An Analysis of Policies from Stochastic Linear Quadratic Gaussian in Robotics Problems with State- and Control-Dependent Noise
Journal of Intelligent and Robotic Systems(2017)
摘要
Among all the mathematical frameworks used in the control and robotics communities to handle uncertainties, the stochastic variants of optimal control frameworks are appealing, in particular because of the existence of efficient tools to solve them computationally, such as dynamic programming. However, in many cases, because of their formulation as a classical optimization problem, it may be difficult to ponder the expected solutions for a given choice of the objective function to minimize. In this paper, we perform an in-depth analysis of the behavior of the policies obtained from solving Stochastic Linear Quadratic Gaussian problems, thinking in particular in robot motion planning applications. To perform this analysis, we assume simplified linear systems perturbed by Gaussian noise, with state-dependend and control-dependent components, and objective functions summing up control-related and state-related costs. We provide (1) useful bounds for understanding the effect of the objective function parameters, (2) insights on what the expected paths of system should be and (3) results on the optimal choice of the planning horizon.
更多查看译文
关键词
Motion Planning under uncertainties,Linear quadratic regulator,Markov decision process
AI 理解论文
溯源树
样例
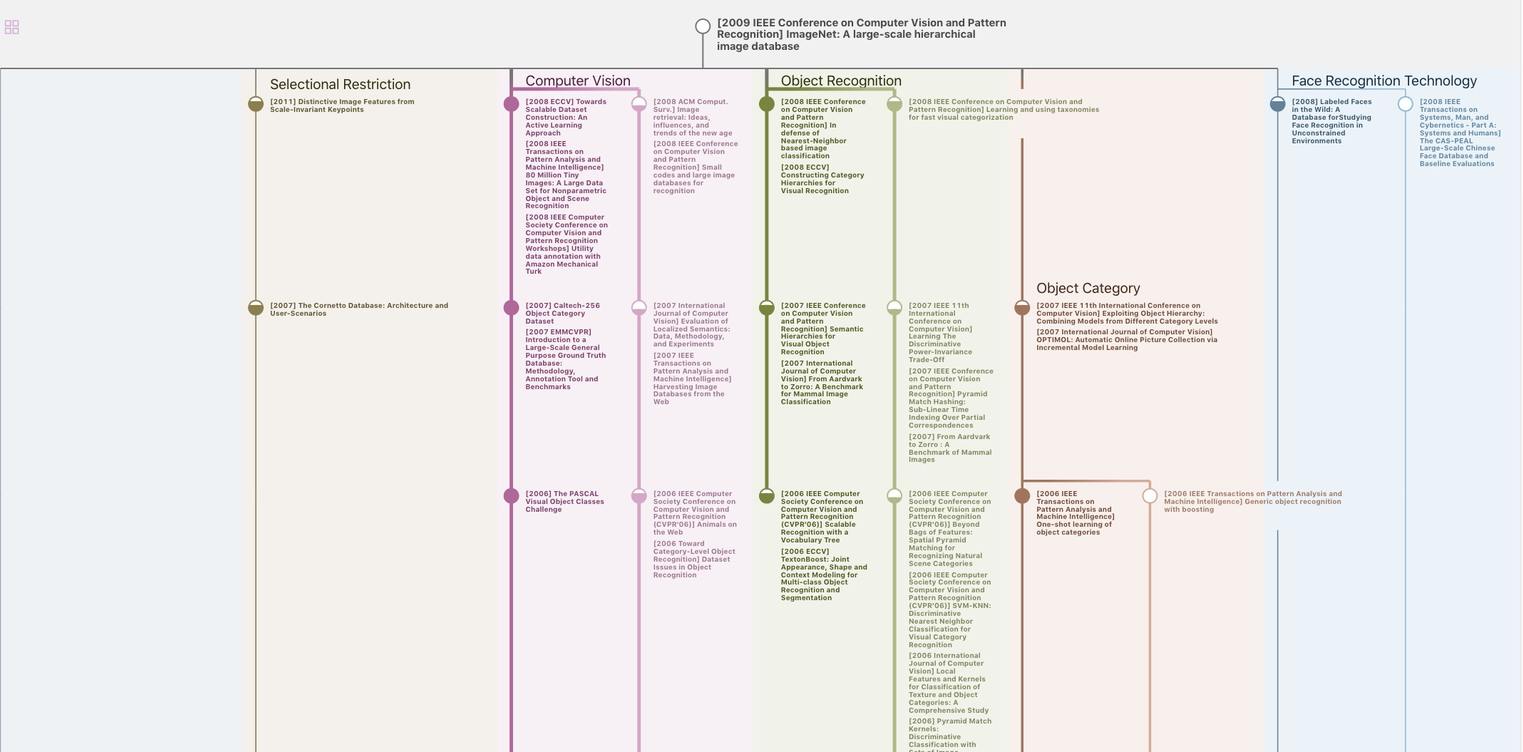
生成溯源树,研究论文发展脉络
Chat Paper
正在生成论文摘要