Leveraging the Power of Informative Users for Local Event Detection.
ASONAM '18: International Conference on Advances in Social Networks Analysis and Mining Barcelona Spain August, 2018(2018)
摘要
Detecting local events (e.g., protests, accidents) in real-time is an important task needed by a wide spectrum of real-world applications. In recent years, with the proliferation of social media platforms, we can access massive geo-tagged social messages, which can serve as a precious resource for timely local event detection. However, existing local event detection methods either suffer from unsatisfactory performances or need intensive annotations. These limitations make existing methods impractical for large-scale applications. Through the analysis of real-world datasets, we found that the informativeness level of social media users, which is neglected by existing work, plays a highly critical role in distilling event-related information from noisy social media contexts. Motivated by this finding, we propose an unsupervised framework, named LEDetect, to estimate the informativeness level of social media users and leverage the power of highly informative users for local event detection. Experiments on a large-scale real-world dataset show that the proposed LEDetect model can improve the performance of event detection compared with the state-of-the-art unsupervised approach. Also, we use case studies to show that the events discovered by the proposed model are of high quality and the extracted highly informative users are reasonable.
更多查看译文
关键词
local events,real-world applications,social media platforms,social messages,local event detection methods,real-world dataset,informativeness level,social media users,event-related information,noisy social media contexts,informative users
AI 理解论文
溯源树
样例
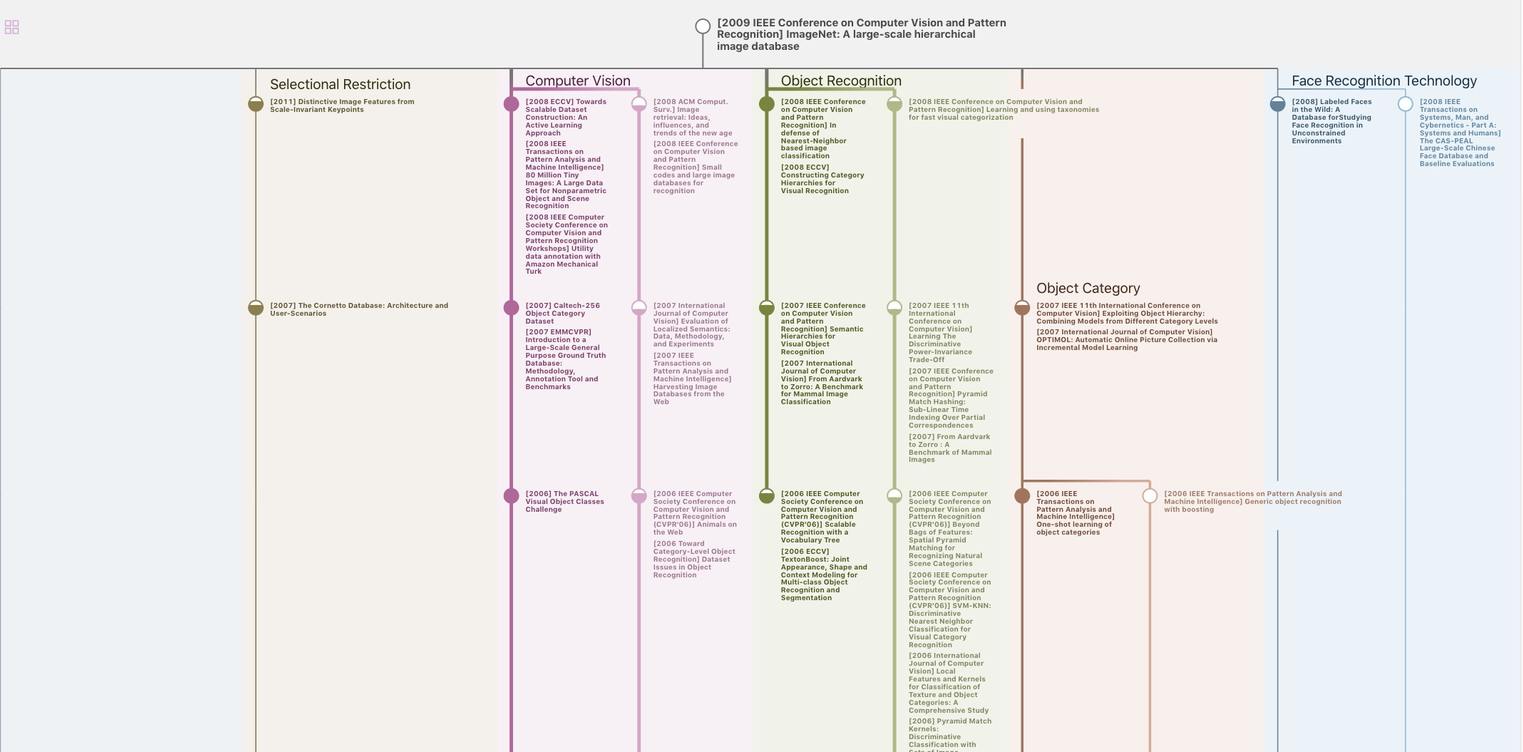
生成溯源树,研究论文发展脉络
Chat Paper
正在生成论文摘要