Predicting Freezing Of Gait In Parkinsons Disease Patients Using Machine Learning
2018 IEEE CONGRESS ON EVOLUTIONARY COMPUTATION (CEC)(2018)
摘要
Freezing of gait (FoG) is one of the most alarming motor symptoms of Parkinson's disease, most commonly experienced by subjects who have suffered from the disease for a long period of time. Furthermore, freezing of gait can lead to falls and may result to nursing home admissions which can negatively affect the quality of life for patients, in addition to raising a broader set of socioeconomic consequences. In this research, machine learning algorithms are utilised as means of identifying the freezing of gait event prior to its onset. An accelerometer time series dataset containing 237 individual Freezing of Gait events over 8 patients was considered, from which features were extracted and presented to 7 machine learning classifiers. Our simulation results indicated that machine learning algorithms are powerful tools for the early prediction of freezing of gait, capable of obtaining high sensitivity and specificity for the identification of the onset of Freezing of Gait. Support Vector Machines with Polynomial kernel achieved the highest performance in comparison with the benchmarked techniques. The classification algorithm was applied to 5 second windows using 18 features, obtaining balanced accuracies (the mean value of sensitivity and specificity) of 91%, 90%, and 82% over the Walk, FoG and Transition classes, respectively.
更多查看译文
关键词
Freezing of gait, Parkinson disease, signal analysis, prediction, accelerometer, feature extraction, feature selection, machine learning
AI 理解论文
溯源树
样例
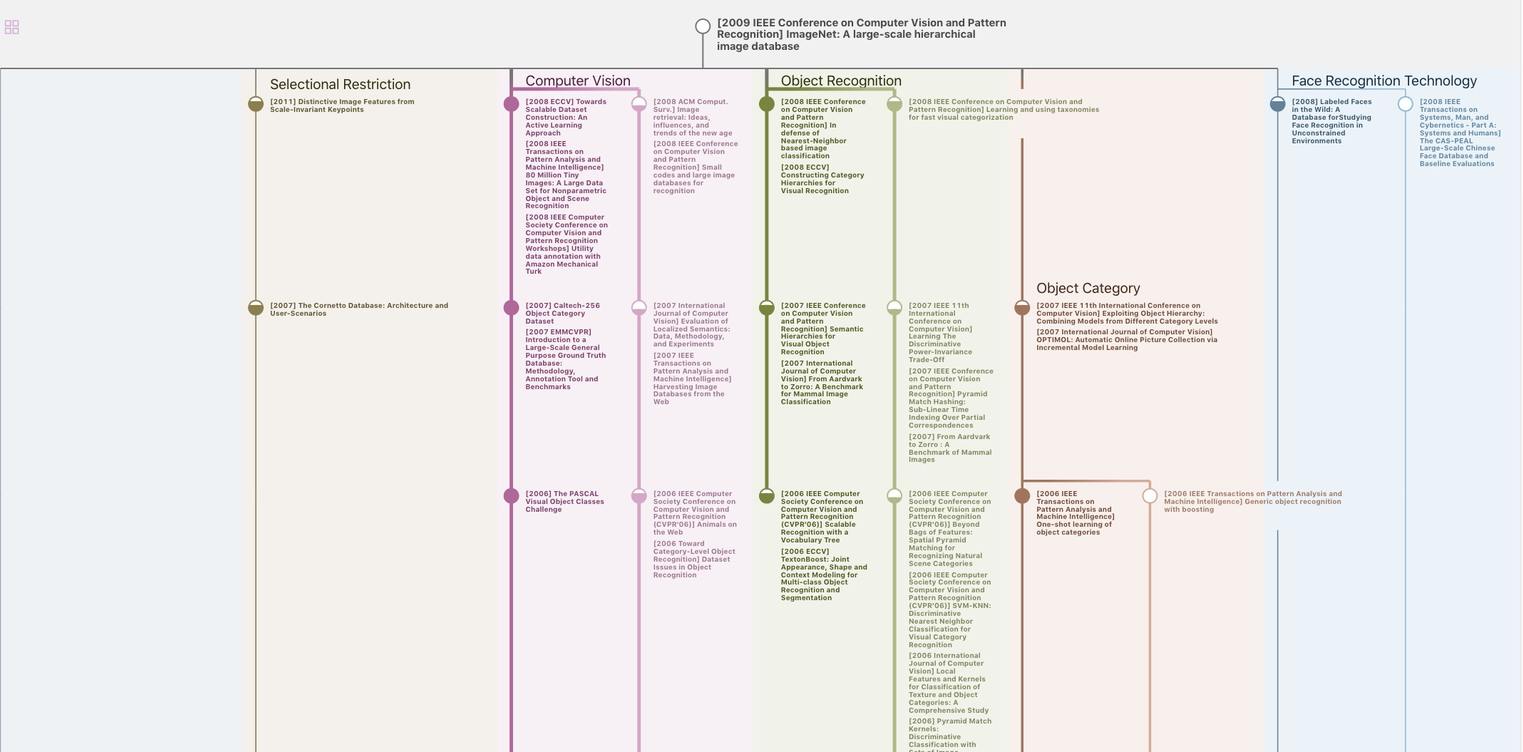
生成溯源树,研究论文发展脉络
Chat Paper
正在生成论文摘要