Feature Re-Learning with Data Augmentation for Content-based Video Recommendation.
MM '18: ACM Multimedia Conference Seoul Republic of Korea October, 2018(2018)
摘要
This paper describes our solution for the Hulu Content-based Video Relevance Prediction Challenge. Noting the deficiency of the original features, we propose feature re-learning to improve video relevance prediction. To generate more training instances for supervised learning, we develop two data augmentation strategies, one for frame-level features and the other for video-level features. In addition, late fusion of multiple models is employed to further boost the performance. Evaluation conducted by the organizers shows that our best run outperforms the Hulu baseline, obtaining relative improvements of 26.2% and 30.2% on the TV-shows track and the Movies track, respectively, in terms of [email protected] The results clearly justify the effectiveness of the proposed solution.
更多查看译文
关键词
Content based video recommendation, Feature re-learning, Data augmentation
AI 理解论文
溯源树
样例
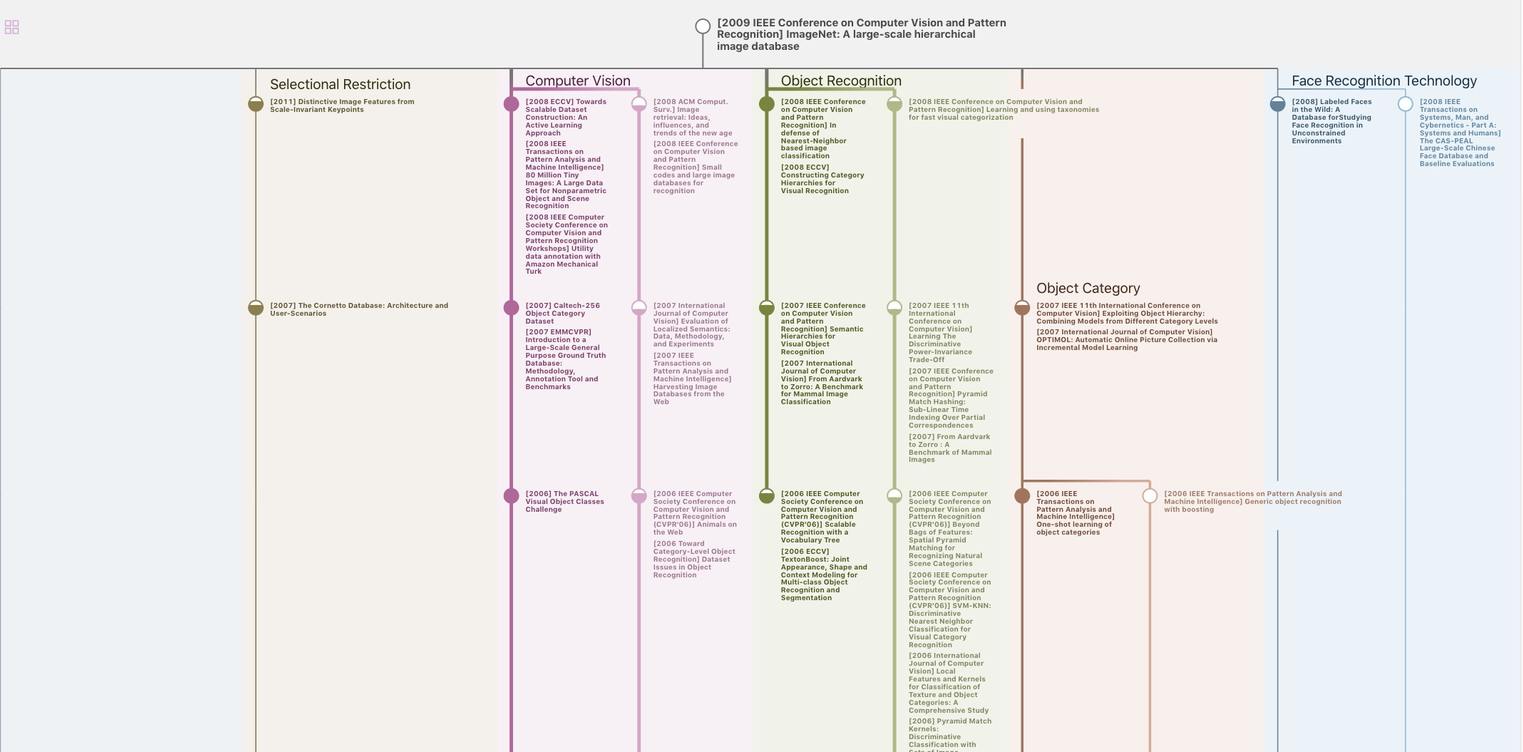
生成溯源树,研究论文发展脉络
Chat Paper
正在生成论文摘要