Context Aware Telco Churn Prediction Powered By Temporal Feature Engineering
2018 IEEE INTERNATIONAL CONFERENCE ON PERVASIVE COMPUTING AND COMMUNICATIONS WORKSHOPS (PERCOM WORKSHOPS)(2018)
摘要
It is still challenging in telecommunication (Telco) industry to precisely predict those who will churn from the current Telco operator to another one in next month. State of art approaches suffer from the issue that the predicted churners might be those silent customers with trivial profits to Telco operators. Thus, it is quite necessary to focus on the churn prediction problem with respect to active customers, instead of silent ones. Thus, we propose 3 kinds of new features in order to capture the context-aware customer behaviour in terms of temporal features. Such features include long-range Feature, trend feature and regression-based feature for Telco churn prediction. After that, we employ an ensemble process on the base learners: Random Forst (RF), XGB and GBDT +SVM. Experimental results confirm that the prediction performance has been improved by using these new features. From millions of active customers, this system can provide a list of prepaid customers who are most likely to churn in the next month, having 0.69 precision for the top 25000 predicted churners in the list. At the same time, achieve 44.69% improvement when given 5 x10(4).
更多查看译文
关键词
temporal Feature engineering,telecommunication industry,Telco operators,context-aware customer behaviour,long-range Feature,trend feature,regression-based feature,context aware Telco churn prediction,Telco operator,ensemble process,Random Forst,XGB,GBDT + SVM
AI 理解论文
溯源树
样例
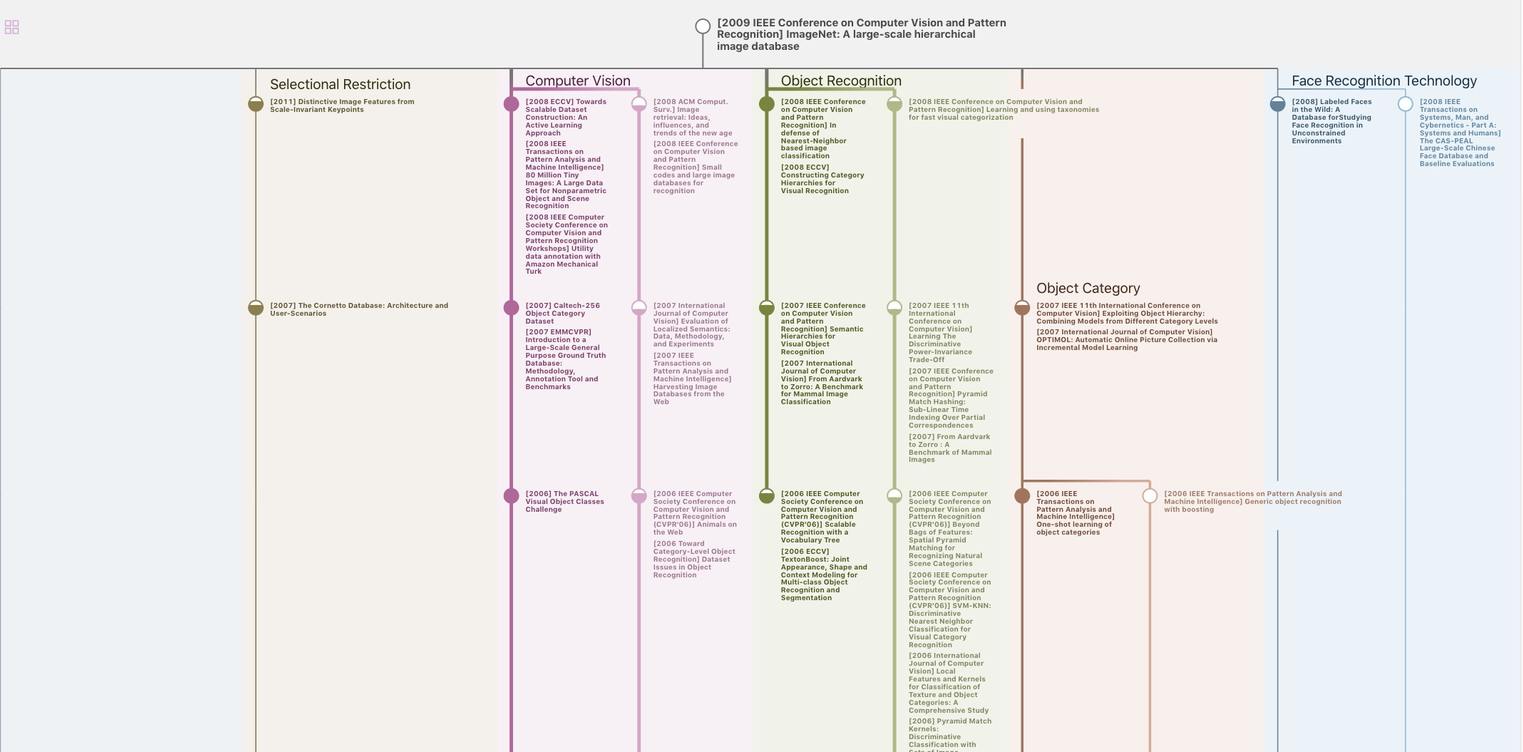
生成溯源树,研究论文发展脉络
Chat Paper
正在生成论文摘要