Learning Multiple Kernel Metrics for Iterative Person Re-Identification.
TOMCCAP(2018)
摘要
In person re-identification most metric learning methods learn from training data only once, and then they are deployed for testing. Although impressive performance has been achieved, the discriminative information from successfully identified test samples are ignored. In this work, we present a novel re-identification framework termed Iterative Multiple Kernel Metric Learning (IMKML). Specifically, there are two main modules in IMKML. In the first module, multiple metrics are learned via a new derived Kernel Marginal Nullspace Learning (KMNL) algorithm. Taking advantage of learning a discriminative nullspace from neighborhood manifold, KMNL can well tackle the Small Sample Size (SSS) problem in re-identification distance metric learning. The second module is to construct a pseudo training set by performing re-identification on the testing set. The pseudo training set, which consists of the test image pairs that are highly probable correct matches, is then inserted into the labeled training set to retrain the metrics. By iteratively alternating between the two modules, many more samples will be involved for training and significant performance gains can be achieved. Experiments on four challenging datasets, including VIPeR, PRID450S, CUHK01, and Market-1501, show that the proposed method performs favorably against the state-of-the-art approaches, especially on the lower ranks.
更多查看译文
关键词
Person re-identification, kernel method, metric learning, nullspace
AI 理解论文
溯源树
样例
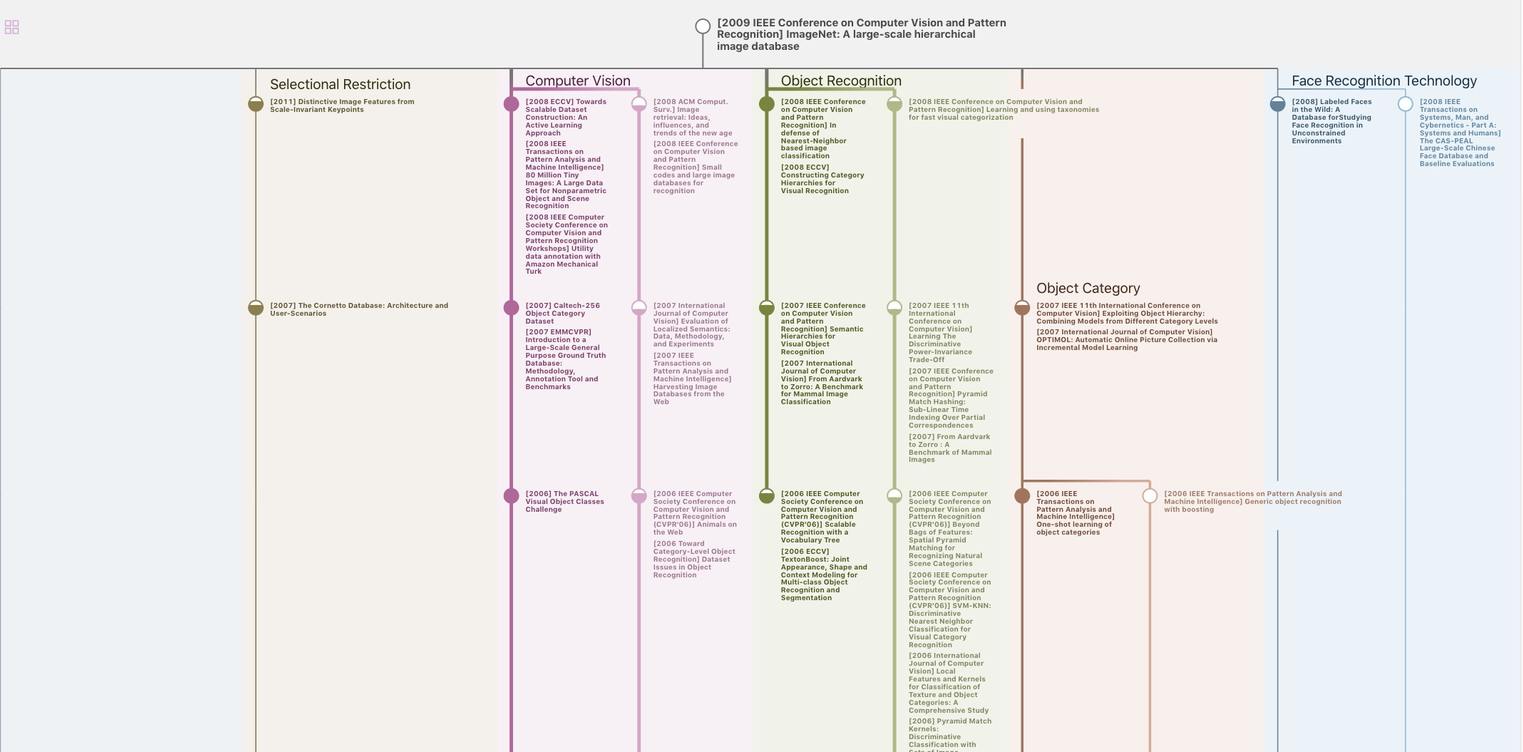
生成溯源树,研究论文发展脉络
Chat Paper
正在生成论文摘要