Forward Model Approximation for General Video Game Learning
2018 IEEE Conference on Computational Intelligence and Games (CIG)(2018)
摘要
This paper proposes a novel learning agent model for a General Video Game Playing agent. Our agent learns an approximation of the forward model from repeatedly playing a game and subsequently adapting its behavior to previously unseen levels. To achieve this, it first learns the game mechanics through machine learning techniques and then extracts rule-based symbolic knowledge on different levels of abstraction. When being confronted with new levels of a game, the agent is able to revise its knowledge by a novel belief revision approach. Using methods such as Monte Carlo Tree Search and Breadth First Search, it searches for the best possible action using simulated game episodes. Those simulations are only possible due to reasoning about future states using the extracted rule-based knowledge from random episodes during the learning phase. The developed agent outperforms previous agents by a large margin, while still being limited in its prediction capabilities. The proposed forward model approximation opens a new class of solutions in the context of General Video Game Playing, which do not try to learn a value function, but try to increase their accuracy in modelling the game.
更多查看译文
关键词
Forward Model Approximation,General Video Games,exception-tolerant Hierarchical Knowledge Bases,Belief Revision,Monte Carlo Tree Search,Breadth First Search
AI 理解论文
溯源树
样例
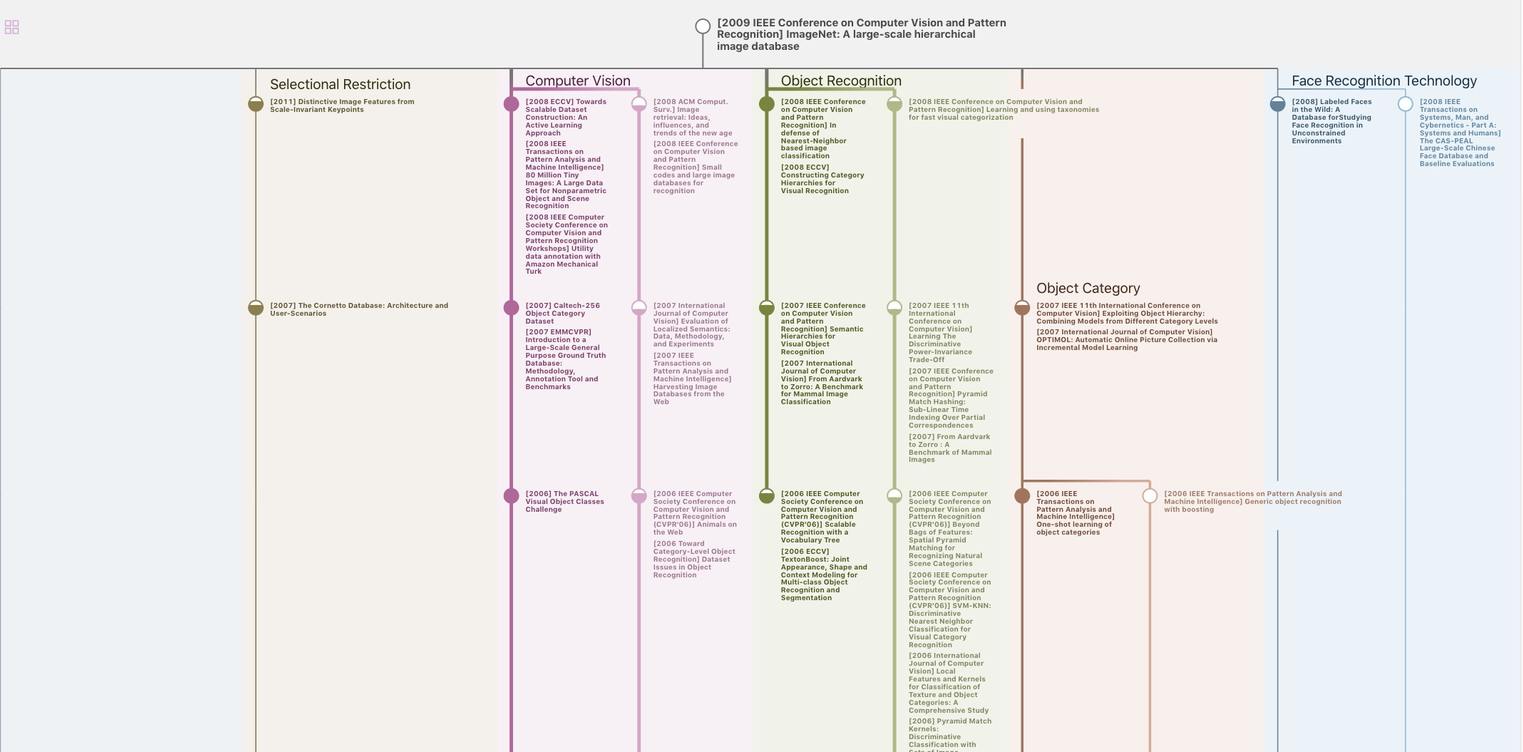
生成溯源树,研究论文发展脉络
Chat Paper
正在生成论文摘要