Aerial-Image Denoising Based On Convolutional Neural Network With Multi-Scale Residual Learning Approach
INFORMATION(2018)
摘要
Aerial images are subject to various types of noise, which restricts the recognition and analysis of images, target monitoring, and search services. At present, deep learning is successful in image recognition. However, traditional convolutional neural networks (CNNs) extract the main features of an image to predict directly and are limited by the requirements of the training sample size (i.e., a small data size is not successful enough). In this paper, using a small sample size, we propose an aerial-image denoising recognition model based on CNNs with a multi-scale residual learning approach. The proposed model has the following three advantages: (1) Instead of directly learning latent clean images, the proposed model learns the noise from noisy images and then subtracts the learned residual from the noisy images to obtain reconstructed (denoised) images; (2) The developed image denoising recognition model is beneficial to small training datasets; (3) We use multi-scale residual learning as a learning approach, and dropout is introduced into the model architecture to force the network to learn to generalize well enough. Our experimental results on aerial-image denoising recognition reveal that the proposed approach is highly superior to the other state-of-the-art methods.
更多查看译文
关键词
CNNs, multi-scale residual learning, aerial imaging, image denoising, dropout
AI 理解论文
溯源树
样例
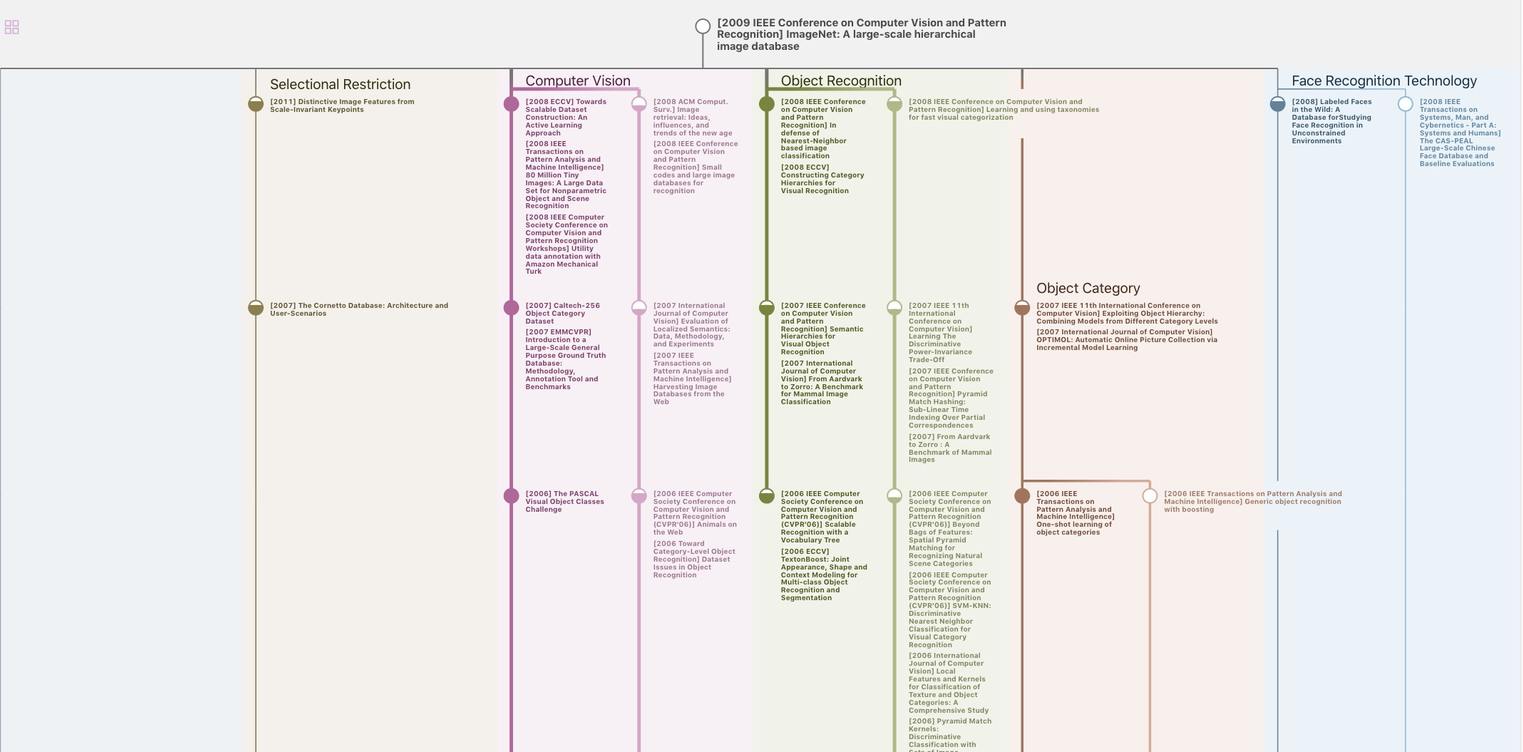
生成溯源树,研究论文发展脉络
Chat Paper
正在生成论文摘要