Accelerating Protein Structure Prediction Using Active Learning and Surrogate-Based Optimization
2018 IEEE Congress on Evolutionary Computation (CEC)(2018)
摘要
The surrogate models are offered as effective tools to approximate computationally expensive objective functions. This study investigates how approximation strategy of such models can be used for high dimensional protein structure prediction (PSP) problems. Two major contributions of the proposed approach are: 1) employing Stochastic Response Surface (SRS) to bias the initial population toward promising areas and 2) using queries of an active learning algorithm and a surrogate model to replace in part the original computationally expensive solver. The introduced framework is applied on several extensions of the differential evolution (DE) algorithm which are among noteworthy approaches for the PSP. Numerical experiments indicate that the proposed schema is able to improve performance of the conventional algorithms for the PSP problems in both terms of convergence speed and accuracy.
更多查看译文
关键词
evolutionary computation,surrogate-assisted computation,differential evolution,protein structure prediction,active learning
AI 理解论文
溯源树
样例
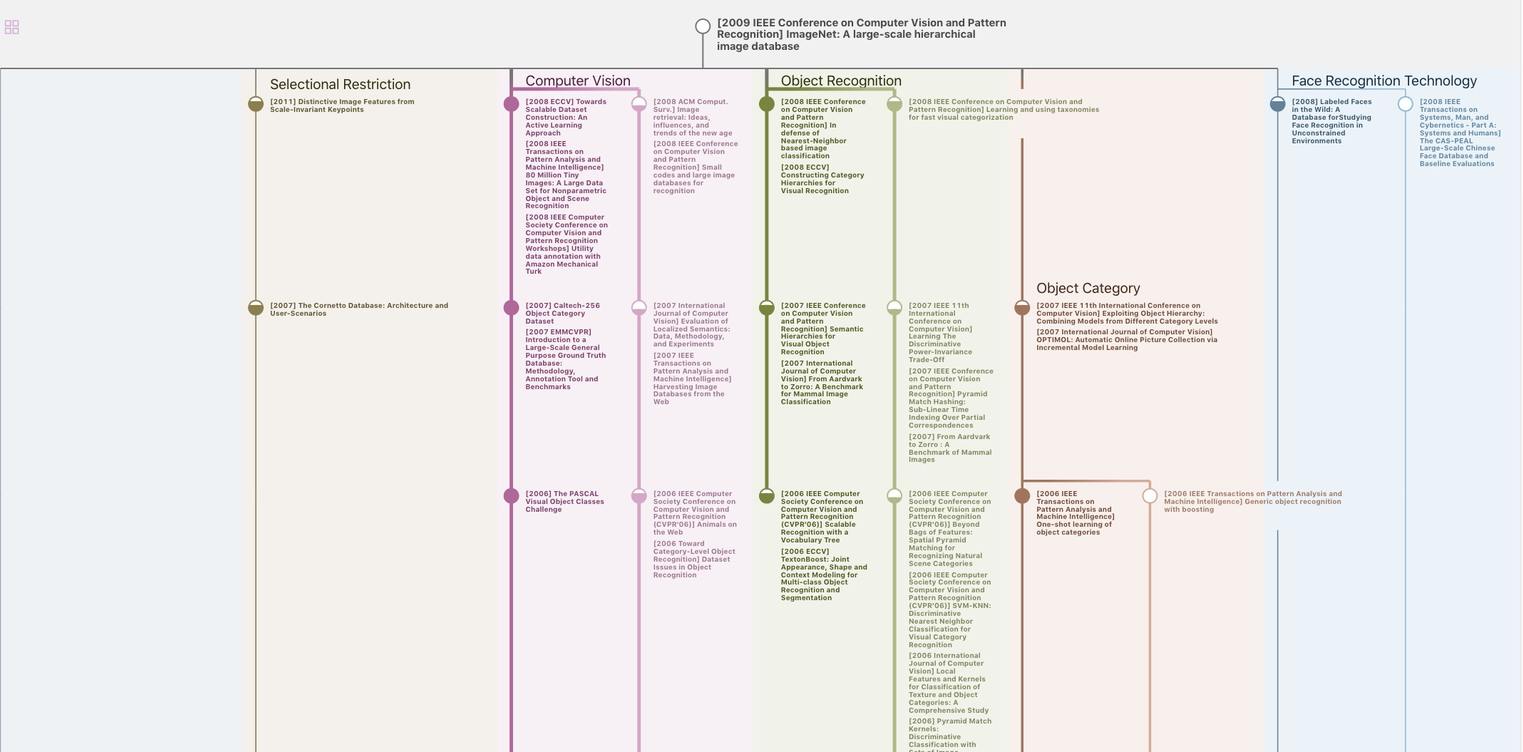
生成溯源树,研究论文发展脉络
Chat Paper
正在生成论文摘要