Augmenting Image Classifiers Using Data Augmentation Generative Adversarial Networks
ARTIFICIAL NEURAL NETWORKS AND MACHINE LEARNING - ICANN 2018, PT III(2018)
摘要
Effective training of neural networks requires much data. In the low-data regime, parameters are underdetermined, and learnt networks generalise poorly. Data Augmentation alleviates this by using existing data more effectively, but standard data augmentation produces only limited plausible alternative data. Given the potential to generate a much broader set of augmentations, we design and train a generative model to do data augmentation. The model, based on image conditional Generative Adversarial Networks, uses data from a source domain and learns to take a data item and augment it by generating other within-class data items. As this generative process does not depend on the classes themselves, it can be applied to novel unseen classes. We demonstrate that a Data Augmentation Generative Adversarial Network (DAGAN) augments classifiers well on Omniglot, EMNIST and VGGFace.
更多查看译文
关键词
Generative Adversarial Networks (GAN), Data Augmentation, Unseen Classes, Source Domain, Conditional GANs
AI 理解论文
溯源树
样例
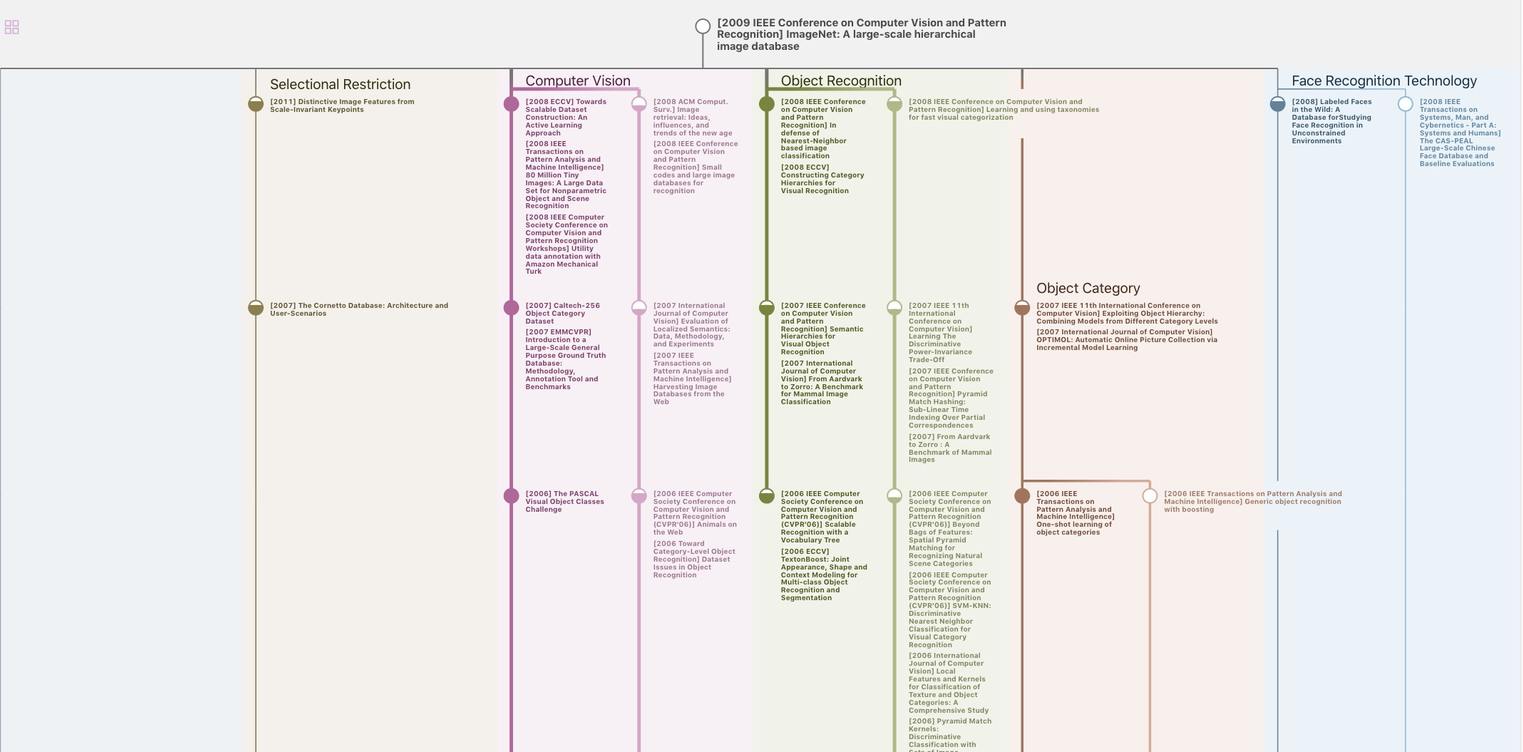
生成溯源树,研究论文发展脉络
Chat Paper
正在生成论文摘要