Predicting trajectories of vehicles using large-scale motion priors
2018 IEEE INTELLIGENT VEHICLES SYMPOSIUM (IV)(2018)
摘要
We present a simple yet effective paradigm to accurately predict the future trajectories of observed vehicles in dense city environments. We equipped a large fleet of cars with cameras and performed city-scale structure-from-motion to accurately reconstruct 10M positions of their trajectories spanning over 1000h of driving. We demonstrate that this information can be used as a powerful high-fidelity prior to predict future trajectories of newly observed vehicles in the area without the need for any knowledge of road infrastructure or vehicle motion models. By relating the current position of the observed car to a large dataset of the previously exhibited motion in the area we can directly perform prediction of its future position. We evaluate our method on two large-scale data sets from San Francisco and New York City and demonstrate an order of magnitude improvement compared to a linear-motion based method. We also demonstrate that the performance naturally improves with the amount of data and ultimately yields a system that can accurately predict vehicle motion in challenging situations across extremes in traffic, time, and weather conditions.
更多查看译文
关键词
city-scale structure-from-motion,linear-motion based method,New York City,large-scale data sets,observed car,powerful high-fidelity,dense city environments,large-scale motion priors,vehicle motion
AI 理解论文
溯源树
样例
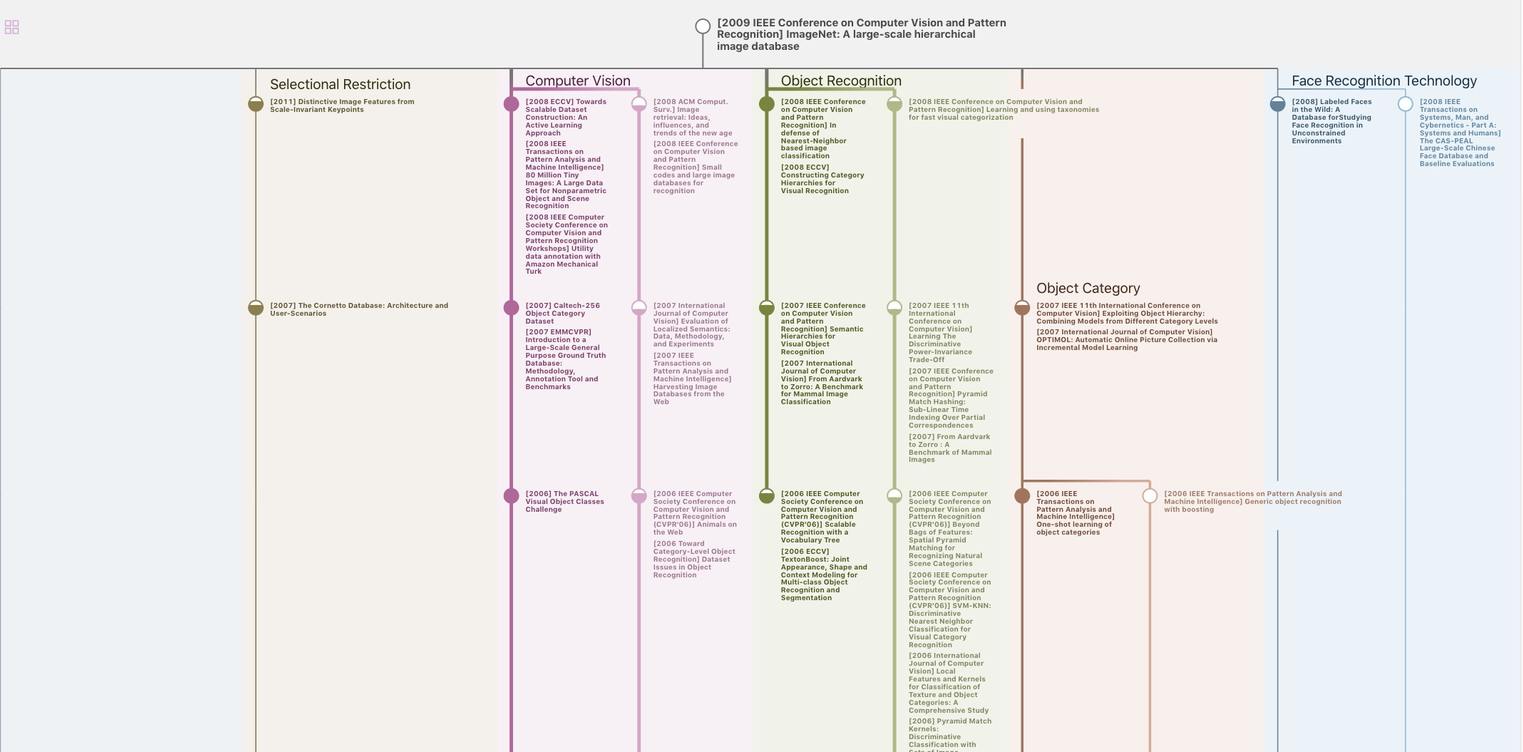
生成溯源树,研究论文发展脉络
Chat Paper
正在生成论文摘要