Real-Time Fpga-Based Anomaly Detection For Radio Frequency Signals
2018 IEEE INTERNATIONAL SYMPOSIUM ON CIRCUITS AND SYSTEMS (ISCAS)(2018)
摘要
We describe an open source, FPGA accelerated neural network-based anomaly detector. The detector derives its training set from observed exemplar data and continuous learning in software can be undertaken in an unsupervised manner. Trained network weights are passed to the FPGA, which performs continuous high-speed anomaly detection, combining parallelism reduced precision, and a single-chip design to maximise performance and energy efficiency. Our design can process continuous 200 MS/s complex inputs, producing anomaly classifications at the same rate, with a latency of 105 ns, an improvement of at least 4 orders of magnitude over a software radio such as GNU Radio.
更多查看译文
关键词
continuous learning,trained network weights,continuous high-speed anomaly detection,parallelism reduced precision,single-chip design,energy efficiency,anomaly classifications,software radio,GNU Radio,Radio frequency signals,open source,observed exemplar data,real-time FPGA-based anomaly detection,FPGA accelerated neural network-based anomaly detector,time 105.0 ns
AI 理解论文
溯源树
样例
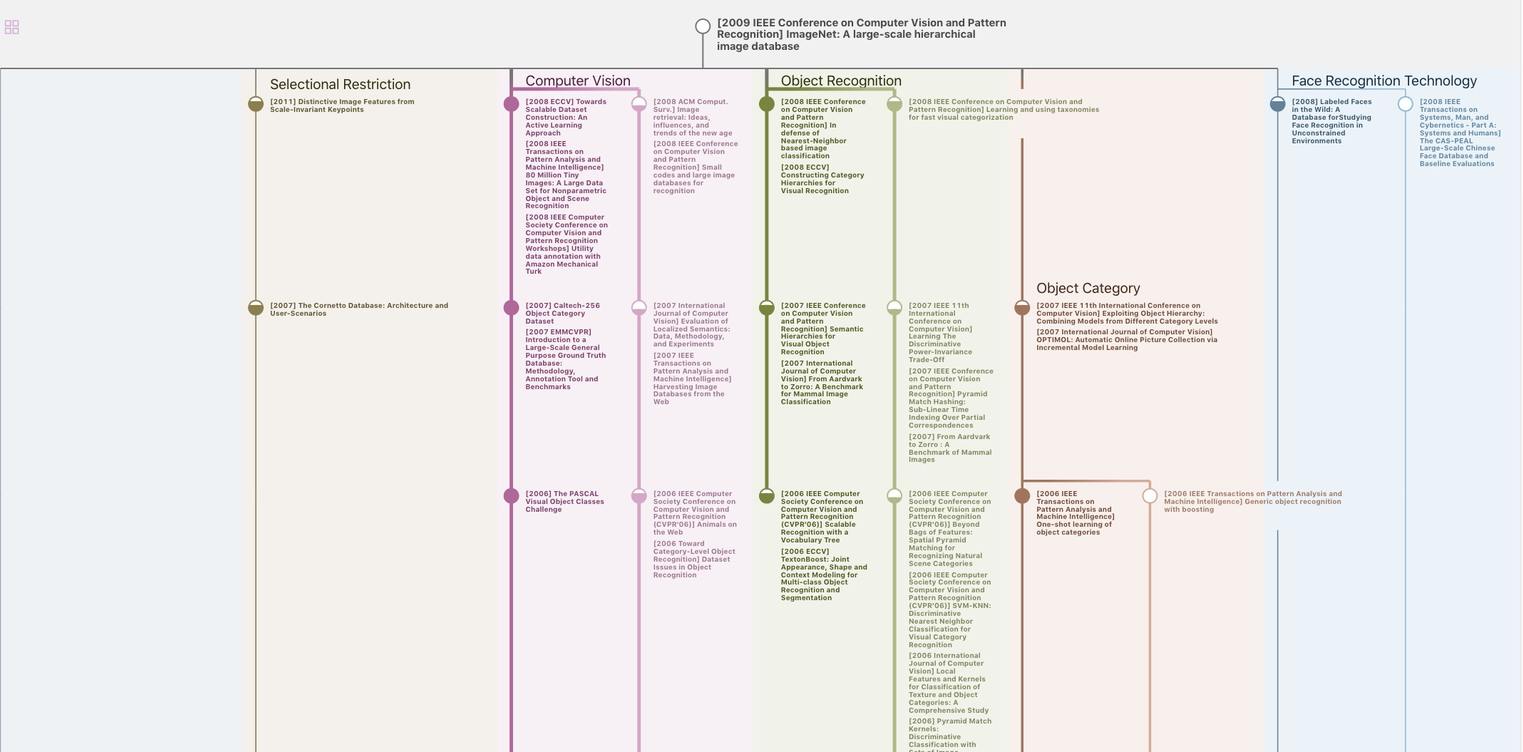
生成溯源树,研究论文发展脉络
Chat Paper
正在生成论文摘要