Transductive Centroid Projection For Semi-Supervised Large-Scale Recognition
COMPUTER VISION - ECCV 2018, PT V(2018)
摘要
Conventional deep semi-supervised learning methods, such as recursive clustering and training process, suffer from cumulative error and high computational complexity when collaborating with Convolutional Neural Networks. To this end, we design a simple but effective learning mechanism that merely substitutes the last fully-connected layer with the proposed Transductive Centroid Projection (TCP) module. It is inspired by the observation of the weights in the final classification layer (called anchors) converge to the central direction of each class in hyperspace. Specifically, we design the TCP module by dynamically adding an ad hoc anchor for each cluster in one mini-batch. It essentially reduces the probability of the inter-class conflict and enables the unlabelled data functioning as labelled data. We inspect its effectiveness with elaborate ablation study on seven public face/person classification benchmarks. Without any bells and whistles, TCP can achieve significant performance gains over most state-of-the-art methods in both fully-supervised and semi-supervised manners.
更多查看译文
关键词
Person Re-ID, Face recognition, Deep semi-supervised learning
AI 理解论文
溯源树
样例
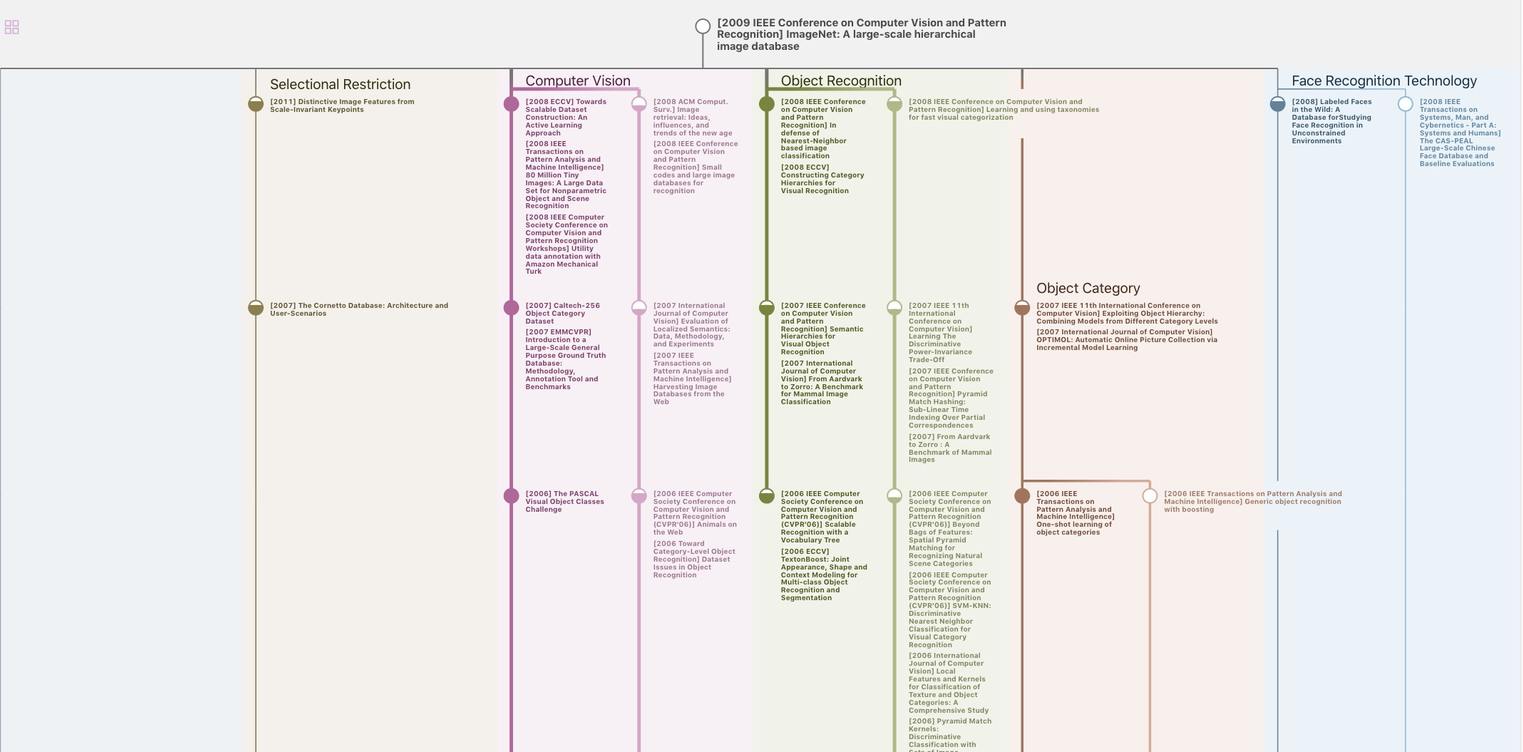
生成溯源树,研究论文发展脉络
Chat Paper
正在生成论文摘要