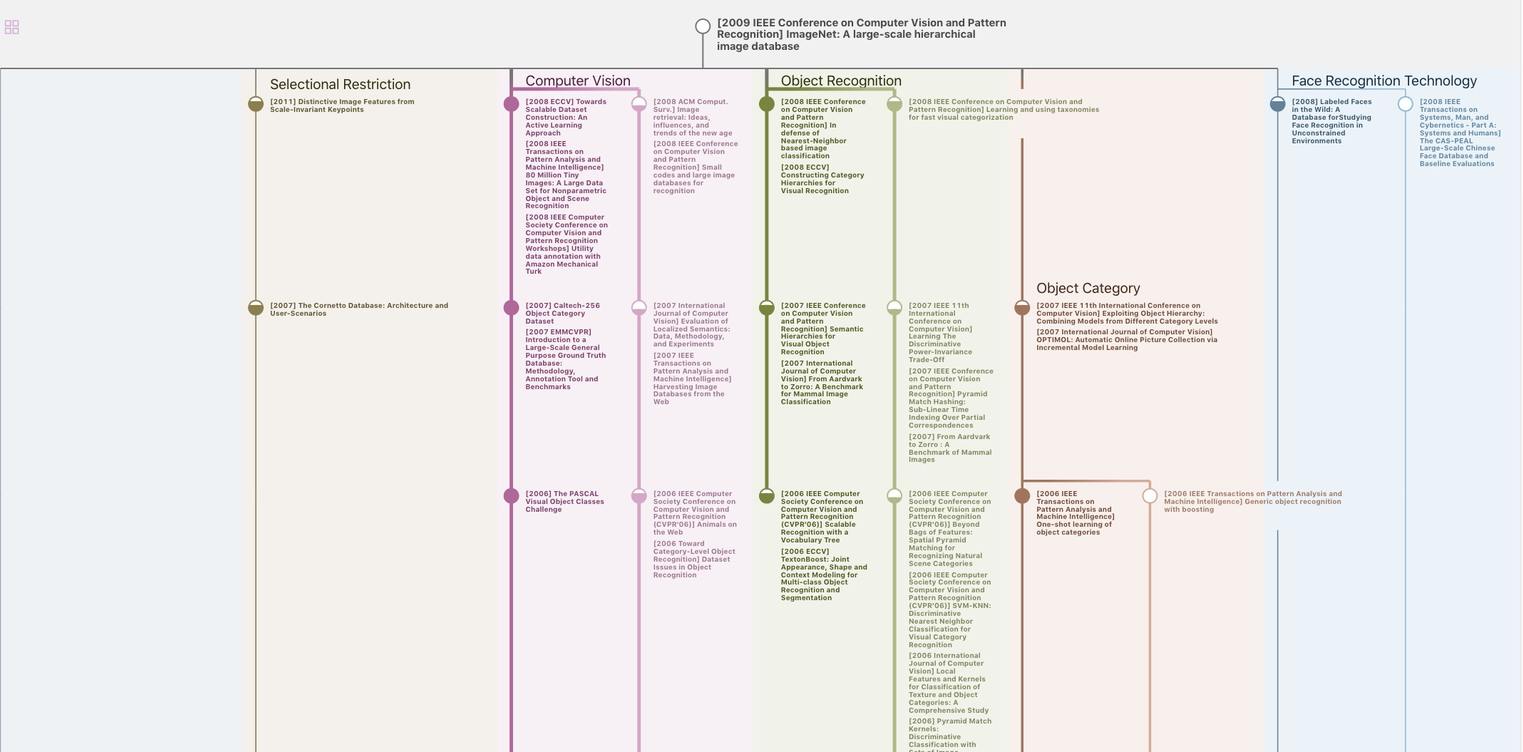
生成溯源树,研究论文发展脉络
Chat Paper
正在生成论文摘要