Guided Genetic Algorithm for Information Diffusion Problems
2018 IEEE CONGRESS ON EVOLUTIONARY COMPUTATION (CEC)(2018)
摘要
Information diffusion is a process that involves the propagation of an arbitrary signal (message) in an environment. In the area of social networks, it is often associated with influence maximization. Influence maximization consists in the search for an optimum set of k network nodes (seed sets) that trigger the activation of a maximum total number of remaining network nodes according to a chosen propagation model. It is an attractive research topic due to its well-known difficulty and many practical applications. Influence maximization can be used in various areas spanning from social network analysis and data mining to practical applications such as viral marketing and opinion making. Formally, it can be formulated as a subset selection problem. Because of the proven hardness of the influence maximization problem, many metaheuristic and evolutionary methods have been proposed to tackle it. This paper presents and evaluates a new genetic algorithm for influence maximization. It is based on a recent genetic algorithm for fixed-length subset selection and takes advantage of the knowledge of the environment. The evolutionary algorithm is in this approach executed with respect to network properties and the probability that vertices with chosen properties are selected is increased. The experiments show that this approach improves the results of the evolutionary procedure and leads to the discovery of better seed sets.
更多查看译文
关键词
information diffusion, influence maximization, network science, genetic algorithms
AI 理解论文
溯源树
样例
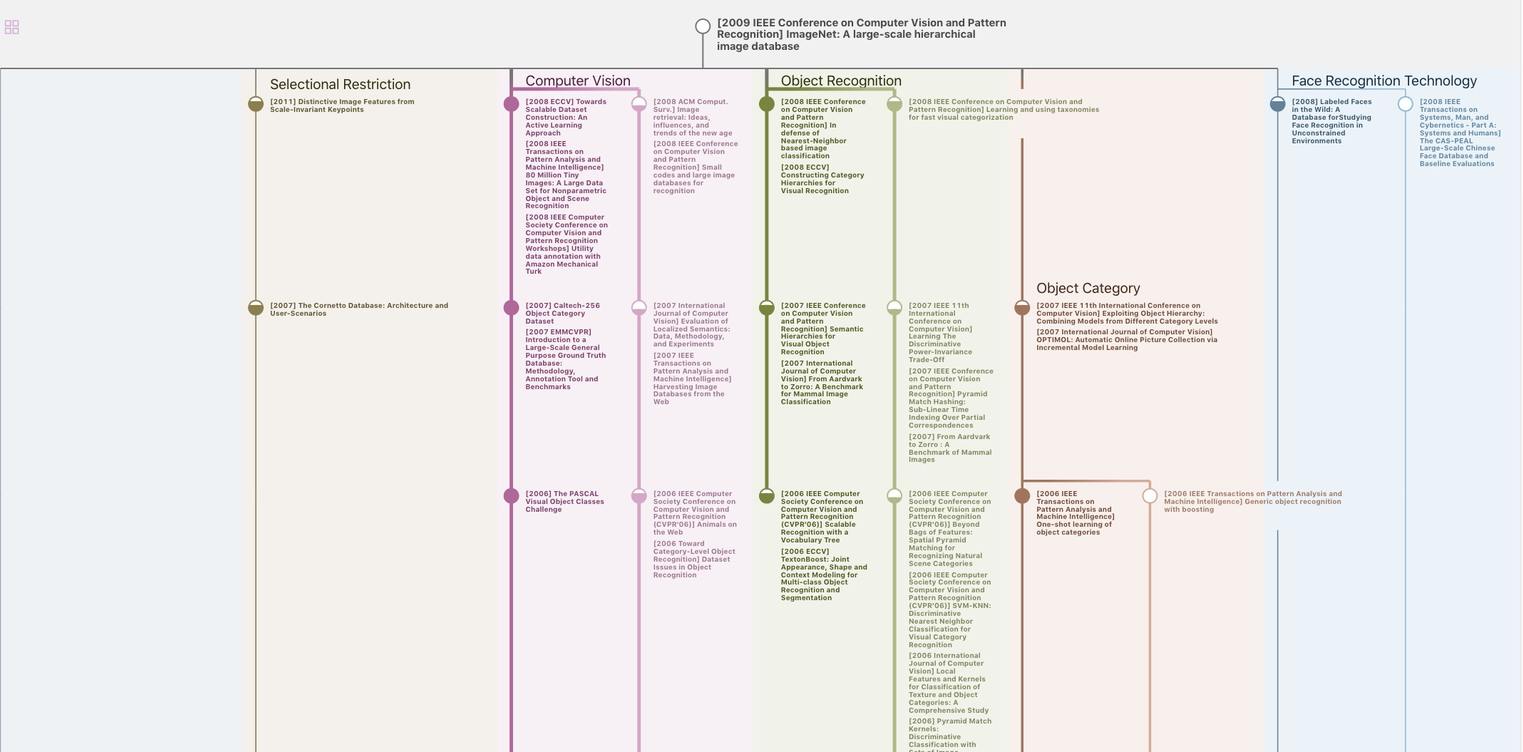
生成溯源树,研究论文发展脉络
Chat Paper
正在生成论文摘要