Ensemble of Label Specific Features for Multi-Label Classification
2018 IEEE International Conference on Multimedia and Expo (ICME)(2018)
摘要
In this paper, we focus on multi-label classification which associates one instance with multiple labels. The approach Label-specIfic FeaTures (LIFT) achieves state-of-the-art performance due to the label-specific features. However, the main limitation of LIFT is the poor local optima in k-means used at training stage. For this issue, in this paper, we propose to mitigate the limitation for high classification accuracy with ensemble way and term our approach as Ensemble of Label specIfic FeaTures (ELIFT). Specifically, our approach firstly constructs multiple LIFT classifiers by using multiple training sets generated by bagging strategy. Furthermore, different classifiers are weighted automatically according to the loss of each classifier. Finally, for each new instance, the predicted label vector is obtained by the weighted ensemble classifiers learned. Experiments conducted on five benchmark datasets demonstrate the performance of the proposed method outperforms the state-of-the-art approaches.
更多查看译文
关键词
multi-label classification,ensemble,LIFT
AI 理解论文
溯源树
样例
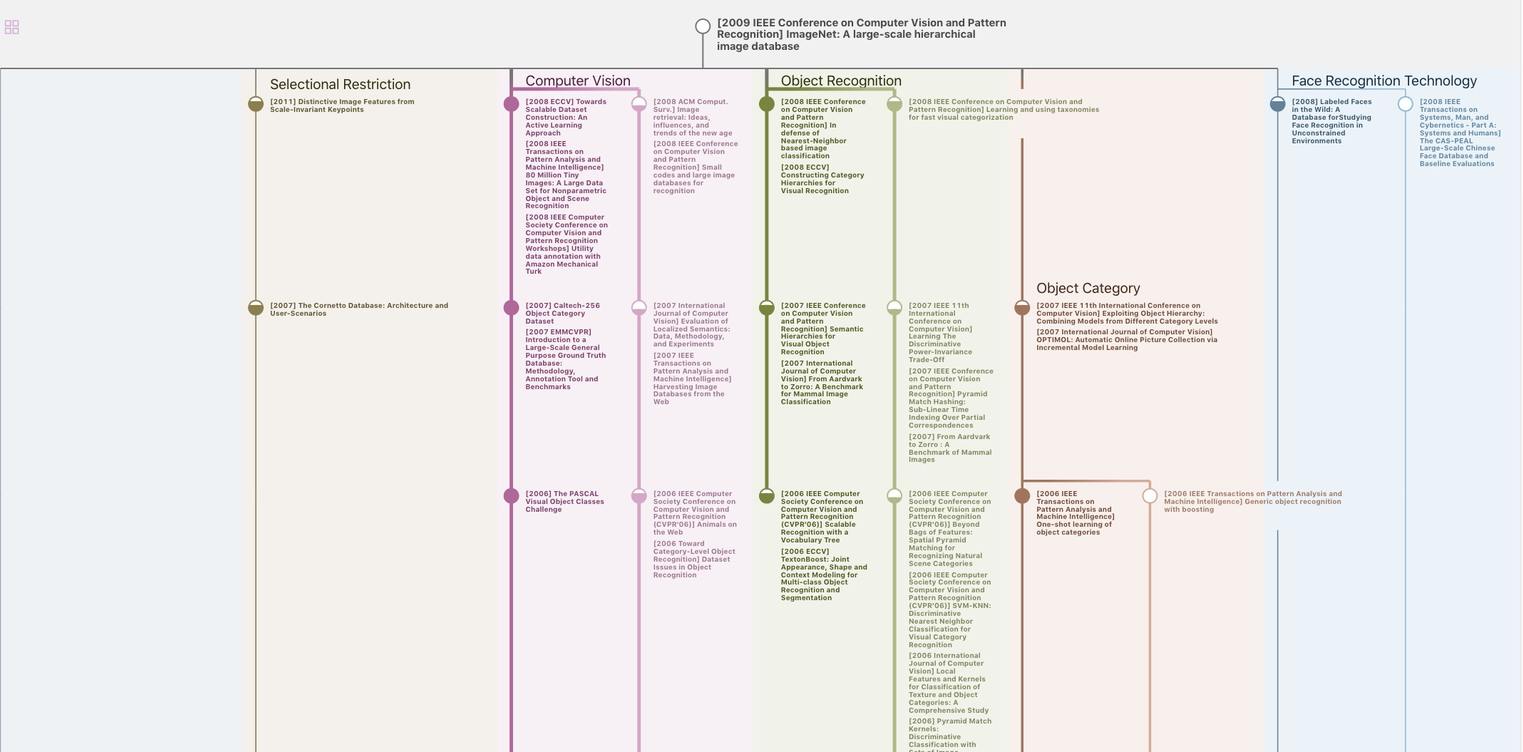
生成溯源树,研究论文发展脉络
Chat Paper
正在生成论文摘要