Using Object Information For Spotting Text
COMPUTER VISION - ECCV 2018, PT XVI(2018)
摘要
Text spotting, also called text detection, is a challenging computer vision task because of cluttered backgrounds, diverse imaging environments, various text sizes and similarity between some objects and characters, e.g., tyre and `o'. However, text spotting is a vital step in numerous AI and computer vision systems, such as autonomous robots and systems for visually impaired. Due to its potential applications and commercial values, researchers have proposed various deep architectures and methods for text spotting. These methods and architectures concentrate only on text in images, but neglect other information related to text. There exists a strong relationship between certain objects and the presence of text, such as signboards or the absence of text, such as trees. In this paper, a text spotting algorithm based on text and object dependency is proposed. The proposed algorithm consists of two subconvolutional neural networks and three training stages. For this study, a new NTU-UTOI dataset containing over 22k non-synthetic images with 277k bounding boxes for text and 42 text-related object classes is established. According to our best knowledge, it is the second largest nonsynthetic text image database. Experimental results on three benchmark datasets with clutter backgrounds, COCO-Text, MSRA-TD500 and SVT show that the proposed algorithm provides comparable performance to state-of-the-art text spotting methods. Experiments are also performed on our newly established dataset to investigate the effectiveness of object information for text spotting. The experimental results indicate that the object information contributes significantly on the performance gain.
更多查看译文
关键词
Text detection, Natural Scenes, Deep learning, Object detection, RCNN
AI 理解论文
溯源树
样例
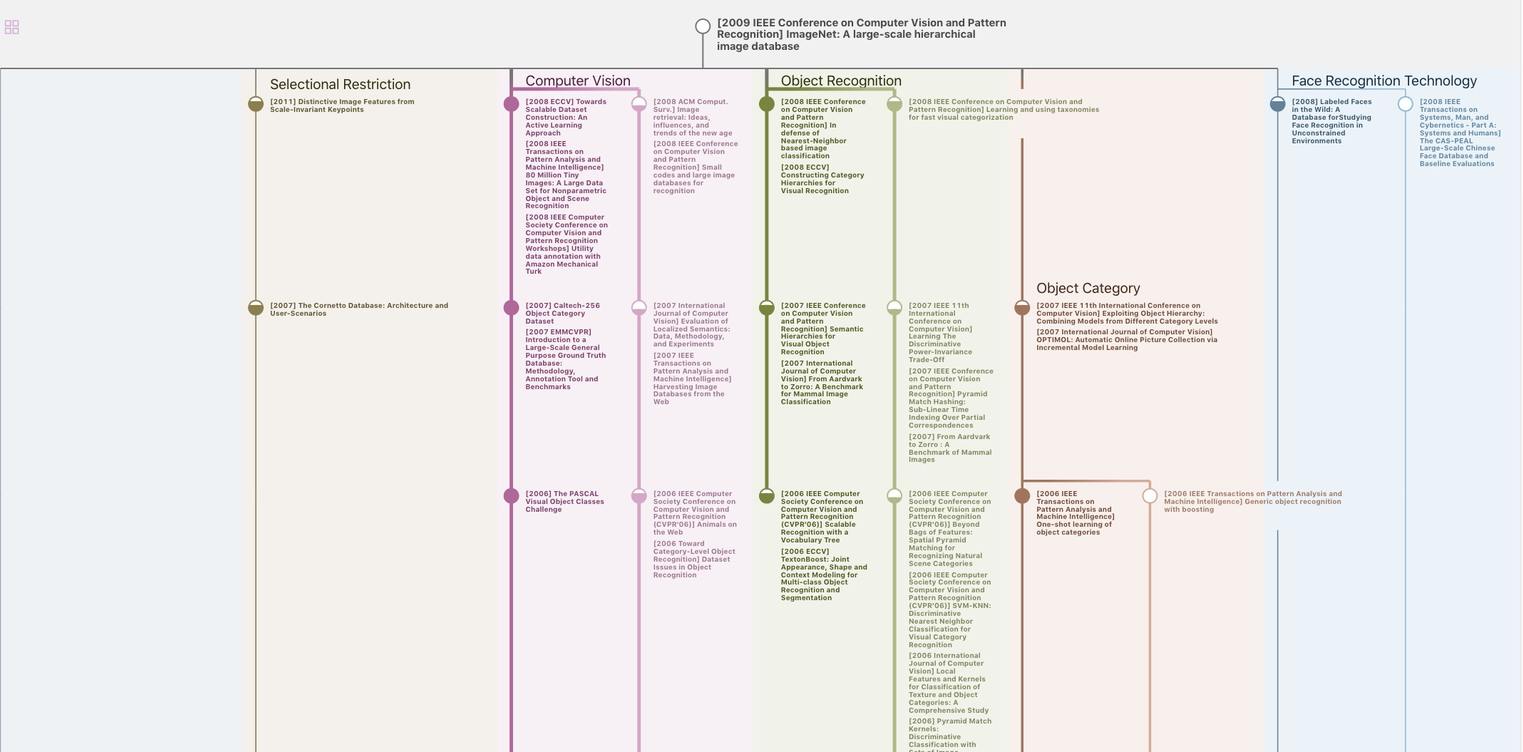
生成溯源树,研究论文发展脉络
Chat Paper
正在生成论文摘要