Energy-Efficient Deep In-Memory Architecture For Nand Flash Memories
2018 IEEE INTERNATIONAL SYMPOSIUM ON CIRCUITS AND SYSTEMS (ISCAS)(2018)
摘要
This paper proposes an energy-efficient deep inmemory architecture for NAND flash (DIMA-F) to perform machine learning and inference algorithms on NAND flash memory. Algorithms for data analytics, inference, and decision-making require processing of large data volumes and are hence limited by data access costs. DIMA-F achieves energy savings and throughput improvement for such algorithms by reading and processing data in the analog domain at the periphery of NAND flash memory. This paper also provides behavioral models of DIMA-F that can be used for analysis and large scale system simulations in presence of circuit non-idealities and variations. DIMA-F is studied in the context of linear support vector machines and k-nearest neighbor for face detection and recognition, respectively. An estimated 8 x -to-23 x reduction in energy and 9 x -to-15 x improvement in throughput resulting in EDP gains up to 345x over the conventional NAND flash architecture incorporating an external digital ASIC for computation.
更多查看译文
关键词
Energy-Efficient Deep In-memory Architecture,NAND flash memory,DIMA-F,data volumes,data access costs,energy savings,conventional NAND flash architecture incorporating,inference algoriths,machine learning,linear support vector machines,k-nearest neighbor,face detection,face recognition,external digital ASIC
AI 理解论文
溯源树
样例
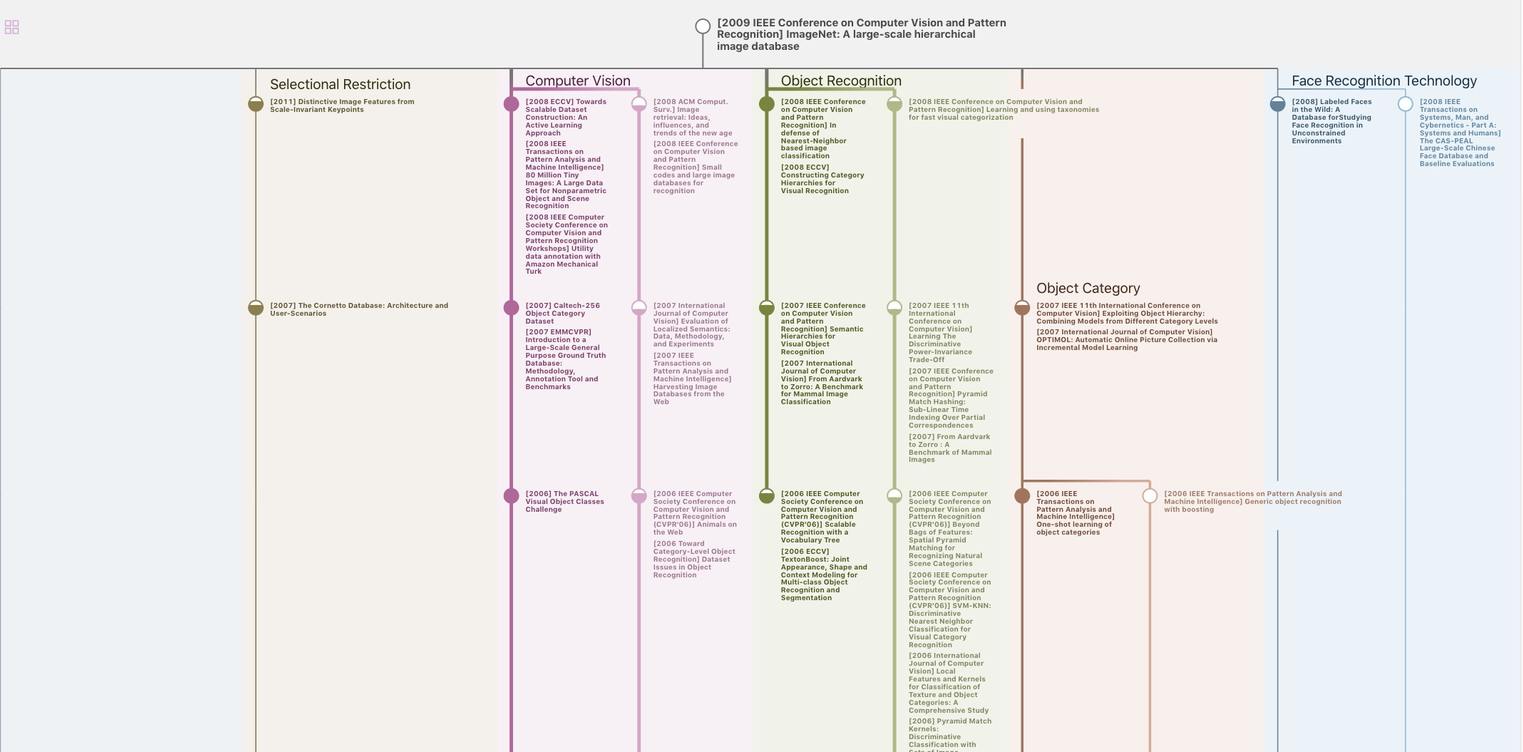
生成溯源树,研究论文发展脉络
Chat Paper
正在生成论文摘要