Possibilistic Clustering With Seeds
2018 IEEE INTERNATIONAL CONFERENCE ON FUZZY SYSTEMS (FUZZ-IEEE)(2018)
摘要
Clustering methods assign objects to clusters using only as prior information the characteristics of the objects. However, clustering algorithms performance can be improved when background knowledge is available. Such background knowledge can be incorporated in a clustering method as label constraints which results in a semi-supervised clustering algorithm. We propose to extend two possibilistic clustering algorithms to make use of available a priori information. The goal is twofold: to improve the accuracy of the clustering result by leading the method towards a desired solution and to detect outliers by taking advantage of the generated possibilistic partition. The proposed methods are called semi-supervised repulsive possibilistic c-means (SRPCM) and semi-supervised possibilistic fuzzy c-means (SPFCM). They correspond to possibilistic clustering algorithms that introduce label constraints. Experimental results show that the proposed algorithms using label constraints improve (1) the clustering result and (2) the outliers detection.
更多查看译文
关键词
clustering method,prior information,clustering algorithms performance,background knowledge,label constraints,possibilistic clustering algorithms,available a priori information,generated possibilistic partition,semisupervised repulsive clustering algorithm,semisupervised possibilistic fuzzy c-means
AI 理解论文
溯源树
样例
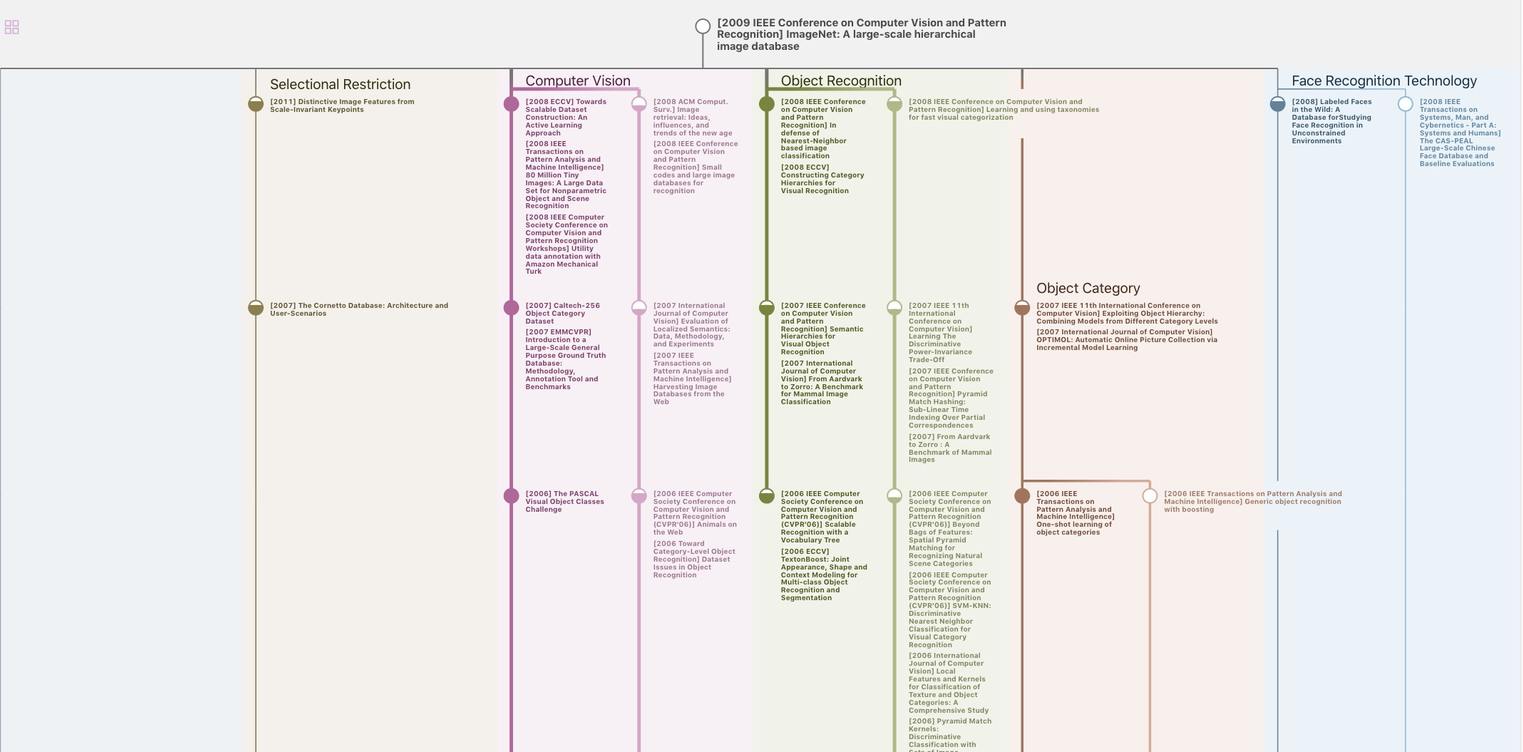
生成溯源树,研究论文发展脉络
Chat Paper
正在生成论文摘要