Modular Generative Adversarial Networks
European Conference on Computer Vision(2018)
摘要
Existing methods for multi-domain image-to-image translation (or generation) attempt to directly map an input image (or a random vector) to an image in one of the output domains. However, most existing methods have limited scalability and robustness, since they require building independent models for each pair of domains in question. This leads to two significant shortcomings: (1) the need to train exponential number of pairwise models, and (2) the inability to leverage data from other domains when training a particular pairwise mapping. Inspired by recent work on module networks, this paper proposes ModularGAN for multi-domain image generation and image-to-image translation. ModularGAN consists of several reusable and composable modules that carry on different functions (e.g., encoding, decoding, transformations). These modules can be trained simultaneously, leveraging data from all domains, and then combined to construct specific GAN networks at test time, according to the specific image translation task. This leads to ModularGAN's superior flexibility of generating (or translating to) an image in any desired domain. Experimental results demonstrate that our model not only presents compelling perceptual results but also outperforms state-of-the-art methods on multi-domain facial attribute transfer.
更多查看译文
关键词
Neural modular network,Generative adversarial network,Image generation,Image translation
AI 理解论文
溯源树
样例
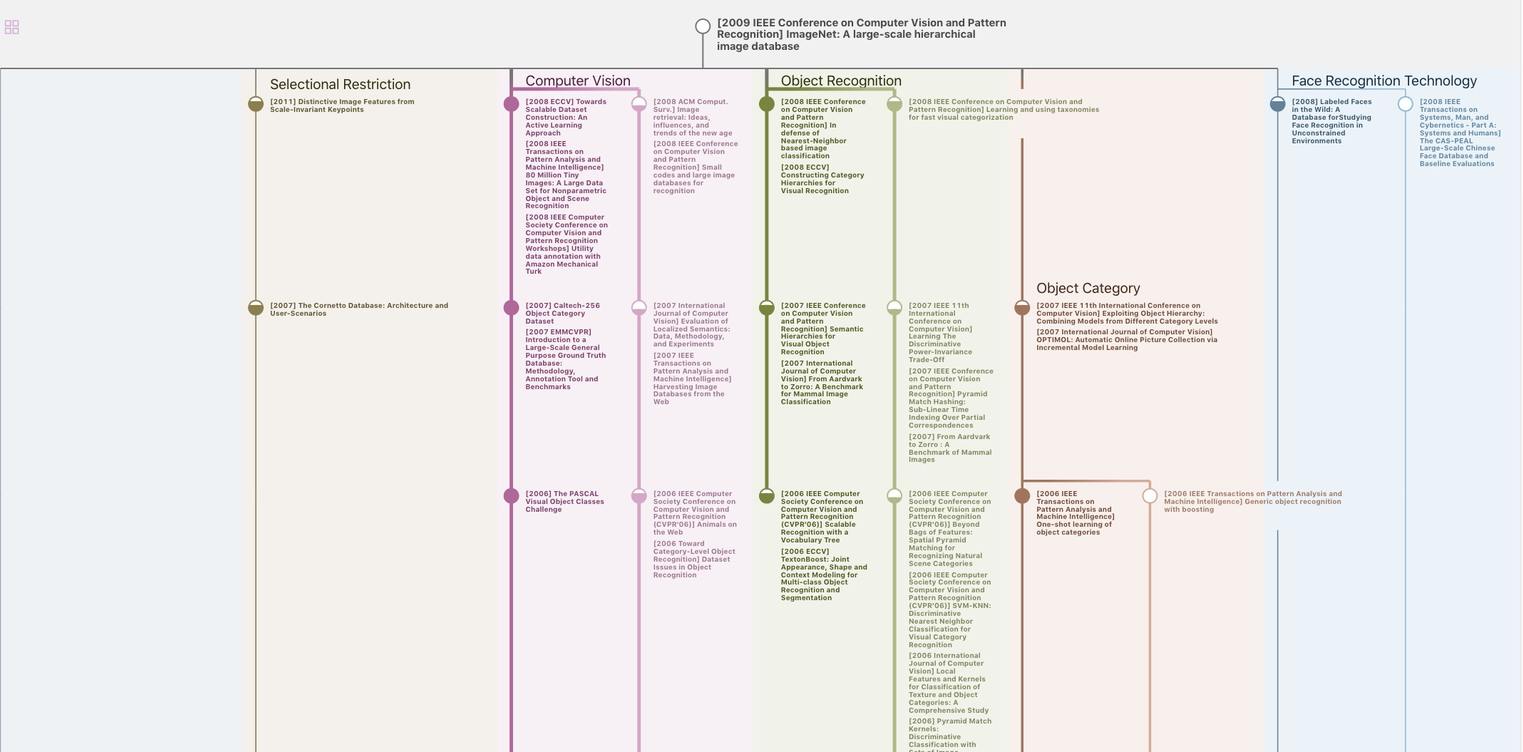
生成溯源树,研究论文发展脉络
Chat Paper
正在生成论文摘要