R2p2: A Reparameterized Pushforward Policy For Diverse, Precise Generative Path Forecasting
COMPUTER VISION - ECCV 2018, PT XIII(2018)
摘要
We propose a method to forecast a vehicle's ego-motion as a distribution over spatiotemporal paths, conditioned on features (e.g., from LIDAR and images) embedded in an overhead map. The method learns a policy inducing a distribution over simulated trajectories that is both "diverse" (produces most of the likely paths) and "precise" (mostly produces likely paths). This balance is achieved through minimization of a symmetrized cross-entropy between the distribution and demonstration data. By viewing the simulated-outcome distribution as the pushforward of a simple distribution under a simulation operator, we obtain expressions for the cross-entropy metrics that can be efficiently evaluated and differentiated, enabling stochastic-gradient optimization. We propose concrete policy architectures for this model, discuss our evaluation metrics relative to previously-used degenerate metrics, and demonstrate the superiority of our method relative to state-of-the-art methods in both the KITTI dataset and a similar but novel and larger real-world dataset explicitly designed for the vehicle forecasting domain.
更多查看译文
关键词
Trajectory forecasting, Imitation learning, Generative modeling, Self-driving vehicles
AI 理解论文
溯源树
样例
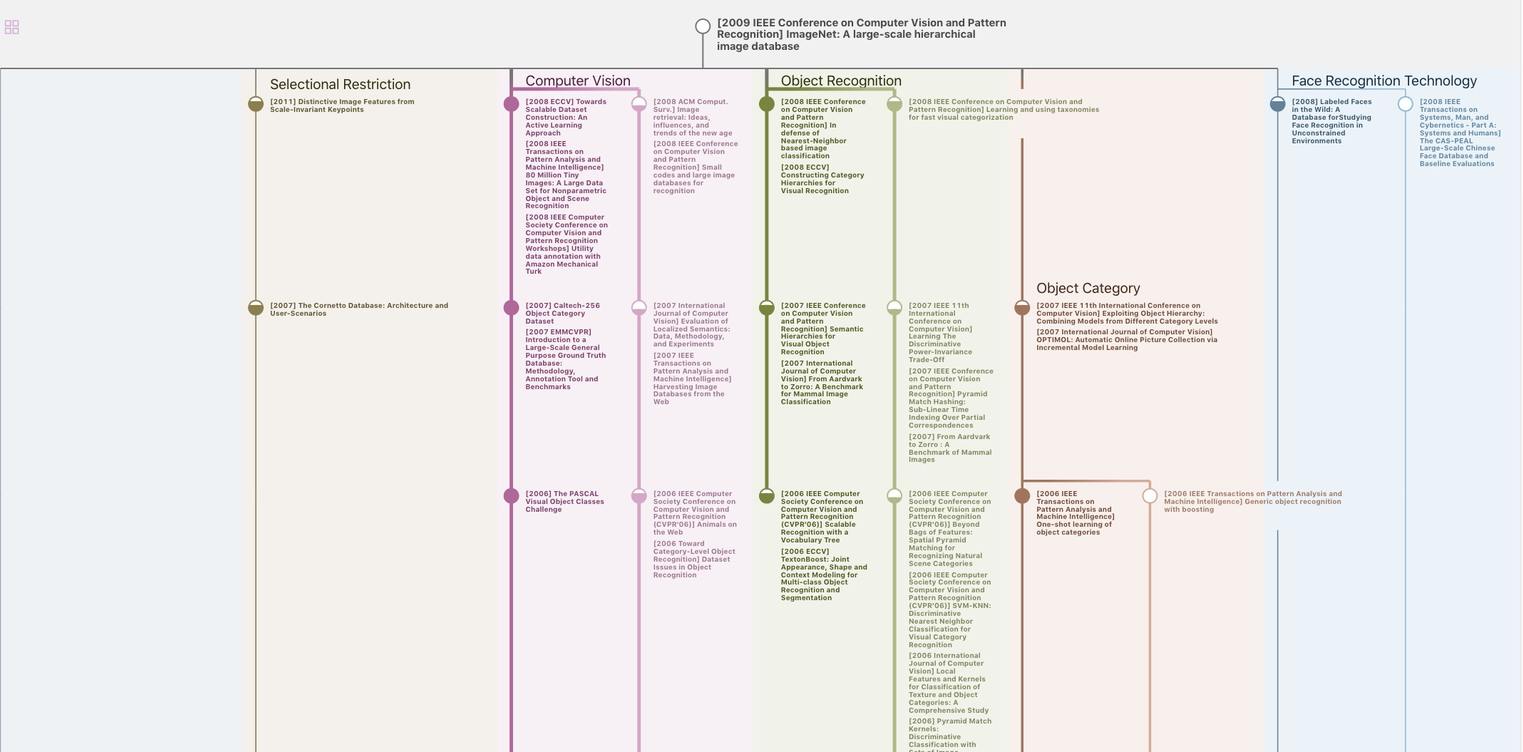
生成溯源树,研究论文发展脉络
Chat Paper
正在生成论文摘要