Confidence Measures for Carbon-Nanotube / Liquid Crystals Classifiers
2018 IEEE CONGRESS ON EVOLUTIONARY COMPUTATION (CEC)(2018)
摘要
This paper focuses on a performance analysis of single-walled-carbon-nanotube / liquid crystal classifiers produced by evolution in materio. A new confidence measure is proposed in this paper. It is different from statistical tools commonly used to evaluate the performance of classifiers in that it is based on physical quantities extracted from the composite and related to its state. Using this measure, it is confirmed that in an untrained state, ie: before being subjected to an algorithm-controlled evolution, the carbon-nanotube-based composites classify data at random. The training, or evolution, process brings these composites into a state where the classification is no longer random. Instead, the classifiers generalise well to unseen data and the classification accuracy remains stable across tests. The confidence measure associated with the resulting classifier's accuracy is relatively high at the classes' boundaries, which is consistent with the problem formulation.
更多查看译文
关键词
performance analysis,confidence measure,untrained state,carbon-nanotube-based composites,single-walled-carbon-nanotube/liquid crystal classifiers,data classification,evolution in materio,EiM,evolutionary algorithms
AI 理解论文
溯源树
样例
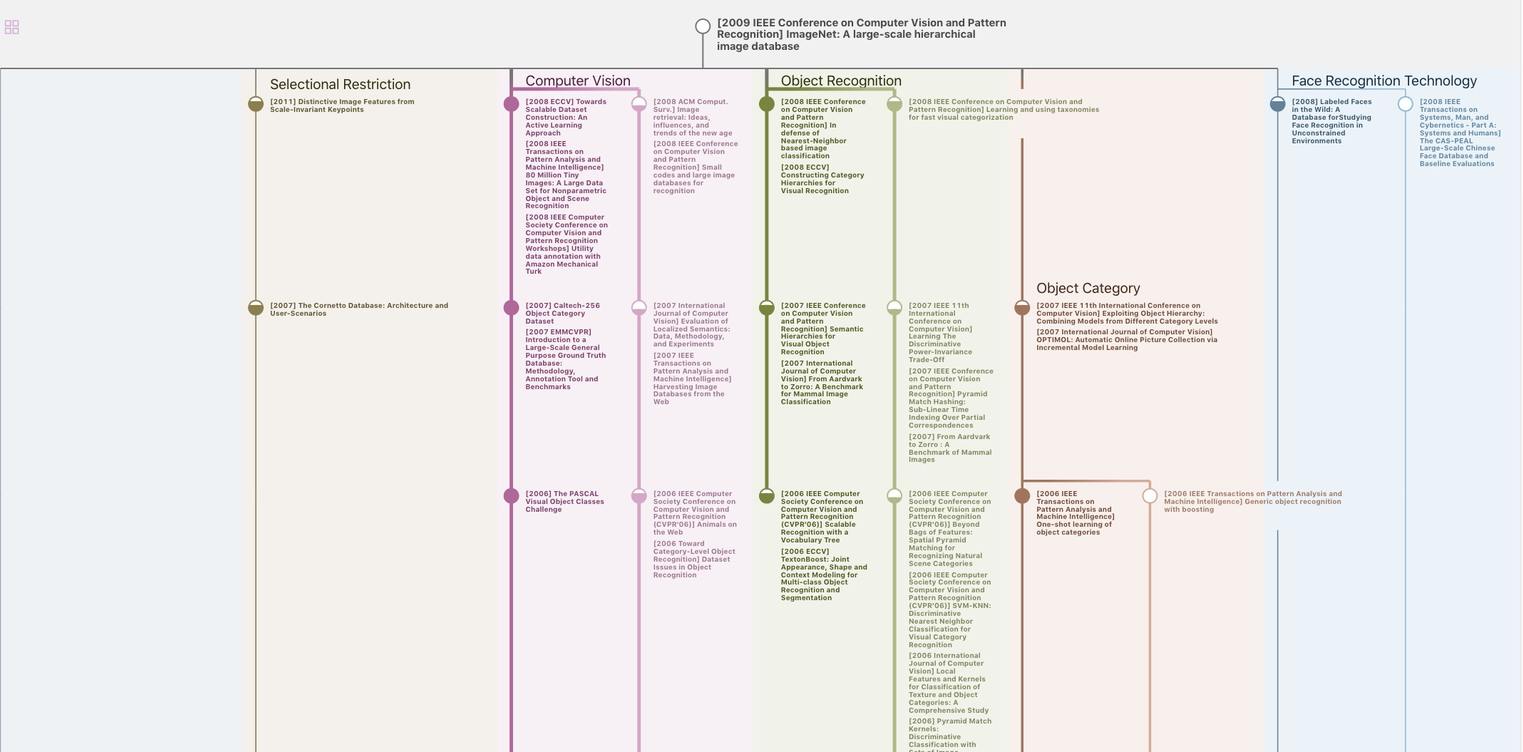
生成溯源树,研究论文发展脉络
Chat Paper
正在生成论文摘要