Beyond trans-dimensional RJMCMC with a case study in impulsive data modeling.
Signal Processing(2018)
摘要
Abstract Reversible jump Markov chain Monte Carlo (RJMCMC) is a Bayesian model estimation method, which has been generally used for trans-dimensional sampling and model order selection studies in the literature. In this study, we draw attention to unexplored potentials of RJMCMC beyond trans-dimensional sampling. the proposed usage, which we call trans-space RJMCMC exploits the original formulation to explore spaces of different classes or structures. This provides flexibility in using different types of candidate classes in the combined model space such as spaces of linear and nonlinear models or of various distribution families. As an application, we looked into a special case of trans-space sampling, namely trans-distributional RJMCMC in impulsive data modeling. In many areas such as seismology, radar, image, using Gaussian models is a common practice due to analytical ease. However, many noise processes do not follow a Gaussian character and generally exhibit events too impulsive to be successfully described by the Gaussian model. We test the proposed usage of RJMCMC to choose between various impulsive distribution families to model both synthetically generated noise processes and real-life measurements on power line communications impulsive noises and 2-D discrete wavelet transform coefficients.
更多查看译文
关键词
Reversible jump MCMC,Impulsive data modeling,PLC impulsive noise modeling,Wavelet coefficients modeling,Symmetric α-stable distribution,Generalized Gaussian distribution,Student’s t distribution
AI 理解论文
溯源树
样例
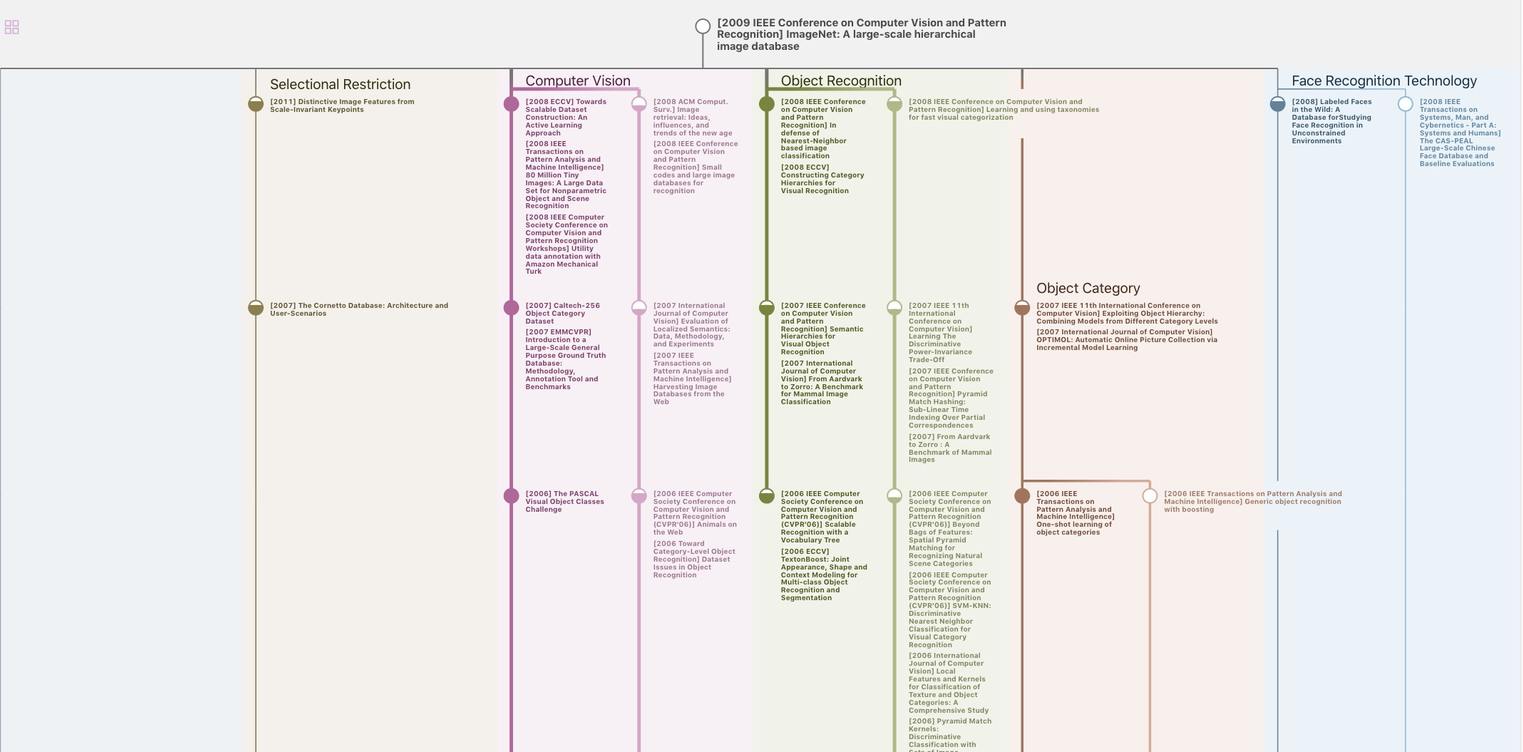
生成溯源树,研究论文发展脉络
Chat Paper
正在生成论文摘要