Support Vector Metric Learning on Symmetric Positive Definite Manifold
2018 IEEE International Conference on Multimedia and Expo (ICME)(2018)
摘要
The manifold of symmetric positive definite (SPD) matrices has drawn significant attention because of its widespread applications. SPD matrices provide compact nonlinear representations of data and form a special type of Riemannian manifold. The direct application of support vector machines on SPD manifold maybe fails due to lack of samples per class. In this paper, we propose a support vector metric learning (SVML) model on SPD manifold. We define a positive definite kernel for point pairs on SPD manifold and transform metric learning on SPD manifold to a point pair classification problem. The metric learning problem can be efficiently solved by standard support vector machines. Compared with classifying points on SPD manifold by support vector machines directly, SVML effectively learns a distance metric for SPD matrices by training a binary support vector machine model. Experiments on video based face recognition, image set classification, and material classification show that SVML outperforms the state-of-the-art metric learning algorithms on SPD manifold.
更多查看译文
关键词
Riemannian manifold,Support vector,Metric learning
AI 理解论文
溯源树
样例
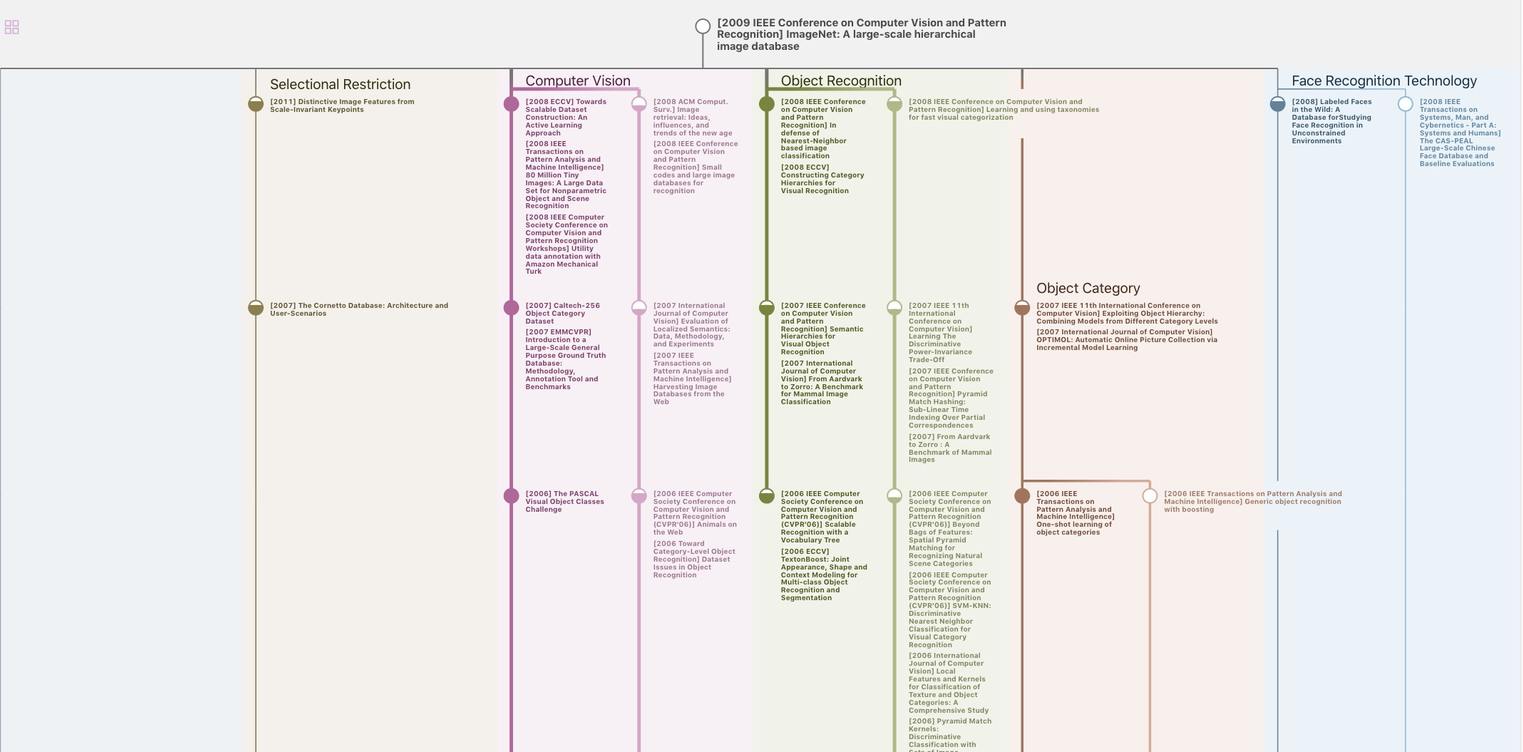
生成溯源树,研究论文发展脉络
Chat Paper
正在生成论文摘要